
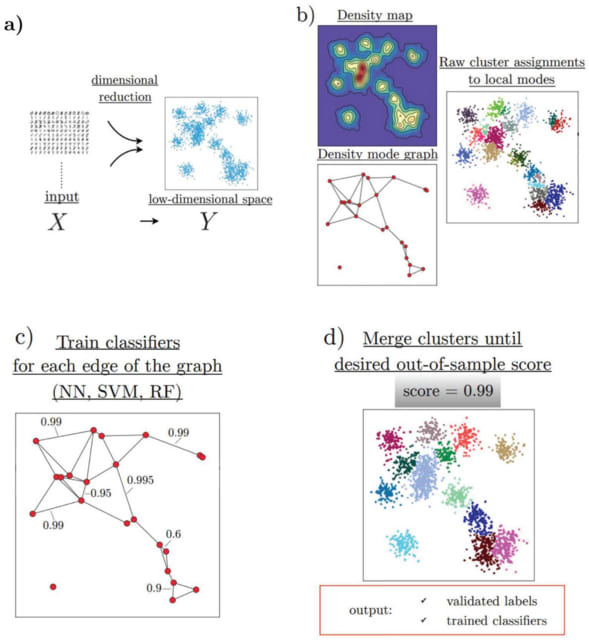
□ HAL-X: Scalable hierarchical clustering for rapid and tunable single-cell analysis
>> https://journals.plos.org/ploscompbiol/article?id=10.1371/journal.pcbi.1010349
HAL-X builds upon the idea that clustering can be viewed as a supervised learning problem where the goal is to predict the “true class labels”. HAL-X can generate multiple clusterings at varied depths to account for the specificity/sensitivity trade-off.
HAL-x is designed to cluster datasets with up to 100 million points embedded in a 50+ dimensional space. HAL-x defines an extended density neighborhood for each pure cluster, identifying spurious clusters that are representative of the same density maxima.
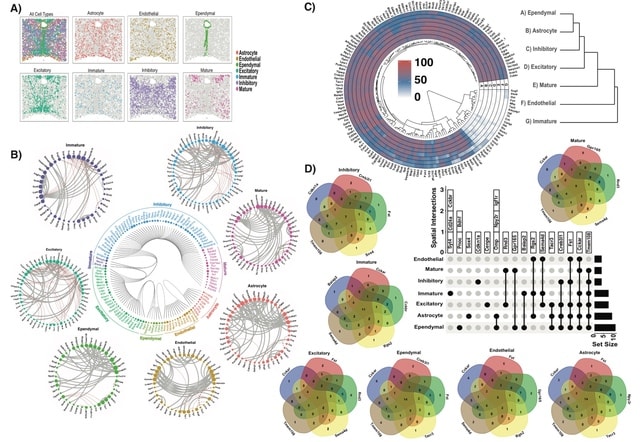
□ SpaceX: Gene Co-expression Network Estimation for Spatial Transcriptomics
>> https://academic.oup.com/bioinformatics/advance-article-abstract/doi/10.1093/bioinformatics/btac645/6731919
SpaceX employs a Bayesian model to infer spatially varying co-expression networks via incorporation of spatial information in determining network topology. The probabilistic model is able to quantify the uncertainty and based on a coherent dimension reduction.
SpaceX algorithm takes gene expression matrix, spatial locations and cluster annotations as input. The algorithm estimates the latent gene expression level using a Poisson mixed model while adjusting for covariates and spatial localization information.
SpaceX uses a tractable Bayesian estimation procedure along with a computationally efficient and scalable algorithm, as outlined below. As opposed to full-scale Markov chain Monte Carlo (MCMC) algorithm which tends to be computationally intensive.
Spatial Poisson mixed models (sPMM) is an additive structure that connects log-scaled Λ with covariate effect. The PQLseq algorithm which is a scalable penalized quasi-likelihood algorithm for sPMM with Gaussian priors using to obtain the latent gene expressions.

□ RADIAN: Language-Informed Basecalling Architecture for Nanopore Direct RNA Sequencing
>> https://www.biorxiv.org/content/10.1101/2022.10.19.512968v1
RADIAN (RNA lAnguage informeD decodIng of nAnopore sigNals), a nanopore direct RNA basecaller. RADIAN uses a probabilistic model of mRNA language, and is incorporated in a modified CTC beam search decoding algorithm.
RADIAN uses a novel way of combining chunk-level CTC matrices through averaging overlapping rows in each chunk to assemble a global matrix prior to CTC beam search decoding. Because chunk-level assembly is exact in matrix space but ambiguous in nucleotide space.

□ HALO: Towards Hierarchical Causal Representation Learning for Nonstationary Multi-Omics Data
>> https://www.biorxiv.org/content/10.1101/2022.10.17.512602v1
HALO (Hierarchical cAusal representation Learning for Omics data) adopts a causal approach to model these non- stationary causal relations using independent changing mechanisms in co-profiled single-cell ATAC- and RNA-seq data.
HALO enforces hierarchical causal relations between coupled and decoupled omics information in latent space. It allows us to identify the dynamic interplay between chromatin accessibility and transcription through temporal modulations.
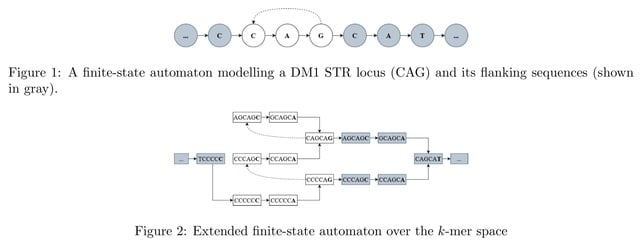
□ WarpSTR: Determining tandem repeat lengths using raw nanopore signals
>> https://www.biorxiv.org/content/10.1101/2022.11.05.515275v1
Nanopore signal is scaled and shifted differently in each sequencing read and it needs to be normalized before analysis so that the resulting values can be compared to the expected signal levels defined in the k-mer tables.
WarpSTR is an alignment-free algorithm for analysing STR alleles using nanopore sequencing raw reads. The method uses guppy basecalling annotation output for the extraction of region of interest, and dynamic time warping based finite-state automata.

□ Falign: An effective alignment tool for long noisy 3C data
>> https://www.biorxiv.org/content/10.1101/2022.10.30.514399v1
Falign, a sequence alignment method that adapts to fragmented long noisy reads, such as Pore-C reads. Falign contains four modules: 1) long fragment candidate detection; 2) monosome long fragment candidate extension; 3) monosome gap filling; and 4) polysomy gap filling.
Falign uses a local DDF chain scoring algorithm to select fragment candidates and extend the long fragment candidates. Falign selects short fragments and uses a dynamic programming-based method to generate the most plausible set of fragment alignments.

□ Seed-chain-extend alignment is accurate and runs in close to O(m log n) time for similar sequences: a rigorous average-case analysis
>> https://www.biorxiv.org/content/10.1101/2022.10.14.512303v1
The first average-case bounds on runtime and optimality for the sketched k-mer seed-chain-extend alignment heuristic under a pairwise mutation model. The alignment is mostly constrained to be near the correct diagonal of the alignment matrix and that runtime is close to linear.
Finding the smallest s-mer among the k − s + 1 s-mers in a k-mer takes k − s + 1 iterations, so finding all open syncmer seeds in S′ takes O((k − s + 1)m) = O(mk) = O(m log n) time. Subsampling Θ( 1/log n ) of k-mers asymptotically reduces the bounds on chaining time.
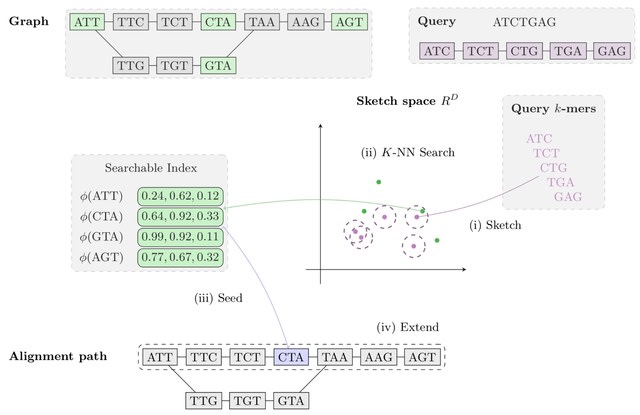
□ Aligning Distant Sequences to Graphs using Long Seed Sketches
>> https://www.biorxiv.org/content/10.1101/2022.10.26.513890v1
MetaGraph Align (MG-Align) follows a seed-and-extend approach, with a dynamic program to deter- mine which path to take in the graph, producing a semi-global alignment. A few modifications to adjust for misaligned anchors in the MG-Sketch seeder.
Using long inexact seeds based on Tensor Sketching, to be able to efficiently retrieve similar sketch vectors, the sketches of nodes are stored in a Hierarchical Navigable Small Worlds.
The method scales to graphs with 1 billion nodes, with time and memory requirements for preprocessing growing linearly with graph size and query time growing quasi-logarithmically with query length.

□ MetaGraph-MLA: Label-guided alignment to variable-order De Bruijn graphs
>> https://www.biorxiv.org/content/10.1101/2022.11.04.514718v1
Multi-label alignment (MLA) extends current sequence alignment scoring models with additional label change operations for incorporating mixtures of samples into an alignment, penalizing mixtures that are dissimilar in their sequence content.
MetaGraph-MLA, an algorithm implementing this strategy using annotated De Bruijn graphs within the MetaGraph framework. MetaGraph-MLA utilizes a variable-order De Bruijn graph and introduce node length change as an operation.
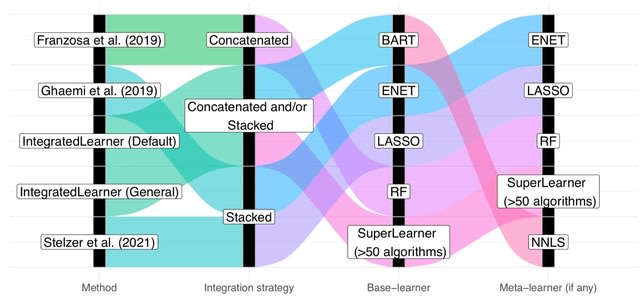
□ IntegratedLearner: An integrated Bayesian framework for multi-omics prediction and classification
>> https://www.biorxiv.org/content/10.1101/2022.11.06.514786v1
IntegratedLearner algorithm proceeds by fitting a machine learning algorithm per-layer to predict outcome (base_learner) and combining the layer-wise cross-validated predictions using a meta model (meta_learner) to generate final predictions based on all available data points.

□ RecGraph: adding recombinations to sequence-to-graph alignments
>> https://www.biorxiv.org/content/10.1101/2022.10.27.513962v1
RecGraph is a sequence-to-graph aligner written in Rust. RecGraph is an exact approach that implements a dynamic programming algorithm for computing an optimal alignment that allows recombinations with an affine penalty.
RecGraph can allow recombinations in the alignment in a controlled (i.e., non heuristic) way. RecGraph identifies a new path of the variation graph which is a mosaic of two different paths, possibly joined by a new arc.

□ Echtvar: compressed variant representation for rapid annotation and filtering of SNPs and indels
>> https://academic.oup.com/nar/advance-article/doi/10.1093/nar/gkac931/6775383
Echtvar efficiently encodes variant allele frequency and other information from huge pupulation datasets to enable rapid (1M variants/second) annotation of genetic variants. It chunks the genome into 1 - 20 (~1 million) bases, encodes each variant into a 32 bit integer.
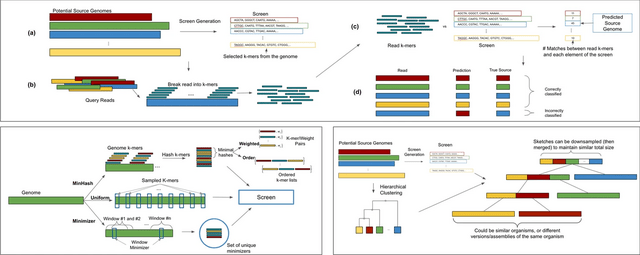
□ Sketching and sampling approaches for fast and accurate long read classification
>> https://bmcbioinformatics.biomedcentral.com/articles/10.1186/s12859-022-05014-0
Hierarchical clustering requires O(n3) time / Ω(n2) space to cluster n elements. Computation of a minimizer sketch can be done naively in O(nw) by choosing the minimum of the hashes in the O(n) windows, or in O(n) by using an integer representation of the k-mers in the sequence.
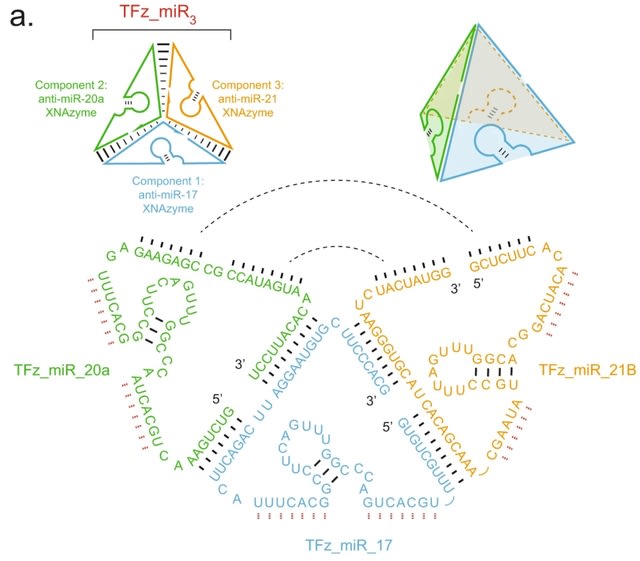
□ Targeting non-coding RNA family members with artificial endonuclease XNAzymes
>> https://www.nature.com/articles/s42003-022-03987-5
Engineering a series of artificial oligonucleotide enzymes (XNAzymes) composed of 2’-deoxy-2’-fluoro-β-D-arabino nucleic acid (FANA) that specifically or preferentially cleave individual ncRNA family members under quasi-physiological conditions.
A catalytic XNA nanostructure has improved biostability and targets multiple microRNAs. An electrophoretic mobility shift equivalent to the assembled tetrahedron (207 nts) was observed when all three components were annealed.

□ SPACE: Exploiting spatial dimensions to enable parallelized continuous directed evolution
>> https://www.embopress.org/doi/full/10.15252/msb.202210934
SPACE, a system for rapid / parallelizable evolution of biomolecules, which introduces spatial dimensions into the continuous evolution system. The system leverages competition over space, wherein evolutionary progress is closely associated w/ the production of spatial patterns.
SPACE uses a mathematical model, RESIR - Range Expansion with Susceptible Infected Recovered kinetics. SPACE is applied to evolve the promoter recognition of T7 RNA polymerase to a library of 96 random sequences in parallel.

□ Holographic-(V)AE: an end-to-end SO(3)-Equivariant (Variational) Autoencoder in Fourier Space
>> https://www.biorxiv.org/content/10.1101/2022.09.30.510350v1
As spherical harmonics form a basis for the irreps of SO(3), the SO(3) group acts on spherical Fourier space via a direct sum of irreps. The ZFT encodes a data point into a tensor composed of a direct sum of features, each associated with a degree l indicating the irrep.
Refer to these tensors as SO(3)-steerable tensors and to the vector spaces they occupy as SO(3)-steerable vector spaces, or simply steerable for short since they only deal with the SO(3) group in this work.
H-(V)AE reconstructs the spherical Fourier space encoding of data, learning in the process a latent space with a maximally informative invariant embedding alongside an equivariant frame describing the orientation of the data.

□ Entropy predicts fuzzy-seed sensitivity
>> https://www.biorxiv.org/content/10.1101/2022.10.13.512198v1
The entropy of a seed cover (a stretch of neighboring seeds) is a good predictor for seed sensitivity. Proposing a model to estimate the entropy of a seed cover, and find that seed covers with high entropy typically have high match sensitivity.
Altstrobes are modified randstrobes where the strobe length alternates between shorter and longer strobes. Mixedstrobes samples either a k-mer or a strobemer at a specified fraction. Using subsampled randstrobes and mixedstrobes within minimap2 for the most divergent sequence.
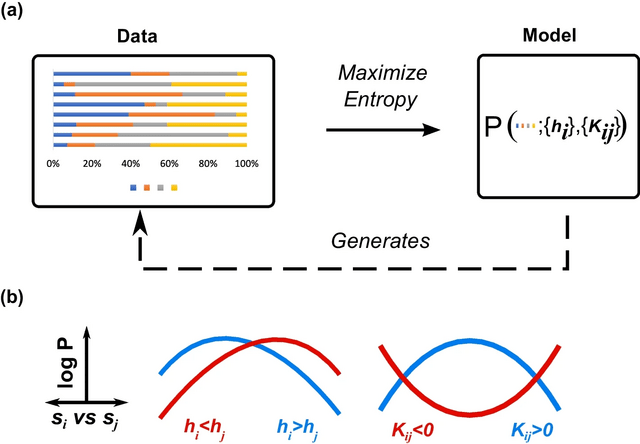
□ The maximum entropy principle for compositional data
>> https://bmcbioinformatics.biomedcentral.com/articles/10.1186/s12859-022-05007-z
Compositional Maximum Entropy (CME), a probabilistic framework for inferring the behaviors of compositional systems. By integrating the prior geometric structure of compositions, CME infers the underlying multivariate relationships b/n the constituent components.
The principle of maximum entropy deduces the simplex-truncated normal distribution from the given moment constraints. The simplex pseudolikelihood method provides consistent and asymptotically parameter estimates and is asymptotically equivalent to maximum likelihood estimation.

□ SDRAP for annotating scrambled or rearranged genomes
>> https://www.biorxiv.org/content/10.1101/2022.10.24.513505v1
SDRAP, Scrambled DNA Rearrangement Annotation Protocol, annotates DNA segments in DNA rearrangement precursor and product genomes which describe the rearrangement, and computes properties of the rearrangements reflecting their complexity.
SDRAP implements a heuristic adaptation of the Smith-Waterman gapped local sequence alignment algorithm. The regions on the precursor sequence in between precursor intervals of the union of all arrangements are annotated as eliminated sequences.

□ Free decomposition spaces
>> https://arxiv.org/pdf/2210.11192v1.pdf
Constructing an equivalence of ∞-categories. Left Kan extension along the inclusion j : ∆inert → ∆ takes general objects to Mobius decomposition spaces and general maps to CULF maps.
The Aguiar–Bergeron– Sottile map to the decomposition space of quasi-symmetric functions, from any Mobius decomposition space, factors through the free decomposition space of nondegenerate simplices, and offer an explanation of the zeta function in the universal property of QSym.

□ The central sheaf of a Grothendieck category
>> https://arxiv.org/pdf/2210.12419v1.pdf
The center Z(A) of an abelian category A is the endomorphism ring of the identity functor on that category. A localizing subcategory of a Grothendieck category C is said to be stable if it is stable under essential extensions.
The Grothendieck category C is locally noetherian. And constructing an alternative version of the central sheaf ZC which will be a sheaf on the topological space Sp(C) equipped with the so-called stable topology.
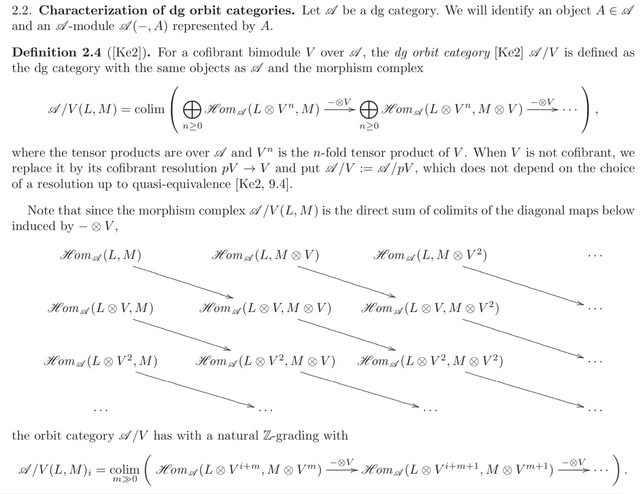
□ Enhanced Auslander-Reiten duality and tilting theory for singularity categories
>> https://arxiv.org/abs/2209.14090v1
Proving an equivalence exists as soon as there is a triangle equivalence between the graded singularity category of a Gorenstein ring and the derived category of a finite dimensional algebra.
Gorenstein rings of dimension at most 1, quotient singularities, and Geigle-Lenzing complete intersections, including finite or infinite Grassmannian cluster categories, to realize their singularity categories as cluster categories of finite dimensional algebras.

□ MD-Cat: Expectation-Maximization enables Phylogenetic Dating under a Categorical Rate Model
>> https://www.biorxiv.org/content/10.1101/2022.10.06.511147v1
MD-Cat (Molecular Dating using Categorical-models) uses a categorical model to approximate the unknown continuous clock model. It is inspired by non-parametric statistics and can approximate a large family of models by discretizing the rate distribution into k categories.
Although the rate categories are discrete, the model has the power to approximate a continuous clock model if k is large and there are enough data. MD-Cat has fewer assumptions about the true clock model than parametric models such as Gamma or LogNormal distribution.
EM algorithm maximizes the likelihood function associated w/ this model, where the k rate categories and branch lengths in time units are modeled as unknown parameters and co-estimated. The E-step / M-step can be computed efficiently, and the algorithm is guaranteed to converge.
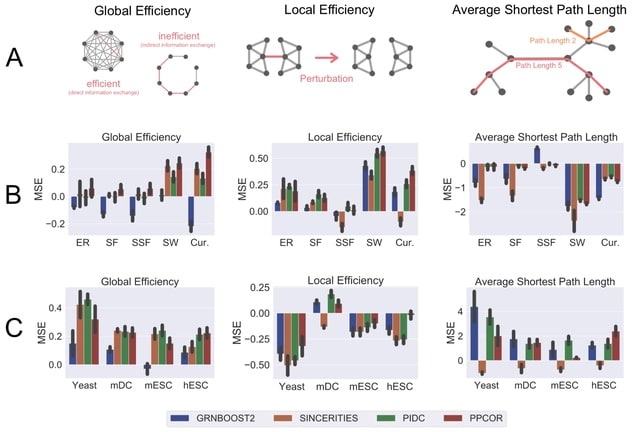
□ STREAMLINE: Structural and Topological Performance Analysis of Algorithms for the Inference of Gene Regulatory Networks from Single-Cell Transcriptomic Data
>> https://www.biorxiv.org/content/10.1101/2022.10.31.514493v1
STREAMLINE quantifies the ability of algorithms to capture topological properties of networks and identify hubs. This repository contains all the necessary files that are necessary to perform the analysis. The implementation is compatible with BEELINE.
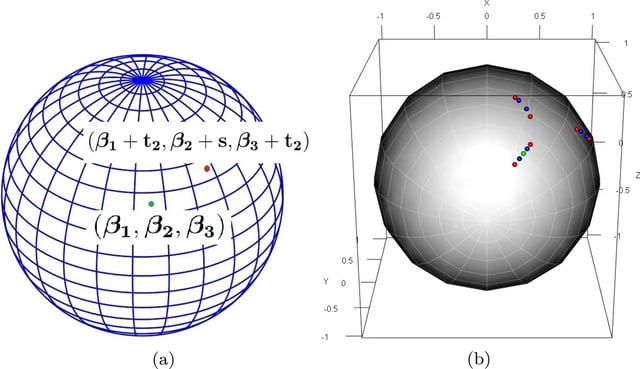
□ SCOR: Estimating the optimal linear combination of predictors using spherically constrained optimization
>> https://bmcbioinformatics.biomedcentral.com/articles/10.1186/s12859-022-04953-y
Spherically Constrained Optimization Routine (SCOR) can be used in various other statistical problems such as directional statistics or single-index models where fixing the norm of the coefficient vector is needed to avoid the issue of non-identifiability.
SCOR obtains better estimates of the empirical hypervolume under the manifold (EHUM). In the future, the SCOR algorithms can be extended to the variable selection problem over the coefficients belonging to the surface of a unit sphere.

□ BRANEnet: embedding multilayer networks for omics data integration
>> https://bmcbioinformatics.biomedcentral.com/articles/10.1186/s12859-022-04955-w
BRANEnet, a novel multi-omics integration framework for multilayer heterogeneous networks. BRANENET is an expressive, scalable, and versatile method to learn node embeddings, leveraging random walk information within a matrix factorization framework.
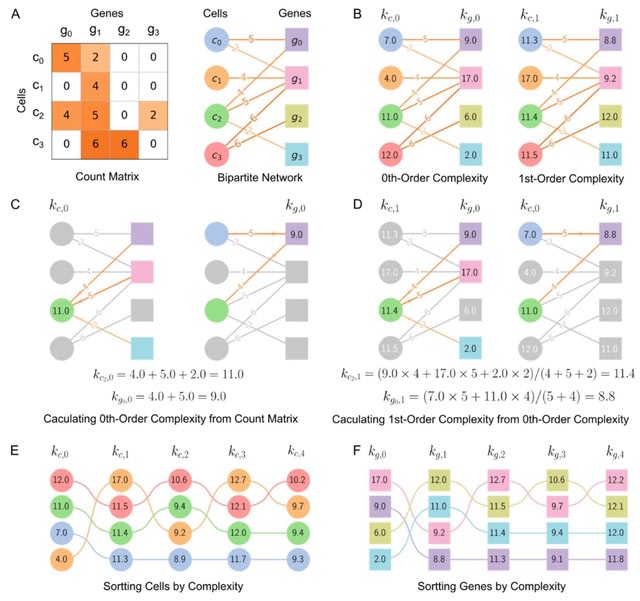
□ SCTC: inference of developmental potential from single-cell transcriptional complexity
>> https://www.biorxiv.org/content/10.1101/2022.10.14.512265v1
Calculating 0th-order complexities of cell and gene by summing over the weights of edges connected to them. 1st-order complexities of cell and gene can be obtained by averaging the 0th-order complexities. It calculate each order complexity and to reconstruct pseudo-temporal path.
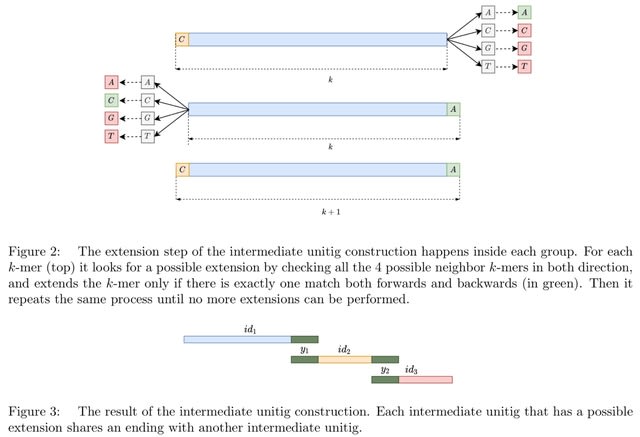
□ DeepSelectNet: Deep Neural Network Based Selective Sequencing for Oxford Nanopore Sequencing
>> https://www.biorxiv.org/content/10.1101/2022.10.24.513498v1
DeepSelecNet is an improved 1D ResNet based model to classify Oxford Nanopore raw electrical signals as target or non-target for Read-Until sequence enrichment or depletion. DeepSelecNet provides enhanced model performances.
DeepSelectNet relies on neural net regularization to minimise model complexity thereby reducing the overfitting of data. A longer signal segment means having a larger k-mer size that allows distinguishing species better, thereby the model may classify better with longer segments.

□ INSERT-seq enables high-resolution mapping of genomically integrated DNA using Nanopore sequencing
>> https://genomebiology.biomedcentral.com/articles/10.1186/s13059-022-02778-9
INSERT-seq incorporates amplification based enrichment and UMI amplification with a computational pipeline to process integration sites. INSERT-seq can sensitively detect insertion sites with frequencies as low as 1%. Such sensitivity could be improved with more sequencing depth.
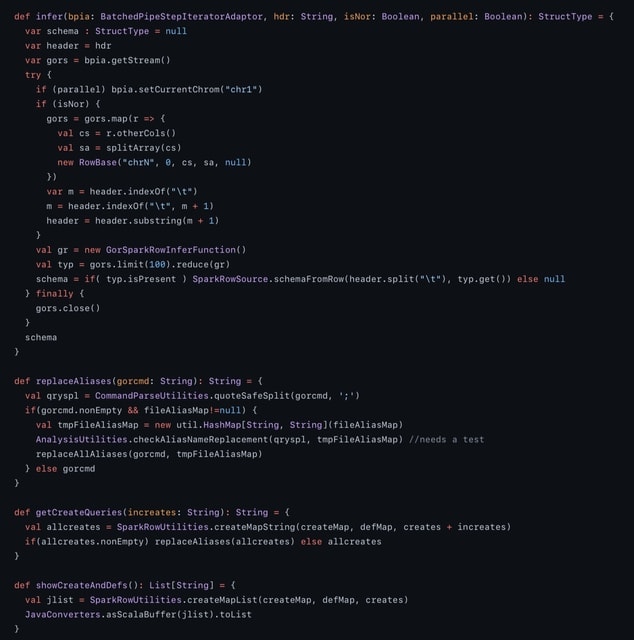
□ Ultra-fast joint-genotyping with SparkGOR
>> https://www.biorxiv.org/content/10.1101/2022.10.25.513331v1
The pipeline accepts single sample gVCF-like input and generates pVCF-like output. By converting multi-allelic locus based variant calls to bi-allelic variants, It simplify the joint-genotyping computation dramatically while maintaining quality and concordance with GIAB samples.
Using a Spark implementation of XGBoost to train and predict variant classification. And they used the Sentieon release of the GATK VQSR Gaussian-mixture algorithm using the features MQ, QD, DP, MQRankSum, ReadPosRankSum, FS, SOR, InbreedingCoeff.

□ Deep mendelian randomization: Investigating the causal knowledge of genomic deep learning models
>> https://journals.plos.org/ploscompbiol/article?id=10.1371/journal.pcbi.1009880
Deep Mendelian Randomization (DeepMR), obtains local and global estimates of linear causal relationship between marks. DeepMR gives accurate and unbiased estimates of the ‘true’ global causal effect, but its coverage decays in the presence of sequence-dependent confounding.
DeepMR can estimate overall per-exposure causal effects using a random effects meta-analysis across sequence regions (loci) and provide further evidence for previously hypothesized relationships between TFs identified by BPNet.

□ NanoBlot: A Simple Tool for Visualization of RNA Isoform Usage From Third Generation RNA-sequencing Data
>> https://www.biorxiv.org/content/10.1101/2022.10.26.513894v1
NanoBlot takes aligned, positionally-sorted, and indexed BAM files as input. NanoBlot requires a series of target genomic regions referred to as “probes”. NanoBlot removes any reads which map to the antiprobe(s) region.

□ MetaLP: An integrative linear programming method for protein inference in metaproteomics
>> https://journals.plos.org/ploscompbiol/article?id=10.1371/journal.pcbi.1010603
MetaLP, a protein inference algorithm in metaproteomics using an integrative linear programming method. Taxonomic abundance information extracted from metagenomics shotgun sequencing or 16s rRNA gene amplicon sequencing, was incorporated as prior information in MetaLP.
MetaLP expresses the joint probability with a chain rule to transform it into a chain of conditional probabilities, which could be easily added as logical constraints. The LP model can be solved quickly by existing LP solvers.
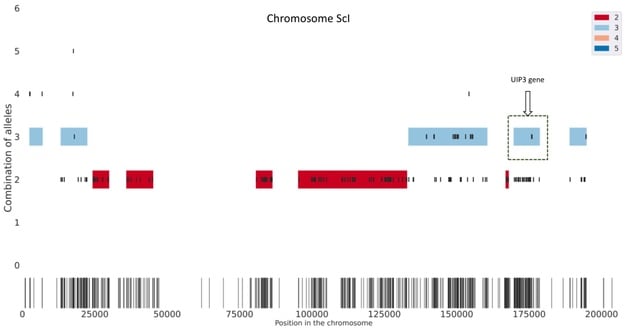
□ HAT: Haplotype Assembly Tool using short and error-prone long reads
>> https://academic.oup.com/bioinformatics/advance-article/doi/10.1093/bioinformatics/btac702/6779972
HAT creates seeds based on short read alignments and the location of SNPs. Then, it removes the combinations of alleles with low support as well as overlapping seeds. Next, HAT finds multiplicity blocks and creates the first phased blocks within them.
HAT assigns reads to the blocks and haplotypes; based on these read assignments it fills the unphased SNPs within blocks. (C.) Finally, HAT can also use miniasm to assemble haplotype sequences for each block and polishes the assemblies using Pilon.
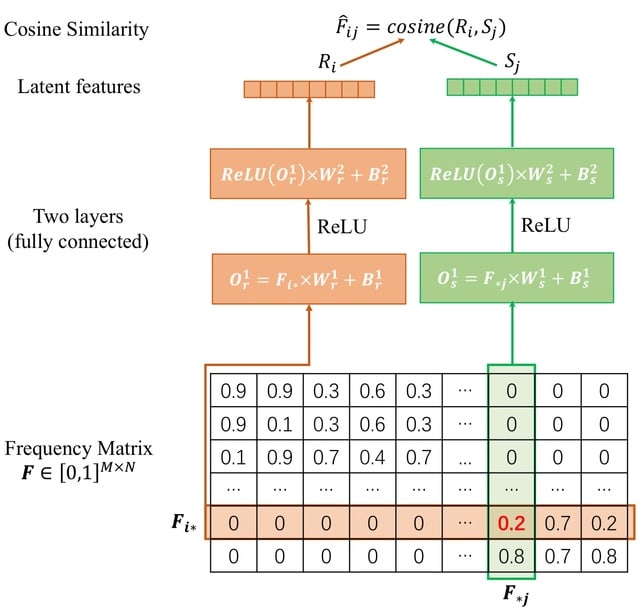
□ HaploDMF: viral Haplotype reconstruction from long reads via Deep Matrix Factorization
>> https://academic.oup.com/bioinformatics/advance-article/doi/10.1093/bioinformatics/btac708/6780015
HaploDMF utilizes a deep matrix factorization model with an adapted loss function to learn latent features from aligned reads automatically. The latent features are then used to cluster reads of the same haplotype.

□ kmdiff, large-scale and user-friendly differential k-mer analyses
>> https://academic.oup.com/bioinformatics/advance-article/doi/10.1093/bioinformatics/btac689/6782954
kmdiff provides differential k-mers analysis between two populations (control and case). Each population is represented by a set of short-read sequencing. Outputs are differentially represented k-mers between controls and cases.
kmdiff deviates from HAWK in the k-mer counting part. HAWK counts k-mers of each sample before loading and testing batches of them using a hash table.
kmdiff constructs a k-mer matrix, i.e. an abundance matrix with k-mers in rows and samples in columns. this matrix is not represented as a whole but sub-matrices are streamed in parallel using kmtricks.