
□ pythrahyper_net: Biological network growth in complex environments: A computational framework
>> https://journals.plos.org/ploscompbiol/article?id=10.1371/journal.pcbi.1008003
the properties of complex networks are often calculated with respect to canonical random graphs such as the Erdös-Renyi or Watts-Strogatz model. These models are defined by the topological structure in an adjacency matrix, but usually neglect spatial constraints.
pythrahyper_net is a probabilistic agent-based model to describe individual growth processes as biased, correlated random motion. pythrahyper_net is a computational framework based on directional statistics to model network formation in space and time under arbitrary spatial constraints.
Probability distributions are modeled as multivariate Gaussian distributions (MGD), with mean and covariance determined from the discrete simulation grid. Individual MGDs are combined by convolution, transformed to spherical coordinates, and projected onto the unit sphere.

□ NanosigSim: Simulation of Nanopore Sequencing Signals Based on BiGRU
>> https://www.mdpi.com/1424-8220/20/24/7244
NanosigSim, a signal simulation method based on Bi-directional Gated Recurrent Units (BiGRU). NanosigSim signal processing model has a novel architecture that couples a three-layer BiGRU and a fully connected layer.
NanosigSim can model the relation between ground-truth signal and real-world sequencing signal through experimental data to accurately filter out the useless high-frequency components. This process can be achieved by using Continuous Wavelet Dynamic Time Warping.
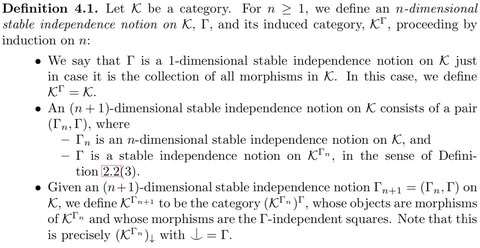
□ Induced and higher-dimensional stable independence
>> https://arxiv.org/pdf/2011.13962v1.pdf
Stable independence in the context of accessible categories. This notion has its origins in the model-theoretic concept of stable nonforking, which can be thought of on one hand as a freeness property of type extensions. As a notion of freeness or independence for amalgams of models.
Given an (n+1)-dimensional stable independence notion Γn+1 = (Γn, Γ), KΓn+1 to be the category(KΓn)Γ, whose objects are morphisms of KΓn and whose morphisms are the Γ-independent squares. ⌣ is λ-accessible, λ an infinite regular cardinal, if the category K↓ is λ-accessible.
a stable independence notion immediately yields higher-dimensional independence, taken to its logical conclusion, leads to a formulation of stable independence as a property of commutative squares in a general category, described by a family of purely category-theoretic axioms.

□ Characterisations of Variant Transfinite Computational Models: Infinite Time Turing, Ordinal Time Turing, and Blum-Shub-Smale machines
>> https://arxiv.org/pdf/2012.08001.pdf
Using admissibility theory, Σ2-codes and Π3-reflection properties in the constructible hierarchy to classify the halting times of ITTMs with multiple independent heads; the same for Ordinal Turing Machines which have On length tapes.
Infinite Time Blum-Shub-Smale machines (IBSSM’s) have a universality property - this is because ITTMs do and the two classes of machine are ‘bi-simulable’. This is in contradistinction to the machine using a ‘continuity’ rule which fails to be universal.
□ Linear space string correction algorithm using the Damerau-Levenshtein distance
>> https://bmcbioinformatics.biomedcentral.com/articles/10.1186/s12859-019-3184-8
Linear space algorithms to compute the Damerau-Levenshtein (DL) distance between two strings and determine the optimal trace. Lowrance and Wagner have developed an O(mn) time O(mn) space algorithm to find the minimum cost edit sequence between strings of length m and n.
The linear space algorithms uses a refined dynamic programming recurrence. the more general algorithm in string correction using the Damerau-Levenshtein distance that runs in O(mn) time and uses O(s∗min{m,n}+m+n) space.
□ The Diophantine problem in finitely generated commutative rings
>> https://arxiv.org/pdf/2012.09787.pdf
the Diophantine problem, denoted D(R), in infinite finitely generated commutative associative unitary rings R.
a polynomial time algorithm that for a given finite system S of polynomial equations with coefficients in O constructs a finite system S ̊ of polynomial equations with coefficients in R such that S has a solution in O if and only if S ̊ has a solution in R.
□ MathFeature: Feature Extraction Package for Biological Sequences Based on Mathematical Descriptors
>> https://www.biorxiv.org/content/10.1101/2020.12.19.423610v1.full.pdf
MathFeature provides 20 approaches based on several studies found in the literature, e.g., multiple numeric mappings, genomic signal processing, chaos game theory, entropy, and complex networks.
Various studies have applied concepts from information theory for sequence feature extraction, mainly Shannon’s entropy. Another entropy-based measure has been successfully explored, e.g., Tsallis entropy, proposed to generalize the Boltzmann/Gibbs’s traditional entropy.
□ Megadepth: efficient coverage quantification for BigWigs and BAMs
>> https://www.biorxiv.org/content/10.1101/2020.12.17.423317v1.full.pdf
Megadepth is a fast tool for quantifying alignments and coverage for BigWig and BAM/CRAM input files, using substantially less memory than the next-fastest competitor.
Megadepth can summarize coverage within all disjoint intervals of the Gencode V35 gene annotation for more than 19,000 GTExV8 BigWig files in approximately one hour using 32 threads.
Megadepth can be configured to use multiple HTSlib threads for reading BAMs, speeding up block-gzip decompression.
megadepth allocates a per-base counts array across the entirety of the current chromosome before processing the alignments from that chromosome.

□ VEGA: Biological network-inspired interpretable variational autoencoder
>> https://www.biorxiv.org/content/10.1101/2020.12.17.423310v1.full.pdf
VEGA (Vae Enhanced by Gene Annotations), a novel sparse Variational Autoencoder architecture, whose decoder wiring is inspired by a priori characterized biological abstractions, providing direct interpretability to the latent variables.
Composed of a deep non-linear encoder and a masked linear decoder, VEGA encodes single-cell transcriptomics data in an interpretable latent space specified a priori.
□ Sfaira accelerates data and model reuse in single cell genomics
>> https://www.biorxiv.org/content/10.1101/2020.12.16.419036v1.full.pdf
a size factor-normalised, but otherwise non-processed feature space, for models so that all genes can contribute to embeddings and classification and the contribution of all genes can be dissected without the issue of removing low variance features.
Sfaira automatizes exploratory analysis of single-cell data. Sfaira allows fitting of cell type classifiers for data sets with different levels of annotation granularity by using cell type ontologies. And allows streamlined embedding models training across whole atlases.
□ Methrix: Systematic aggregation and efficient summarization of generic bedGraph files from Bisufite sequencing
>> https://academic.oup.com/bioinformatics/advance-article-abstract/doi/10.1093/bioinformatics/btaa1048/6042753
Core functionality of Methrix includes a comprehensive bedGraph - which summarizes methylation calls based on annotated reference indices, infers and collapses strands, and handles uncovered reference CpG sites while facilitating a flexible input file format specification.
Methrix enriches established WGBS workflows by bringing together computational efficiency and versatile functionality.

□ Parallel String Graph Construction and Transitive Reduction for De Novo Genome Assembly
>> https://arxiv.org/pdf/2010.10055.pdf
a sparse linear algebra centric approach for distributed memory parallelization of overlap and layout phases. Formulating the overlap detection as a distributed Sparse General Matrix Multiply.
Sparse matrix-matrix multiplication allows diBELLA to efficiently parallelize the computation without losing expressiveness, thanks to the semiring abstraction. a novel distributed memory algorithm for the transitive reduction of the overlap graph.

□ SOMDE: A scalable method for identifying spatially variable genes with self-organizing map
>> https://www.biorxiv.org/content/10.1101/2020.12.10.419549v1.full.pdf
SOMDE, an efficient method for identifying SVgenes in large-scale spatial expression data. SOMDE uses self-organizing map (SOM) to cluster neighboring cells into nodes, and then uses a Gaussian Process to fit the node-level spatial gene expression to identify SVgenes.
SOMDE converts the original spatial gene expression to node-level gene meta-expression profiles. SOMDE models the condensed representation of the original spatial transcriptome data with a modified Gaussian process to quantify the relative spatial variability.
□ LISA: Learned Indexes for DNA Sequence Analysis
>> https://www.biorxiv.org/content/10.1101/2020.12.22.423964v1.full.pdf
LISA (Learned Indexes for Sequence Analysis) accelerates two of the most essential flavors of DNA sequence search—exact search and super-maximal exact match (SMEM) search.
LISA achieves 13.3× higher throughput than Trans-Omics Acceleration Library (TAL). Super-Maximal Exact Match for every position in the read, search of exact matches of longest substring of the read that passes through that position and still has a match in the reference sequence.

□ EVE: Large-scale clinical interpretation of genetic variants using evolutionary data and deep learning
>> https://www.biorxiv.org/content/10.1101/2020.12.21.423785v1.full.pdf
EVE (Evolutionary model of Variant Effect) learns a distribution over amino acid sequences from evolutionary data. It enables the computation of the evolutionary index. A global-local mixture of Gaussian Mixture Models separates variants into benign and pathogenic clusters based on that index.
EVE reflects the probabilistic assignment to either pathogenic or benign clusters. The probabilistic nature of the model enables us to quantify the uncertainty on this cluster assignment, which can bin variants into Benign / Pathogenic by assigning some variants as Uncertain.
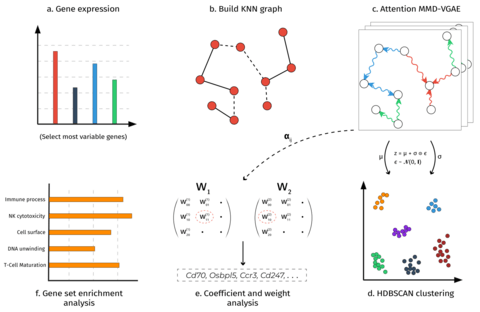
□ CellVGAE: An unsupervised scRNA-seq analysis workflow with graph attention networks
>> https://www.biorxiv.org/content/10.1101/2020.12.20.423645v1.full.pdf
CellVGAE uses the connectivity between cells (e.g. k-nearest neighbour graphs or KNN) with gene expression values as node features to learn high-quality cell representations in a lower-dimensional space, with applications in downstream analyses like (density-based) clustering.
CellVGAE leverages the connectivity between cells, represented as a graph, to perform convolutions on a non-Euclidean structure, thus subscribing to the geometric deep learning paradigm.

□ Cytopath: Simulation based inference of differentiation trajectories from RNA velocity fields
>> https://www.biorxiv.org/content/10.1101/2020.12.21.423801v1.full.pdf
Cytopath is based upon transitions that use the full expression and velocity profiles of cells, it is less prone to projection artifacts distorting expression profile similarity.
The objective of trajectory inference is to estimate trajectories from root to terminal state. a common terminal state are aligned using Dynamic Time Warping. Root / terminal states can either be derived from a Markov random-walk model utilizing the transition probability matrix.

□ GCNG: graph convolutional networks for inferring gene interaction from spatial transcriptomics data
>> https://genomebiology.biomedcentral.com/articles/10.1186/s13059-020-02214-w
GCNG model using spatial single cell expression data. A binary cell adjacent matrix and an expression matrix are extracted from spatial data. After normalization, both matrices are fed into the graph convolutional network.
GCNG consists of two graph convolutional layers, one flatten layer, one 512-dimension dense layer, and one sigmoid function output layer for classification.

□ GraphBLAST: A High-Performance Linear Algebra-based Graph Framework on the GPU
>> https://arxiv.org/pdf/1908.01407.pdf
GraphBLAST has on average at least an order of magnitude speedup over previous GraphBLAS implementations SuiteSparse and GBTL, comparable performance to the fastest GPU hardwired primitives and shared-memory graph frameworks Ligra and Gunrock.
Currently, direction-optimization is only active for matrix-vector multiplication. However, in the future, the optimization can be extended to matrix-matrix multiplication.

□ DipAsm: Chromosome-scale, haplotype-resolved assembly of human genomes
>> https://www.nature.com/articles/s41587-020-0711-0
DipAsm uses long, accurate reads and long-range conformation data for single individuals to generate a chromosome-scale phased assembly within 1 day.
A potential solution is to retain heterozygous events in the initial assembly graph and to scaffold and dissect these events later to generate a phased assembly.
DipAsm accurately reconstructs the two haplotypes in a diploid individual using only PacBio’s long high-fidelity (HiFi) reads and Hi-C data, both at ~30-fold coverage, without any pedigree information.

□ Fully phased human genome assembly without parental data using single-cell strand sequencing and long reads
>> https://www.nature.com/articles/s41587-020-0719-5
A comparison of Oxford Nanopore Technologies and Pacific Biosciences phased assemblies identified 154 regions that are preferential sites of contig breaks, irrespective of sequencing technology or phasing algorithms.
examining the whole major histocompatibility complex (MHC) region and found that it was traversed by a single contig in both haplotype assemblies.

□ Characterizing finitely generated fields by a single field axiom
>> https://arxiv.org/pdf/2012.01307v1.pdf
The Elementary Equivalence versus Isomorphism Problem, for short EEIP, asks whether the elementary theory Th(K) of a finitely generated field K (always in the language of rings) encodes the isomorphism type of K in the class of all finitely generated fields.
every field K is elementary equivalent to its “constant field” κ – the relative algebraic closure of the prime field in K –, and its first-order theory is decidable.
Concerning with fields which are at the centre of (birational) arithmetic geometry, namely the finitely generated fields K, which are the function fields of integral Z-schemes of finite type.
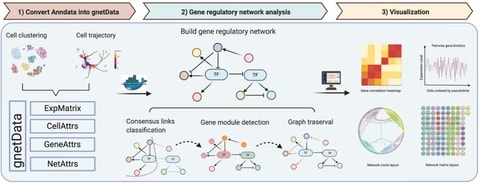
□ PySCNet: A tool for reconstructing and analyzing gene regulatory network from single-cell RNA-Seq data
>> https://www.biorxiv.org/content/10.1101/2020.12.18.423482v1.full.pdf
PySCNet integrates competitive gene regulatory construction methodologies for cell specific or trajectory specific gene regulatory networks (GRNs) and allows for gene co-expression module detection and gene importance evaluation.
PySCNet uses Louvain clustering to detect gene co-expression modules. Node centrality is applied to estimate the importance of gene / TF in the network. To discover hidden regulating links of a target gene node, graph traversal are utilized to predict indirect regulations.

□ SCMER: Single-Cell Manifold Preserving Feature Selection
>> https://www.biorxiv.org/content/10.1101/2020.12.01.407262v1.full.pdf
SCMER, a novel unsupervised approach which performs UMAP style dimensionality reduction via selecting a compact set of molecular features with definitive meanings.
a manifold defined by pairwise cell similarity scores sufficiently represents the complexity of the data, encoding both global relationship between cell groups and local relationship within cell groups.
While clusters usually reflect distinct cell types, continuums reflect similar cell types and trajectory of transitioning/differentiating cell states. SCMER selects optimal features that preserve the manifold and retain inter- and intra-cluster diversity.
SCMER does not require clusters or trajectories, and thereby circumvents the associated biases. It is sensitive to detect diverse features that delineate common and rare cell types, continuously changing cell states, and multicellular programs shared by multiple cell types.
If a dataset with n cells is separate into b batches, the space complexity will reduce from O(n^2) to O(b * (n/b)^2) = O(n^2 / b).
Orthant-Wise Limited memory quasi-Newton (OWL-QN) algorithm solves the l2-regularized regression problem by introducing pseudo-gradients and restrict the optimization to an orthant without discontinuities in the gradient.

□ A Scalable Optimization Mechanism for Pairwise based Discrete Hashing
>> https://ieeexplore.ieee.org/document/9280410
a novel alternative optimization mechanism to reformulate one typical quartic problem, in term of hash functions in the original objective of Kernel- based Supervised Hashing, into a linear problem by introducing a linear regression model.
a scalable symmetric discrete hashing algorithm that gradually and smoothly updates each batch of binary codes. And a greedy symmetric discrete hashing algorithm to update each bit of batch binary codes.

□ SpaGCN: Integrating gene expression, spatial location and histology to identify spatial domains and spatially variable genes by graph convolutional network
>> https://www.biorxiv.org/content/10.1101/2020.11.30.405118v1.full.pdf
SpaGCN draws a circle around each spot with a pre-specified radius, and all spots that reside in the circle are considered as neighbors of this spot. SpaGCN allows to combine multiple domains as one target domain or specify which neighboring domains to be included in DE analysis.
SpaGCN can identify spatial domains with coherent gene expression and histology and detect SVGs and meta genes that have much clearer spatial expression patterns and biological interpretations than genes detected by SPARK and SpatialDE.

□ GRGNN: Inductive inference of gene regulatory network using supervised and semi-supervised graph neural networks
>> https://www.sciencedirect.com/science/article/pii/S200103702030444X
GRGNN - an end-to-end gene regulatory graph neural network approach to reconstruct GRNs from scratch utilizing the gene expression data, in both a supervised and a semi-supervised framework.
One of the time-consuming parts of GRGNN practice is extracting the enclosed subgraphs. The time complexity is O(n|V|h) and the memory complexity is O(n|E|) for extracting n subgraphs in h-hop, where |V| and |E| are numbers of nodes and edges in the whole graph.
□ spVCF: Sparse project VCF: efficient encoding of population genotype matrices
>> https://academic.oup.com/bioinformatics/advance-article/doi/10.1093/bioinformatics/btaa1004/6029516
Sparse Project VCF (spVCF), an evolution of VCF with judicious entropy reduction and run-length encoding, delivering >10X size reduction for modern studies with practically minimal information loss.
spVCF interoperates with VCF efficiently, including tabix-based random access. spVCF provides the genotype matrix sparsely, by selectively reducing QC measure entropy and run-length encoding repetitive information about reference coverage.
□ SDPR: A fast and robust Bayesian nonparametric method for prediction of complex traits using summary statistics
>> https://www.biorxiv.org/content/10.1101/2020.11.30.405241v1.full.pdf
SDPR (Summary statistics based Dirichelt Process Regression) is a method to compute polygenic risk score (PRS) from summary statistics. It is the extension of Dirichlet Process Regression (DPR) to the use of summary statistics.
SDPR connects the marginal coefficients in summary statistics with true effect sizes through Bayesian multiple DPR. And utilize the concept of approximately independent LD blocks and reparameterization to develop a parallel and fast-mixing Markov Chain Monte Carlo algorithm.

□ Maximum Caliber: Inferring a network from dynamical signals at its nodes
>> https://journals.plos.org/ploscompbiol/article?id=10.1371/journal.pcbi.1008435
an approximate solution to the difficult inverse problem of inferring the topology of an unknown network from given time-dependent signals at the nodes.
The method of choice for inferring dynamical processes from limited information is the Principle of Maximum Caliber. Max Cal can infer both the dynamics and interactions within arbitrarily complex, non-equilibrium systems, albeit in an approximate way.
□ scGMAI: a Gaussian mixture model for clustering single-cell RNA-Seq data based on deep autoencoder
>> https://academic.oup.com/bib/advance-article/doi/10.1093/bib/bbaa316/6029147
scGMAI is a new single-cell Gaussian mixture clustering method based on autoencoder networks and the fast independent component analysis (FastICA).
scGMAI utilizes autoencoder networks to reconstruct gene expression values from scRNA-Seq data and FastICA is used to reduce the dimensions of reconstructed data.
□ Assembling Long Accurate Reads Using de Bruijn Graphs
>> https://www.biorxiv.org/content/10.1101/2020.12.10.420448v1.full.pdf
an efficient jumboDB algorithm for constructing the de Bruijn graph for large genomes and large k-mer sizes and the LJA genome assembler that error-corrects HiFi reads and uses jumboDB to construct the de Bruijn graph on the error-corrected reads.
Since the de Bruijn graph constructed for a fixed k-mer size is typically either too tangled or too fragmented, LJA uses a new concept of a multiplex de Bruijn graph.
□ SCCNV: A Software Tool for Identifying Copy Number Variation From Single-Cell Whole-Genome Sequencing
>> https://www.frontiersin.org/articles/10.3389/fgene.2020.505441/full
Several statistical models have been developed for analyzing sequencing data of bulk DNA, for example, Circular Binary Segmentation (CBS), Mean Shift-Based (MSB) model, Shifting Level Model (SLM), Expectation Maximization (EM) model, and Hidden Markov Model (HMM).
SCCNV is a read-depth based approach with adjustment for the WGA bias. it controls not only bias during sequencing and alignment, e.g., bias associated with mappability and GC content, but also the locus-specific amplification bias.

□ A generative spiking neural-network model of goal-directed behaviour and one-step planning
>> https://journals.plos.org/ploscompbiol/article?id=10.1371/journal.pcbi.1007579
The first hypothesis allows the architecture to learn the world model in parallel with its use for planning: a new arbitration mechanism decides when to explore, for learning the world model, or when to exploit it, for planning, based on the entropy of the world model itself.
The entropy threshold decreases linearly with each planning cycle so that the exploration component is eventually called to select the action if the planning process fails to reach the goal multiple time.

□ Probabilistic Contrastive Principal Component Analysis
>> https://arxiv.org/pdf/2012.07977.pdf
PCPCA, a model-based alterna- tive to contrastive principal component analysis (CPCA). model is both generative and discriminative, PCPCA provides a model based approach that allows for uncertainty quantification and principled inference.
PCPCA can be applied to a variety of statistical and machine learning problem domains including dimension reduction, synthetic data generation, missing data imputation, and clustering.

□ scCODA: A Bayesian model for compositional single-cell data analysis
>> https://www.biorxiv.org/content/10.1101/2020.12.14.422688v1.full.pdf
scCODA, a Bayesian approach for cell type composition differential abundance analysis to further address the low replicate issue.
scCODA framework models cell type counts with a hierarchical Dirichlet-Multinomial distribution that accounts for the uncertainty in cell type proportions and the negative correlative bias via joint modeling of all measured cell type proportions instead of individual ones.