
(https://vimeo.com/244965984)

□ RENDOR: Reverse network diffusion to remove indirect noise for better inference of gene
>>
https://academic.oup.com/bioinformatics/advance-article/doi/10.1093/bioinformatics/btae435/7705978
RENDOR (REverse Network Diffusion On Random walks) formulates a network diffusion model under the graph-theory framework to capture indirect noises and attempts to remove these noises by applying reverse network diffusion.
RENDOR excels in modeling high-order indirect influences, it normalizes the product of edge weights by the degree of the nodes in the path, thereby diminishing the significance of paths with higher intermediate node degrees. RENDOR can use the inverse diffusion to denoise GRNs.

□ ADM: Adaptive Graph Diffusion for Meta-Dimension Reduction
>>
https://www.biorxiv.org/content/10.1101/2024.06.28.601128v1
ADM, a novel meta-dimension reduction and visualization technique based on information diffusion. For each individual dimension reduction result, ADM employs a dynamic Markov process to simulate the information propagation and sharing between data points.
ADM introduces an adaptive mechanism that dynamically selects the diffusion time scale. ADM transforms the traditional Euclidean space dimension reduction results into an information space, thereby revealing the intrinsic manifold structure of the data.

□ Pangenome graph layout by Path-Guided Stochastic Gradient Descent
>>
https://academic.oup.com/bioinformatics/advance-article/doi/10.1093/bioinformatics/btae363/7705520
PG-SGD (Path-Guided Stochastic Gradient Descent) uses the genomes, represented in the pangenome graph as paths, as an embedded positional system to sample genomic distances between pairs of nodes.
PG-SGD computes the pangenome graph layout that best reflects the nucleotide sequences. PG-SGD can be extended in any number of dimensions. It can be seen as a graph embedding algorithm that converts high-dimensional, sparse pangenome graphs into continuous vector spaces.

□ BiRNA-BERT Allows Efficient RNA Language Modeling with Adaptive Tokenization
>>
https://www.biorxiv.org/content/10.1101/2024.07.02.601703v1
BiRNA-BERT, a 117M parameter Transformer encoder pretrained with our proposed tokenization on 36 million coding and non-coding RNA sequences. BiRNA-BERT uses Byte Pair Encoding(BPE) tokenization which allows to merge statistically significant residues into a single token.
BiRNA-BERT uses Attention with Linear Biases (ALiBi) which allows the context window to be extended without retraining and can dynamically choose between nucleotide-level (NUC) and BPE tokenization based on the input sequence length.

□ GeneLLM: A Large cfRNA Language Model for Cancer Screening from Raw Reads
>>
https://www.biorxiv.org/content/10.1101/2024.06.29.601341v1
GeneLLM (Gene Large Language Model), an innovative transformer-based approach that delves into the genome's 'dark matters' by processing raw cRNA sequencing data to identify 'pseudo-biomarkers' independently, without relying on genome annotations.
GeneLLM can reliably distinguish between cancerous and non-cancerous fRNA samples. Pseudo-biomarkers are used to allocate feature vectors from the given patient. Stacks of multi-scale feature extractors are employed to uncover deep, hidden information within the gene features.

□ GenomeDelta: detecting recent transposable element invasions without repeat library
>>
https://www.biorxiv.org/content/10.1101/2024.06.28.601149v1.full.pdf
GenomeDelta identifies sample-specific sequences, such as recently invading TEs, without prior knowledge of the sequence. can thus be used with model and non-model organisms.
Beyond identifying recent TE invasions, GenomeDelta can detect sequences with spatially heterogeneous distributions, recent insertions of viral elements and recent lateral gene transfers.

□ e3SIM: epidemiological-ecological-evolutionary simulation framework for genomic epidemiology
>>
https://www.biorxiv.org/content/10.1101/2024.06.29.601123v1
e3SIM (epidemiological-ecological-evolutionary simulator), an open-source framework that concurrently models the transmission dynamics and molecular evolution of pathogens within a host population while integrating environmental factors.
e3SIM incorporates compartmental models, host-population contact networks, and quantitative-trait models for pathogens. e3SIM uses NetworkX for backend random network generation, supporting Erdós-Rényi, Barabási-Albert, and random-partition networks.
SeedGenerator performs a Wright-Fisher simulation, using a user-specified mutation rate and effective population size, starting from the reference genome and running for a specified number of generations.

□ otopia: A scalable computational framework for annotation-independent combinatorial target identification in scRNA-seq databases
>>
https://www.biorxiv.org/content/10.1101/2024.06.24.600275v1
otopia, a computational framework designed for efficiently querying large-scale SCRNA-seq databases to identify cell populations matching single targets, as well as complex combinatorial gene expression patterns. otopia uses precomputed neighborhood graphs.
Each vertex represents a single cell, and the graph collectively accounts for all the cells. The expression pattern matching score is defined as the fraction of cells among its K-NN that match the pattern. If a cell does not match the target pattern, its score is set to zero.

□ PIE: A Computational Approach to Interpreting the Embedding Space of Dimension Reduction
>>
https://www.biorxiv.org/content/10.1101/2024.06.23.600292v1
PIE (Post-hoc Interpretation of Embedding) offers a systematic post-hoc analysis of embeddings through functional annotation, identifying the biological functions associated with the embedding structure. PIE uses Gene Ontology Biological Process to interpret these embeddings.
PIE filters informative gene vectors. PlE maps the selected genes to the embedding space using projection pursuit. Projection pursuit determines a linear projection that maximizes the association between the embedding coordinates and each gene vector.
The normalized weighting vectors represent the corresponding genes on a unit circle/sphere in the embedding space. PIE calculates the eigengene by integrating the expression patterns of these overlapping genes. The eigengenes are then mapped to the embedding space.

□ HyDRA: a pipeline for integrating long- and short-read RNAseq data for custom transcriptome assembly
>>
https://www.biorxiv.org/content/10.1101/2024.06.24.600544v1
HyDRA (Hybrid de novo RNA assembly), a true-hybrid pipeline that integrates short- and long-read RNAseq data for de novo transcriptome assembly, with additional steps for IncRNA discovery. HyDRA combines read treatment, assembly, filtering and parallel quality.
HyDRA corrects sequencing errors by handling low-frequency k-mers and removing contaminants. It assembles the filtered and corrected reads and further processes the resulting assembly to discover a high-confidence set of lncRNAs supported by multiple machine learning models.
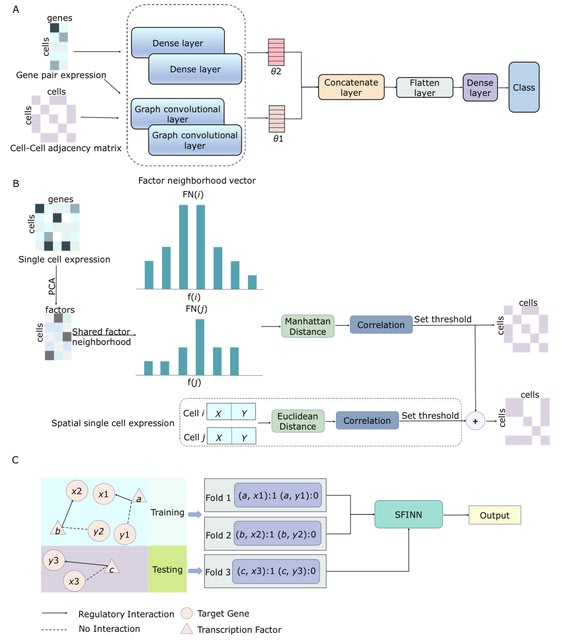
□ SFINN: inferring gene regulatory network from single-cell and spatial transcriptomic data with shared factor neighborhood and integrated neural network
>>
https://academic.oup.com/bioinformatics/advance-article/doi/10.1093/bioinformatics/btae433/7702330
SFINN is a gene regulatory network construction algorithm. SFINN uses a cell neighborhood graph generated from shared factor neighborhood strategy and gene pair expression data as input for the integrated neural network.
SFINN fuses the cell-cell adjacency matrix generated by shared factor neighborhood strategy and that generated using cell spatial location. These are fed into an integrated neural network consisting of a graph convolutional neural network and a fully-connected neural network.
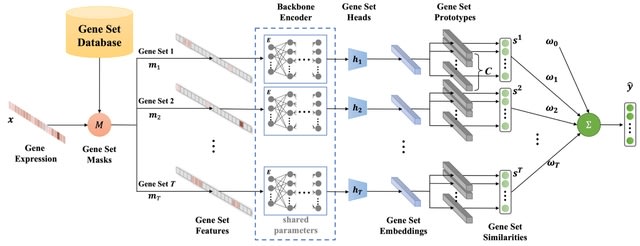
□ DeepGSEA: Explainable Deep Gene Set Enrichment Analysis for Single-cell Transcriptomic Data
>>
https://academic.oup.com/bioinformatics/advance-article/doi/10.1093/bioinformatics/btae434/7702331
DeepSEA, an explainable deep gene set enrichment analysis approach which leverages the expressiveness of interpretable, prototype-based neural networks to provide an in-depth analysis of GSE.
DeepGSEA learns common encoding knowledge shared across gene sets. It learns latent vectors corresponding to the centers of Gaussian distributions, called prototypes, each representing a cell subpopulation in the latent space of gene sets.

□ GeneCOCOA: Detecting context-specific functions of individual genes using co-expression data
>>
https://www.biorxiv.org/content/10.1101/2024.06.27.600936v1
GeneCOCOA (comparative co-expression anaylsis focussed on a gene of interest) has been developed as an integrative method which aims to apply curated knowledge to experiment-specific expression data in a gene-centric manner based on a robust bootstrapping approach.
The input to GeneCOCOA is a list of curated gene sets, a gene-of-interest (GOI) that the user wishes to interrogate, and a gene expression matrix of sample * gene. Genes are sampled and used as predictor variables in a linear regression modelling the expression of the GOI.

□ PredGCN: A Pruning-enabled Gene-Cell Net for Automatic Cell Annotation of Single Cell Transcriptome Data
>>
https://academic.oup.com/bioinformatics/advance-article/doi/10.1093/bioinformatics/btae421/7699793
PredGCN incorporates a Coupled Gene-Cell Net (CGCN) to enable representation learning and information storage. PredGCN integrates a Gene Splicing Net (GSN) / a Cell Stratification Net / a Pruning Operation to dynamically tackle the complexity of heterogeneous cell identification.
PredGCN constructs a GSN which synergistic five discrete feature extraction modalities to selectively assemble discriminative / integral redundant genes. It resorts variance-based hypothesis testing to actualize feature selection by evaluating inter-gene correlation structures.

□ RTF: An R package for modelling time course data
>>
https://www.biorxiv.org/content/10.1101/2024.06.21.599527v1
RTF(The retarded transient function) estimates the best-fit RTF parameters for the provided input data and can be run in 'singleDose' or 'doseDependent' mode, depending on whether signalling data at multiple doses are available.
All parameters are jointly estimated based on maximum likelihood by applying multi-start optimization. The sorted multi-start optimization results are visualized in a waterfall plot, where the occurrence of a plateau for the best likelihood value indicates the global optimum.

□ ema-tool: a Python Library for the Comparative Analysis of Embeddings from Biomedical Foundation Models
>>
https://www.biorxiv.org/content/10.1101/2024.06.21.600139v1
ema-tool, a Python library designed to analyze and compare embeddings from different models for a set of samples, focusing on the representation of groups known to share similarities.
ema-tool examines pair-wise distances to uncover local and global patterns and tracks the representations and relationships of these groups across different embedding spaces.

□ Fast-scBatch: Batch Effect Correction Using Neural Network-Driven Distance Matrix Adjustment
>>
https://www.biorxiv.org/content/10.1101/2024.06.25.600557v1
Fast-scBatch to correct batch effects. It bears some resemblance to scBatch in that it also uses a two-phase approach, and starts with the corrected correlation matrix in phase.
On the other hand, the second phase of restoring the count matrix is newly designed to incorporate the idea of using dominant latent space in batch effect removal, and a customized gradient descent-supported algorithm.

□ Evolving reservoir computers reveals bidirectional coupling between predictive power and emergent dynamics
>>
https://arxiv.org/abs/2406.19201
Mimicking biological evolution, in evolutionary optimization a population of individuals (here RCs) with randomly initialized hyperparameter configurations is evolved towards a specific optimization objective.
This occurs over the course of many generations of competition between individuals and subsequent mutation of the hyperparameter configurations. They evolved RCs with two different objective functions to maximise prediction performance, and to maximise causal emergence.

□ GeneRAG: Enhancing Large Language Models with Gene-Related Task by Retrieval-Augmented Generation
>>
https://www.biorxiv.org/content/10.1101/2024.06.24.600176v1
Retrieval-Augmented Generation (RAG) dynamically retrieves relevant information from external databases, integrating this knowledge into the generation process to produce more accurate and contextually appropriate responses.
GENERAG, a framework that enhances LLMs' gene-related capabilities using RAG and the Maximal Marginal Relevance (MMR) algorithm. These embeddings are vector representations of the gene data, capturing the semantic meaning of the information.

□ scClassify2: A Message Passing Framework for Precise Cell State Identification
>>
https://www.biorxiv.org/content/10.1101/2024.06.26.600770v1
scClassify2, a cell state identification method based on log-ratio values of gene expression, a message passing framework with dual-layer architecture and ordinal regression. scClassify2 effectively distinguishes adjacent cell states with similar gene expression profiles.
The MPNN model of scClassify2 has an encoder-decoder architecture. The dual-layer encoder absorbs nodes and edges of the cell graph to gather messages from neighbourhoods and then alternatively updates nodes and edges by these messages passing along edges.
After aligning all input vectors, scClassify2 concatenate every two node vectors w/ the edge vector connecting them and calculate the message of this edge by a perceptron. Then scClassify2 updates node vectors using this message by a residual module w/ normalisation and dropout.
scClassify2 recalculates the message via another similar perceptron and then update edge vectors this time using new messages. The decoder takes nodes and edges from the encoder and computes messages along edges. The decoder reconstructs the distributed representation of genes.

□ STAN: a computational framework for inferring spatially informed transcription factor activity across cellular contexts
>>
https://www.biorxiv.org/content/10.1101/2024.06.26.600782v1
STAN (Spatially informed Transcription factor Activity Network), a linear mixed-effects computational method that predicts spot-specific, spatially informed TF activities by integrating curated gene priors, mRNA expression, spatial coordinates, and morphological features.
STAN uses a kernel regression model, where we created a spot-specific TF activity matrix, that is decomposed into two terms: one required to follow a spatial pattern (Wsd) generated using a kernel matrix and another that is unconstrained but regularized using the L2-norm.

□ MotifDiff: Ultra-fast variant effect prediction using biophysical transcription factor binding models
>>
https://www.biorxiv.org/content/10.1101/2024.06.26.600873v1
motifDiff, a novel computational tool designed to quantify variant effects using mono and di-nucleotide position weight matrices that model TF-DNA interaction.
motifDiff serves as a foundational element that can be integrated into more complex models, as demonstrated by their application of linear fine-tuning for tasks downstream of TF binding, such as identifying open chromatin regions.

□ Poregen: Leveraging Basecaller's Move Table to Generate a Lightweight k-mer Model
>>
https://www.biorxiv.org/content/10.1101/2024.06.30.601452v1
Poregen extracts current samples for each k-mer based on a provided alignment. The alignment can be either a signal-to-read alignment, such as a move table, or a signal-to-reference alignment, like the one generated by Nanopolish/F5c event-align.
The move table can be either the direct signal-to-read alignment or a signal-to-reference alignment derived using Squigualiser reform and realign. Poregen takes the raw signal in SLOW5 format, the sequence in FASTA format, and the signal-to-sequence in SAM or PAF formats.

□ FLAIR2: Detecting haplotype-specific transcript variation in long reads
>>
https://genomebiology.biomedcentral.com/articles/10.1186/s13059-024-03301-y
FLAIR2 can approach phasing variants in a manner that is agnostic to ploidy: from the isoform-defining collapse step, FLAIR2 generates a set of reads assigned to each isoform.
FLAIR2 tabulates the most frequent combinations of variants present in each isoform from its supporting read sequences; so isoforms that have sufficient read support for a particular haplotype or consistent collection of variants are determined.

□ SCREEN: a graph-based contrastive learning tool to infer catalytic residues and assess mutation tolerance in enzymes
>>
https://www.biorxiv.org/content/10.1101/2024.06.27.601004v1
SCREEN constructs residue representations based on spatial arrangements and incorporates enzyme function priors into such representations through contrastive learning.
SCREEN employs a graph neural network that models the spatial arrangement of active sites in enzyme structures and combines data derived from enzyme structure, sequence embedding and evolutionary information obtained by using BLAST and HMMER.

□ SGCP: a spectral self-learning method for clustering genes in co-expression networks
>>
https://bmcbioinformatics.biomedcentral.com/articles/10.1186/s12859-024-05848-w
SGCP (self-learning gene clustering pipeline), a spectral method for detecting modules in gene co-expression networks. SGCP incorporates multiple features that differentiate it from previous work, including a novel step that leverages gene ontology (GO) information in a self-leaning step.
SGCP yields modules with higher GO enrichment. Moreover, SGCP assigns highest statistical importance to GO terms that are mostly different from those reported by the baselines.
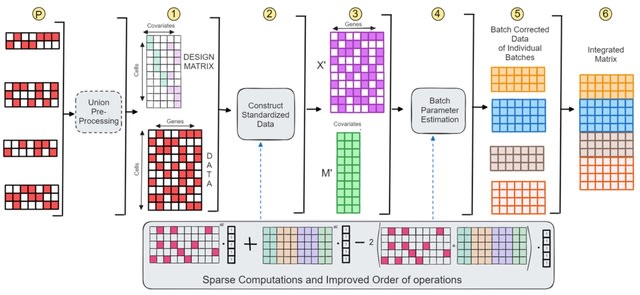
□ SCEMENT: Scalable and Memory Efficient Integration of Large-scale Single Cell RNA-sequencing Data
>>
https://www.biorxiv.org/content/10.1101/2024.06.27.601027v1
SCEMENT (SCalablE and Memory-Efficient iNTegration), a new parallel algorithm builds upon and extends the linear regression model previously applied in ComBat, to an unsupervised sparse matrix setting to enable accurate integration of diverse and large collections of single cell RNA-sequencing data.
SCEMENT improves a sparse implementation of the Empirical Bayes-based integration method, and maintains sparsity of matrices throughout and avoiding dense intermediate matrices through algebraic manipulation of the matrix equations.
SCEMENT employs an efficient order of operations that allows for accelerated computation of the batch integrated matrix, and a scalable parallel implementation that enables integration of diverse datasets to more than four millions cells.

□ StarSignDNA: Signature tracing for accurate representation of mutational processes
>>
https://www.biorxiv.org/content/10.1101/2024.06.29.601345v1
StarSignDNA, an NMF model that offers de novo mutation signature extraction. The algorithm combines the use of regularisation to allow stable estimates with low sample sizes with the use of a Poisson model for the data to accommodate low mutational counts.
StarSignDNA utilizes LASSO regularization to minimize the spread (variance) in exposure estimates. StarSignDNA provides confidence levels on the predicted processes, making it suitable for single-patient evaluation of mutational signatures.
StarSignDNA combines unsupervised cross-validation and the probability mass function as a loss function to select the best combination of the number of signatures and regularisation parameters. The StarSignDNA algorithm avoids introducing bias towards unknown signatures.

□ MetaGXplore: Integrating Multi-Omics Data with Graph Convolutional Networks for Pan-cancer Patient Metastasis Identification
>>
https://www.biorxiv.org/content/10.1101/2024.06.30.601445v1
MetaGXplore integrates Graph Convolutional Networks (GCNs) with multi-omics pan-cancer data to predict metastasis. MetaGXplore was trained and tested on a dataset comprising 754 samples from 11 cancer types, each with balanced evidence of metastasis and non-metastasis.
MetaGXplore employs Graph Mask and Feature Mask methods from GNNExplainer. These two masks are treated as trainable matrices, randomly initialized, and combined with the original graph through element-wise multiplication.

□ TEtrimmer: a novel tool to automate the manual curation of transposable elements
>>
https://www.biorxiv.org/content/10.1101/2024.06.27.600963v2
TEtrimmer employs the clustered, extended and cleaned MSAs to generate consensus sequences for the definition of putative TE boundaries.
Then, potential terminal repeats are identified, and a prediction of open reading frames (ORFs) and protein domains on the basis of the protein families database (PFAM) are conducted.
Subsequently, TE sequences are classified and an output evaluation is performed mainly based on the existence of terminal repeats, and the full length BLASTN hit numbers.

□ Rockfish: A transformer-based model for accurate 5-methylcytosine prediction from nanopore sequencing
>>
https://www.nature.com/articles/s41467-024-49847-0
Rockfish predicts read-level 5mC probability for CpG sites. The model consists of signal projection and sequence embedding layers, a deep learning Transformer model used to obtain contextualized signal and base representation and a modification prediction head used for classification.
Attention layers in Transformer learn optimal contextualized representation by directly attending to every element in the signal and nucleobase sequence. Moreover, the attention mechanism corrects any basecalling and alignment errors by learning optimal signal-to-sequence alignment.

□ GTestimate: Improving relative gene expression estimation in scRNA-seq using the Good-Turing estimator
>>
https://www.biorxiv.org/content/10.1101/2024.07.02.601501v1
GTestimate is a scRNA-seq normalization method. In contrast to other methods it uses the Simple Good-Turing estimator for the per cell relative gene expression estimation.
GTestimate can account for the unobserved genes and avoid overestimation of the observed genes. At default settings it serves as a drop-in replacement for Seurat's NormalizeData.

□ BaCoN (Balanced Correlation Network) improves prediction of gene buffering
>>
https://www.biorxiv.org/content/10.1101/2024.07.01.601598v1
BaCoN (Balanced Correlation Network), a method to correct correlation-based networks post-hoc. BaCoN emphasizes specific high pair-wise coefficients by penalizing values for pairs where one or both partners have many similarly high values.
BaCoN takes a correlation matrix and adjusts the correlation coefficient between each gene pair by balancing it relative to all coefficients each gene partner has with all other genes in the matrix.