مشاهد حصرية من الفضاء للإعصار المداري في بحر العرب.. من محطة الفضاء الدولية نقدر نتابع ظواهر طبيعية كثيرة، ونتعاون مع الخبراء على الأرض بمجال رصد الأحوال الجوية.. 🌩️🌀الله يحفظ الجميع pic.twitter.com/QlpWDOz0n0
— Sultan AlNeyadi (@Astro_Alneyadi) June 13, 2023
□ Chicane / “ Mass Bloom Beach”
From the album “Nevertheless”
□ Atli Örvarsson / “Silo”

□ 『SILO』(Apple TV+)
>> https://tv.apple.com/us/show/silo
Apple TV+ Original
Created by Graham Yost
Based on the book by Hugh Howey
Music by Atli Örvarsson
Hugh HoweyのSFディストピア小説の映像化。 近未来、外界から隔絶された地下施設『サイロ』に追いやられた人類。レガシーシステムの継承やクローズドコミュニティの瑕疵など、当世的な社会問題に対する示唆にも富む。Atli Örvarssonによるダークで重厚な音楽が素晴らしい
シーズンフィナーレ。最後まで重々しく緊迫感を途切れさせない秀作だった。地下施設の閉鎖的描写やセットのディテールが素晴らしいし、レベッカ・ファーガソンの「真実に迫る職人気質な機械工」というキャラに対する没入ぶりがすごかった
壮大に謎を残したままのエンディングで、シーズン2も楽しみだけど、このまま終わったとしてもディストピアSFとしては美しい幕引きだと思う。




□ 『The Little Mermaid』(2023)
>> https://movies.disney.com/the-little-mermaid-2023
Directed by Rob Marshall
Screenplay by David Magee
Based on the Fairy tale by Hans Christian Andersen
Based on Disney Animation screenplay by John Musker / Ron Clements
Music by Alan Menken
Cinematography by Dion Beebe
Cast: Ariel / Halle Bailey, Eric / Jonah Hauer-King,
Ursula / Melissa McCarthy, Triton / Javier Bardem
Sebastian / Daveed Diggs, Flounder / Jacob Tremblay
Scuttle / Awkwafina
"But a mermaid has no tears, and therefore she suffers so much more."
───Hans Christian Andersen, The Litle Mermaid
夢の国に苦難は付き物だ。人は自分らしさを失った時こそ、自分らしさを試されるのだ。挫けて諦めて、何も信じられなくなった差し伸べられる手は、自分自身が築いてきた信頼、直向きな行動に導かれて来たものに他ならないのだから
□ Halle - Part of Your World (From "The Little Mermaid"/Visualizer Video)



□ BertNDA: a Model Based on Graph-Bert and Multi-scale Information Fusion for ncRNA-disease Association Prediction
>> https://www.biorxiv.org/content/10.1101/2023.05.18.541387v1
BertNDA employs Laplace transform of graph structure and WL(Weisfeiler-Lehman) absolute role coding to extract global information. Construct a connectionless subgraph to aggregate neighbor feature to identify local information.
An EMLP (Element-weight MLP) structure is adopted to obtain the multi-scale feature representation of node. Furtherly, nodes are encoded using Transformer-encoder structure. BertNDA acquires the semantic similarity and Gaussian interaction profile kernel similarity matrix.
BertNDA calculates the Laplace matrix on the structure of the entire graph after data preprocessing. Eigenvectors are defined via the factorization of the graph Laplacian matrix. The absolute role embedding of nodes is calculated by using the WL algorithm.

□ Geneformer: Transfer learning enables predictions in network biology
>> https://www.nature.com/articles/s41586-023-06139-9
Geneformer, a context-aware, attention-based deep learning model, pretrained on a large-scale corpus of about 30 million single-cell transcriptomes to enable context-specific predictions in settings with limited data in network biology.
Geneformer encodes network hierarchy in the attention weights of the model in a completely self-supervised manner. Fine-tuning towards a diverse panel of downstream tasks relevant to chromatin and network dynamics. Geneformer consistently boosted predictive accuracy.

□ Dimension reduction of dynamics on modular and heterogeneous directed networks
>> https://academic.oup.com/pnasnexus/article/2/5/pgad150/7147610
A method for reducing a given N-dimensional dynamical system on a network into an n-dimensional one whose variables, the observables, represent weighted averages of the node activities A reduced adjacency matrix and an approximate system of ODEs for the observables’ evolution.
Calculating the reduction vectors that are used to construct the observables from the node activities. These vectors fully determine the reduced approximate dynamics, incl. a reduced adjacency matrix that specifies the magnitude of the coupling between observables.

□ xRead: a coverage-guided approach for scalable construction of read overlapping graph
>> https://www.biorxiv.org/content/10.1101/2023.05.23.541864v1
×Read keeps a global graph data structure to record read overlaps during the iterative process. The produced alignment skeletons are converted to read overlapping information and supplied to the data structure incrementally.
For a given query read, the produced alignment skeletons that meet one of the following three conditions are filtered out at first since they could be false positives caused by sequencing errors or repeats in local genomic regions:
×Read (re-)estimates read coverages w/ the updated overlapping information. For a given read, its coverage is estimated by the numbers of the seed reads directly connected to it by the CROs. The reads having CROs to the same seed reads which can be regarded as indirectly aligned.

□ NS-DIMCORN: Ordinary differential equations to construct invertible generative models of cell type and tissue-specific regulatory networks
>> https://www.biorxiv.org/content/10.1101/2023.05.18.540731v1
Non-Stiff Dynamic Invertible Model of CO-Regulatory Networks (NS-DIMCORN) defines the genetic nexus underpinning specific cellular functions using invertible warping of flexible multivariate Gaussian distributions by neural Ordinary differential equations.
NS-DIMCORN allows unrestricted neural network architectures. NS-DIMCORN represents different cell states by a continuous latent trajectory and defines a bijective map from the latent learned latent space to data by integrating latent variables.
NS-DIMCORN yields a continuous-time invertible generative model with unbiased density estimation by one-pass sampling. NS-DIMCORN achieves easy sampling of the continuous trajectories using Hamiltonian Monte Carlo and calculates nonlinear gene dependency.

□ Protpardelle: An all-atom protein generative model
>> https://www.biorxiv.org/content/10.1101/2023.05.24.542194v1
Protpardelle, an all-atom diffusion model of protein structure, which instantiates a “superposition” over the possible sidechain states, and collapses it to conduct reverse diffusion for sample generation.
Protpardelle is capable of co-designing sequence and structure, it remains a structure-primary generative model that produces estimates of the sequence during its sampling trajectory.
Protpardelle does not define any noising process on the sequence; nor is it a joint model in the sense that we are able to marginalize and condition in some way to produce solutions to the sub-tasks of structure and sequence generation and forward and inverse folding.

□ scGPCL: Deep single-cell RNA-seq data clustering with graph prototypical contrastive learning
>> https://academic.oup.com/bioinformatics/advance-article/doi/10.1093/bioinformatics/btad342/7180270
scGPCL encodes the cell representations based on Graph Neural Networks (GNNs), and utilizes prototypical contrastive learning scheme to learn cell representations by pushing apart semantically disimillar pairs and pulling together similar ones.
scGPCL adopts instance-wise contrastive learning scheme to fully leverage the relational information as well as prototypical contrastive loss to alleviate the limitation of instance-wise contrastive loss.
scGPCL with a cell-gene graph as the input consistently outperforms that w/ a cell-cell graph, which demonstrates that the cell-gene graph better helps to infuse the inherent relational information b/n cells. scGPCL consistently succeeds in learning the cell representation space.

□ scMTNI: Inference of cell type-specific gene regulatory networks on cell lineages from single cell omic datasets
>> https://www.nature.com/articles/s41467-023-38637-9
scMTNI models a GRN as a Dependency network, a probabilistic graphical model with random variables representing genes and regulators, such as transcription factors (TFs) and signaling proteins.
scMTNI’s multi-task learning framework incorporates a probabilistic lineage tree prior. It models the change of a GRN from a start state (e.g., progenitor cell state) to an end state (e.g., more differentiated state) as a series of individual edge-level probabilistic transitions.

□ scSHARP: Consensus Label Propagation with Graph Convolutional Networks for Single-Cell RNA Sequencing Cell Type Annotation
>> https://academic.oup.com/bioinformatics/advance-article/doi/10.1093/bioinformatics/btad360/7189733
scSHARP uses a Graph Convolutional Network (GCN) as a mechanism to propagate labels from confidently labeled cells to unconfidently labeled cells. Each GCN used EdgeConv feature propagation between each node and its k closest neighbors, with distance determined dynamically.
scSHARP employes DeepLIFT as an effective Gradient-based interpretation tool for the GCN model. The k hyperparameter and convergence method for the non-parametric neighbor majority approach was chosen with the same validation set used for GCN hyperparameter optimization.

□ IndepthPathway: an integrated tool for in-depth pathway enrichment analysis based on single cell sequencing data
>> https://academic.oup.com/bioinformatics/advance-article/doi/10.1093/bioinformatics/btad325/7181277
A Weighted Concept Signature Enrichment Analysis (WCSEA) specialized for pathway enrichment analysis from single cell transcriptomics (scRNA-seq).
WCSEA took a broader approach for assessing the functional relations of pathway gene sets to differentially expressed genes, and leverage the cumulative signature of molecular concepts characteristic of the highly differentially expressed genes.
IndepthPathway presents outstanding stability and depth in pathway enrichment results under stochasticity of the data, thus will substantially improve the scientific rigor of the pathway analysis for single cell sequencing data.

□ ReX: an integrative tool for quantifying and optimizing measurement reliability for the study of individual differences
>> https://www.nature.com/articles/s41592-023-01901-3
Reliability eXplorer (ReX), to facilitate the examination of individual variation and reliability as well as the effective direction for optimization of measuring individual differences in biomarker discovery.
Gradient flows, a two-dimensional field map-based approach to identifying and representing the most effective direction for optimization when measuring individual differences, which is implemented in ReX.

□ Reassessing the modularity of gene co-expression networks using the Stochastic Block Model
>> https://www.biorxiv.org/content/10.1101/2023.05.31.542906v1
The Weighted degree corrected stochastic block model with no free parameters, can find many more gene clusters than competing methods. Second, that such gene clusters are biologically meaningful as revealed by highly specific gene ontology enrichment.
The mean and the variance of the observed edge weights b/n 2 blocks are a function only of the block structure, i.e., genes in the same block have a similar probability of being connected to other genes and the value of the weights in these edges comes from the same distribution.

□ DeepRaccess: High-speed RNA accessibility prediction using deep learning
>> https://www.biorxiv.org/content/10.1101/2023.05.25.542237v1
DeepRaccess, a fast accessibility prediction tool based on deep learning-based software acceleration. DeepRaccess can moderately reproduce the results of Raccess, an existing RNA accessibility calculation method, with high accuracy on both simulation and empirical datasets.
DeepRaccess divides the sequence into subsequences. DeepRaccess predicts the accessibility of these subsequences and integrated them with the accessibility of the full-length RNA. DeepRaccess ignored the accessibility of the 55-base region from the end of each subsequence.

□ Optipyzer: A fast and flexible multi-species codon optimization server
>> https://www.biorxiv.org/content/10.1101/2023.05.22.541759v1
Optipyzer is a new fast and effective multi-species codon optimization server capable of optimizing recombinant DNA sequences for multiple target organisms simultaneously.
Optipyzer leverages the most up-to-date codon usage data through the HIVE-Codon Usage Tables database. The averaged table is used to construct an optimized query using a stochastic selection process and the relative codon adaptation index to ensure a proper expression profile.

□ PatternCode: Design of optimal labeling patterns for optical genome mapping via information theory
>> https://www.biorxiv.org/content/10.1101/2023.05.23.541882v1
An information-theoretic model of optical genome mapping (OGM), which enables the prediction of its accuracy and the design of optimal labeling patterns for specific applications and target organism genomes.
It depends on only four parameters: the target genome length, the DNA fragment length, and two easily estimated parameters: the label detection likelihood, estimated from experimental genome-aligned DNA fragment images, and the labeling pattern distribution.
This enables the design of better OGM experiments, and allows for the intuitive understanding of the importance of different parameters on the accuracy, such as the logarithmic dependence on the target genome length versus the polynomial dependence on the fragment length.
Additionally, the model enables fast computation due to its simple analytical form, allowing for the design of protocols where multiple patterns are labeled with multiple labeling reagents through combinatorial optimization of pattern combination selection.

□ On the invariant subspace problem in Hilbert spaces
>> https://arxiv.org/abs/2305.15442
Every bounded linear operator T on a Hilbert space H has a closed non-trivial invariant subspace. There are situations when we cannot just use the Main Construction to reach a non-cyclic vector.
If we had norm convergence we could continue beyond (εθ)'. We "get stuck" if (am), (bm) (Ko-1, (kim)m»1) become close to being linearly dependent. We create εθ's arbitrarily near to 0, from which we restart the Main Construction.

□ MEGABYTE: Predicting Million-byte Sequences with Multiscale Transformers
>> https://arxiv.org/abs/2305.07185
MEGABYTE is an autoregressive model for efficiently modeling long input sequences. MEGABYTE is able to handle all sequence lengths with a single forward pass of up to 1.2M tokens.
MEGABYTE uses an efficient decoder model by using a intra-patch transformer to predict each sequence element's likelihood, and offseting the inputs to the two models to avoid leaking information.

□ cPeaks: Consensus peaks of chromatin accessibility in the human genome
>> https://www.biorxiv.org/content/10.1101/2023.05.30.542889v1
Predicting all potential open regions in the human genome using cPeaks. It can be regarded as a new set of epigenomic elements in the human genome. cPeaks also have the potential to identify rare cell subtypes that are difficult to be detected using pseudo-bulk peaks.
Each approach provided a genomic region set as a reference for mapping sequencing reads to generate a cell-by-chromatin accessibility feature matrix. cPeaks got similar or better performance in comparison with other feature-defining approaches under all evaluation methods.

□ RaggedExperiment: the missing link between genomic ranges and matrices in Bioconductor
>> https://academic.oup.com/bioinformatics/advance-article/doi/10.1093/bioinformatics/btad330/7174143
RaggedExperiment represents ragged genomic ranges from multiple samples, and to provide flexible and efficient tools for matrix-format summarization across identical ranges in each sample.
RaggedExperiment fills a gap in providing efficient, flexible conversion between "ragged" genomic data and matrix format for which we are not aware of a direct analogy to benchmark against.

□ BRGenomics for analyzing high resolution genomics data in R
>> https://academic.oup.com/bioinformatics/advance-article/doi/10.1093/bioinformatics/btad331/7174141
BRGenomics provides various methods for data importation and processing, read counting and aggregation, spike-in and batch normalization, re-sampling methods for robust “metagene” analyses, and various other functions for cleaning and modifying sequencing and annotation data.
BRGenomics has been used to analyze ATAC-seq, ChIP- seq/ChIP-exo, PRO-seq/PRO-cap, and RNA-seq data; is built to be unobtrusive and maximally compatible with the Bioconductor ecosystem.

□ Matías Gutiérrez
Here’s what coming #NanoporeConf @nanopore

□ GraphSNP: an interactive distance viewer for investigating outbreaks and transmission networks using a graph approach
>> https://bmcbioinformatics.biomedcentral.com/articles/10.1186/s12859-023-05332-x
GraphSNP is an interactive visualisation tool running in a web browser that allows users to rapidly generate pairwise SNP distance networks, investigate SNP distance distributions, identify clusters of related organisms, and reconstruct transmission routes.
GraphSNP generates pairwise Hamming distance from the SNP alignment. GraphSNP provides capability for creating a Minimum Spanning Tree of the resulted clusters using the Kruskal’s algorithm, a transmission tree using the SeqTrack algorithm, and the breadth-first search algorithm.

□ Adversarial training improves model interpretability in single-cell RNA-seq analysis
>> https://www.biorxiv.org/content/10.1101/2023.05.17.541170v1
Adversarial training fortifies a deep learning model, which can be useful for future clinical and health applications, such as diagnostic or prognostic gene expression biomarkers or patient classification, that need to be robust against adversarial attacks.
Projected Gradient Descent (PGD) and Fast Gradient Signed Method (FGSM). These take the trained model and introduce noise in the input data in the direction of the model gradient that has the greatest impact on the model's accuracy.

□ iDeLUCS: A deep learning interactive tool for alignment-free clustering of DNA sequences
>> https://www.biorxiv.org/content/10.1101/2023.05.17.541163v1
iDeLUCS is a standalone software tool that exploits the capabilities of deep learning to cluster genomic sequences. It is agnostic to the data source, making it suitable for genomic sequences taken from any organism in any kingdom of life.
iDeLUCS assigns a cluster identifier to every DNA sequence present in a dataset, while incorporating several built-in visualization tools that provide insights into the underlying training process. iDeLUCS offers an evaluation mode to compare the ground-truth label assignments.
This is accompanied by a visual qualitative assessment of the clustering, through the use of the uniform manifold approximation of the learned lower dimensional embedding. iDeLUCS outputs confidence scores for all of its cluster-label predictions, for enhanced interpretability.

□ Genome Context Viewer (GCV) version 2: enhanced visual exploration of multiple annotated genomes
>> https://academic.oup.com/nar/advance-article/doi/10.1093/nar/gkad391/7173788
Version 2 of the Genome Context Viewer (GCV) – an open-source web application that uses the functional annotations of genes to perform on-demand federated synteny analysis of collections of genomes.
By using functional annotations as the unit of search and comparison, GCV can compute and display multiple regions across several assemblies from different databases in real-time.

□ Benchtop DNA printers are coming soon—and biosecurity experts are worried
>>
https://www.science.org/content/article/benchtop-dna-printers-are-coming-soon-and-biosecurity-experts-are-worried
The current screening system, which is voluntary, “could be upended by benchtop DNA synthesis,” says report co-author Jaime Yassif, vice president for global biological policy and programs at the Nuclear Threat Initiative.
The report recommends that benchtop synthesis devicemakers vet their customers to ensure they are legitimate biotechnology researchers. It also calls for build-in protections, such as software that allows the manufacturer to screen all requests for DNA sequences prior to synthesis.

□ SQANTI3: curation of long-read transcriptomes for accurate identification of known and novel isoforms
>> https://www.biorxiv.org/content/10.1101/2023.05.17.541248v1
SQANTI3 provides an extensive naming framework to characterize transcript model diversity. The incorporates novel metrics and features to better characterize the transcription start and end sites, splice junctions of isoforms, and filter out potential artifacts.
The Rescue module re-evaluates artifacts to suggest a bona fide replacement transcript model and avoid the loss of known genes and transcripts for which evidence of expression exists.
SQANTI3 includes a Random Forest classifier that labels long read transcripts as isoforms or artifacts using SQANTI QC descriptors as predictive variables and a set of user-defined true and false transcripts.

□ Unsupervised single-cell clustering with Asymmetric Within-Sample Transformation and per cluster supervised features selection
>> https://www.biorxiv.org/content/10.1101/2023.05.17.541148v1
The asymmetric transformation is a special winsorization that flattens low-expressed intensities and preserves highly expressed gene levels. An intermediate step removes non-informative genes according to a threshold applied to a per-gene entropy estimate.
Following the clustering, a time-intensive algorithm is shown to uncover the molecular features associated with each cluster. This step implements a resampling algorithm to generate a random baseline to measure up/down-regulated significant genes.
□ 『遺伝情報は誰のものか』問題。DNAは個人の資産か、公衆衛生やセキュリティリスクを内包する資源とするか
□ Mike White QT
>> https://twitter.com/genologos/status/1660414328439287810?s=61&t=YtYFeKCMJNEmL5uKc0oPFg
I’ve never been able to follow this reasoning. If an individual with substantial Native American ancestry wants to contribute their DNA to a genomics project, do they need to get permission from some tribal authority that this person may not even acknowledge?

□ SeATAC: a tool for exploring the chromatin landscape and the role of pioneer factors
>> https://genomebiology.biomedcentral.com/articles/10.1186/s13059-023-02954-5
SeATAC uses a conditional variational autoencoder model to learn the latent representation of ATAC-seq V-plots and outperforms MACS2 and NucleoATAC on six separate tasks.
The SeATAC model uses a V-plot with a width of 640-bp genomic region and a height of 640 bp of fragment sizes that covers nucleosome free reads, mono-nucleosome reads, di-nucleosome reads, and tri-nucleosomes.

□ In silico methods for predicting functional synonymous variants
>> https://genomebiology.biomedcentral.com/articles/10.1186/s13059-023-02966-1
Genscan uses a maximal dependence decomposition (MDD) model, which is a decision tree-based method. Genesplicer combines MDD with Markov models (MM) to capture additional dependencies between neighboring positions.
MES uses maximum entropy principle (MEP) for modeling short sequence motifs found in splice sites while also accounting for higher-order dependencies between adjacent and non-adjacent positions.
usDSM (Deleterious Synonymous Mutation Prediction using Undersampling Scheme) and synVep (Synonymous Variant Effect Predictor) are newer tools that have demonstrated improved proficiencies by implementing undersampling methods and positive-unlabeled learning.

□ GoldRush: Linear time complexity de novo long read genome assembly
>> https://www.nature.com/articles/s41467-023-38716-x
GoldRush, a memory-efficient long-read haploid de novo genome assembler that employs a novel long-read assembly algorithm, which runs in linear time in the number of reads.
GoldPath iterates through the reads, querying each read against a dynamic and probabilistic multi-index Bloom filter data structure in turn, and inserts selected sequence or skips over the read depending on the results of the query to generate multiple silver paths.
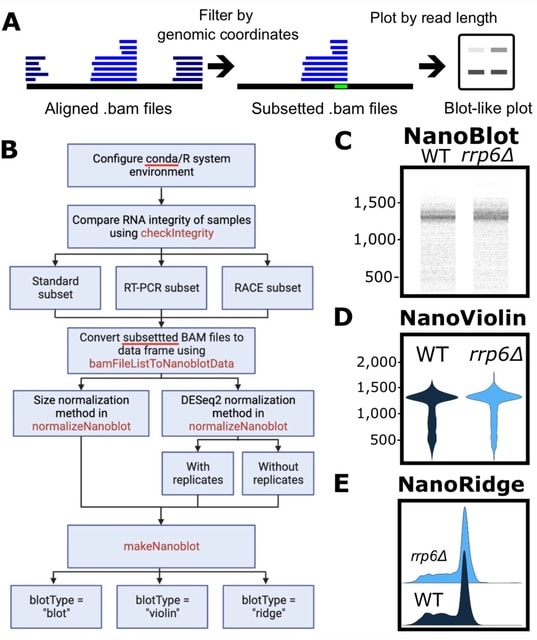
□ NanoBlot: An R-Package for Visualization of RNA Isoforms from Long Read RNA-sequencing Data
>> https://rnajournal.cshlp.org/content/early/2023/05/03/rna.079505.122.abstract
NanoBlot, an open-source, R-package, which generates northern blot and RT-PCR-like images from long-read sequencing data. NanoBlot requires aligned, positionally sorted and indexed BAM files.
NanoBlot can output other visualizations such as violin plots and 3′-RACE-like plots focused on 3′-ends isoforms visualization. The use of the NanoBlot package should provide a simple answer to some of the challenges of visualizing long-read RNA sequencing data.


□ scANNA: Boosting Single-Cell RNA Sequencing Analysis with Simple Neural Attention
>> https://www.biorxiv.org/content/10.1101/2023.05.29.542760v1
scANNA (single-cell Analysis using Neural-Attention) learns salient genes for each cluster enabling accurate / scalable unsupervised annotations. After training scANNA's DL core, the gene attention weights from the Additive Attention Module are used as input for downstream tasks.
scANNA uses the Deep Projection Blocks, which are an ensemble of operators learning a nonlinear mapping between gene scores. This mapping is designed
to increase model capacity and connect the gene associations to the auxiliary objective.

□ COMSE: Analysis of Single-Cell RNA-seq Data Using Community Detection Based Feature Selection
>> https://www.biorxiv.org/content/10.1101/2023.06.03.543526v1
COMSE partitions all genes into different communities in latent space using the Louvain algorithm. A denoising procedure removes noise introduced during sequencing or other procedures. It then selects highly informative genes from each community based on the Laplacian score.
COMSE calculates the Laplacian score with multi-subsample randomization and choose genes with the smallest scores, assuming that data from the same class are often close to each other. COMSE then rank the genes based on gene-gene correlation to remove redundancy.

□ scATAnno: Automated Cell Type Annotation for single-cell ATAC-seq Data
>> https://www.biorxiv.org/content/10.1101/2023.06.01.543296v1
scATAnno, a workflow that directly and automatically annotates scATAC-seq data based on scATAC-seq reference atlases. scATAnno directly uses peaks or CRE genomic regions as input features, eliminating the need to convert the epigenomic features into gene activity scores.
scATAnno uses chromatin state profile of large-scale reference atlas to generate peak signals and reference peaks. scATAnno tackles the high dimensionality of SCATAC-seq data by leveraging spectral embedding to efficiently transform the data into a low dimensional space.
Each query cell is assigned a cell type along with two uncertainty scores: the first uncertainty score is based on the KNN, and the second uncertainty score is derived from a novel computation of the weighted distance between the query cell and reference cell type centroids.

□ Sophia: A Scalable Stochastic Second-order Optimizer for Language Model Pre-training
>> https://arxiv.org/abs/2305.14342
Sophia, Second-order Clipped Stochastic Optimization, a simple scalable second-order optimizer that uses a light-weight estimate of the diagonal Hessian as the pre-conditioner. Sophia only estimates the diagonal Hessian every handful of iterations.
The update is the moving average of the gradients divided by the moving average of the estimated Hessian, followed by element-wise clipping. It controls the worst-case update size and tames the negative impact of non-convexity and rapid change of Hessian along the trajectory.
Sophia has a more aggressive pre-conditioner than Adam Sophia applies a stronger penalization to updates in sharp dimensions (where the Hessian is large) than the flat dimensions (where the Hessian is small), ensuring a uniform loss decrease across all parameter dimensions.

□ AlphaDev: Faster sorting algorithms discovered using deep reinforcement learning
>> https://www.nature.com/articles/s41586-023-06004-9
Formulating the problem of discovering new, efficient sorting algorithms as a single-player game that they refer to as AssemblyGame. The AlphaDev learning algorithm can incorporate both DRL as well as stochastic search optimization algorithms to play AssemblyGame.
The primary AlphaDev representation is based on Transformers. AlphaDev discovered small sorting algorithms from scratch that outperformed previously known human benchmarks. These algorithms have been integrated into the LLVM standard C++ sort library.

□ NOS: diffusioN Optimized Sampling: Protein Design with Guided Discrete Diffusion
>> https://arxiv.org/abs/2305.20009
NOS, a guidance method for discrete diffusion models that follows gradients in the hidden states of the denoising network. NOS can perform design directly in sequence space, circumventing significant limitations of structure-based methods, incl. scarce data and inverse design.
NOS generalizes LaMBO, a Bayesian optimization procedure for sequence design that facilitates multiple objectives and edit-based constraints. The resulting method, LaMBO-2, enables discrete diffusions and stronger performance through a novel application of saliency maps.

□ MISATO - Machine learning dataset for structure-based drug discovery
>> https://www.biorxiv.org/content/10.1101/2023.05.24.542082v1
MISATO, a curated dataset of 20000 experimental structures of protein-ligand complexes, associated molecular dynamics traces, and electronic properties. Semi-empirical quantum mechanics was used to systematically refine protonation states of proteins and small molecule ligands.
Molecular dynamics traces for protein-ligand complexes were obtained in explicit water. The dataset is made readily available to the scientific community via simple python data-loaders. AI baseline models are provided for dynamical and electronic properties.

□ SifiNet: A robust and accurate method to identify feature gene sets and annotate cells
>> https://www.biorxiv.org/content/10.1101/2023.05.24.541352v1
SifiNet (Single-cell feature identification w/ Network topology), a cell-clustering-independent method for directly identifying feature gene sets. SifiNet is based on the observation that co-differentially-expressed genes w/ a cell subpopulation exhibit co-expression patterns.
SifiNet constructs a gene co-expression network and explores its topology to identify feature gene sets. It also applies to scATAC-seq data, generating a gene co-open-chromatin network and exploring network topology to identify epigenomic feature gene sets.

□ scTIE: data integration and inference of gene regulation using single-cell temporal multimodal data
>> https://www.biorxiv.org/content/10.1101/2023.05.18.541381v1
scTIE, an autoencoder-based method for integrating multimodal profiling of scRNA-seq / scATAC-seq data over a time course. scTIE provides the first unified framework for the integration of temporal data and the inference of context-specific GRNs that predict cell fates.
scTIE uses iterative optimal transport (OT) fitting to align cells in similar states between different time points and estimate their transition probabilities. scTIE removes the need for selecting highly variable genes (HVGs) as input through a pair of coupled batchnorm layers.
scTIE provides the means to extract interpretable features from the embedding space by linking the developmental trajectories of cell representations. scTIE formulates a trajectory prediction using the estimated transition probabilities and uses gradient-based saliency mapping.

□ scME: A Dual-Modality Factor Model for Single-Cell Multi-Omics Embedding https://academic.oup.com/bioinformatics/advance-article/doi/10.1093/bioinformatics/btad337/7176368
scME can generate a better joint representation of multiple modalities than those generated by other single-cell multi-omics integration algorithms, which gives a clear elucidation of nuanced differences among cells.
scME relies on clustering to determine the shared and complementary information between modalities. Hence, the parameters of a clustering algorithm, such as resolution of the Leiden algorithm, could affect the efficacy of this algorithm.

□ scBalance – a scalable sparse neural network framework for rare cell type annotation of single-cell transcriptome data
>> https://www.nature.com/articles/s42003-023-04928-6
scBalance, a sparse neural network framework that can automatically label rare cell types in scRNA-seq datasets of all scales. scBalance will automatically choose the weight for each cell type in the reference dataset and construct the training batch.
scBalance leverages the combination of weight sampling and sparse neural network, whereby minor (rare) cell types are more informative without harming the annotation efficiency of the common (major) cell populations.
scBalance will iteratively learn mini batches from a three-layer neural network until the cross-entropy loss converges. In the training stage, scBalance randomly disables neurons in the network.

□ SIMBA: single-cell embedding along with features
>> https://www.nature.com/articles/s41592-023-01899-8
SIMBA is a single-cell embedding method that supports single- or multi-modality analyses. It leverages recent graph embedding techniques to embed cells and genomic features into a shared latent space.
SIMBA introduces several crucial procedures, including Softmax transformation, weight decay for controlling overfitting and entity-type constraints to generate comparable embeddings (co-embeddings) of cells and features and to address unique challenges in single-cell data.

□ gRNAde: Multi-State RNA Design with Geometric Multi-Graph Neural Networks
>> https://arxiv.org/abs/2305.14749
gRNAde, a geometric deep learning-based pipeline for RNA sequence design conditioned on multiple backbone conformations.
gRNAde explicitly accounts for RNA conformational flexibility via a novel multi-Graph Neural Network architecture which independently encodes a set of conformers via message passing, followed by conformer order-invariant pooling and sequence design.

□ Cellenium—a scalable and interactive visual analytics app for exploring multimodal single-cell data
>>
Cellenium, a full-stack scalable visual analytics web application which enables users to semantically integrate and organize all their single-cell RNA-, ATAC- , and CITE-sequencing studies.
Cellenium consists of a central Postgres database for hosting all expression- and meta-data, a Postgraphile based GraphQL API layer. Cellenium precalculates differential gene expressions between each annotated cell type and all other cells.

□ Lineage motifs: developmental modules for control of cell type proportions
>> https://www.biorxiv.org/content/10.1101/2023.06.06.543925v1
Lineage Motif Analysis (LMA), a method that recursively identifies statistically overrepresented patterns of cell fates on lineage trees as potential signatures of committed progenitor states.
LMA is based on motif detection, which has been used to identify the building blocks of complex regulatory networks, DNA sequences, and other biological features.
Motifs could be generated by progenitors intrinsically programmed to autonomously give rise to specific patterns of descendant cell fates. It reflects developmental programs invl. extrinsic cues and cell-cell signaling that generate correlated cell fate patterns on lineage trees.

□ MCPNet: A parallel maximum capacity-based genome-scale gene network construction framework
>> https://academic.oup.com/bioinformatics/advance-article/doi/10.1093/bioinformatics/btad373/7192172
MCP (Maximum Capacity Path) Score, a novel maximum-capacity-path based metric to quantify the relative strengths of direct and indirect gene-gene interactions. MCPNet combines interactions from multiple path lengths using optimized weights identified with partial groundtruth.

□ Spider: a flexible and unified framework for simulating spatial transcriptomics data
>> https://www.biorxiv.org/content/10.1101/2023.05.21.541605v1
Spider generates locations of cells on a plate randomly or in a uniform grid-like pattern. Spider supports various neighborhood metrics, such as k-nearest neighbors or neighbors identified by Delaunay triangulation.

□ SanntiS: Expansion of novel biosynthetic gene clusters from diverse environments
>> https://www.biorxiv.org/content/10.1101/2023.05.23.540769v1
At the core of SanntiS is the detection model, an Artificial Neural Network with a one-dimensional convolutional layer, plus a BiLSTM. The model was developed using linearized sequences of protein annotations based on a subset of InterPro as input.
SanntiS employes a duration robust loss function (RLF). RLF mitigates the issue of class imbalance, which can arise from the disparities in BGC counts by class and the variation in the duration of detection events - the disparities in length across different BGC classes.

□ Identification of Biochemical Pathways Responsible for Distinct Phenotypes Using Gene Ontology Causal Activity Models
>> https://www.biorxiv.org/content/10.1101/2023.05.22.541760v1
Phenotypic variability among affected individuals described as incomplete penetrance and variable expressivity can be the result of interactions between the mutated gene and other genes with which it normally interacts.
Integrating the information about human biology from Reactome with model-organism biology from MGI. It can be used not only to understand the similarities of the pathways but as a testing ground for manipulation of pathways in more experimentally tractable organisms than human.

□ MetaBayesDTA: codeless Bayesian meta-analysis of test accuracy, with or without a gold standard
>> https://bmcmedresmethodol.biomedcentral.com/articles/10.1186/s12874-023-01910-y
MetaBayesDTA uses the bivariate model to conduct analysis assuming a perfect reference test, and users can also conduct univariate meta-regression and subgroup analysis. It uses latent class models (LCMs) to conduct analyses without assuming a perfect gold standard.
MetaBayesDTA allows the user to run models assuming conditional independence or dependence, options for whether to model the reference and index test sensitivities and specificities as fixed or random effects, and can model multiple reference tests using a meta-regression covariate.

□ WebAtlas pipeline for integrated single cell and spatial transcriptomic data
>> https://www.biorxiv.org/content/10.1101/2023.05.19.541329v1
WebAtlas incorporates integrated scRNA-seq, imaging- and sequencing-based ST datasets for interactive web visualisation, enabling cross-query of cell types and gene expressions across modalities.
WebAtlas unifies commonly used atlassing technologies into the cloud-optimised Zarr format and builds on Vitessce to enable remote data navigation. On WebAtlas, single cell and spatial datasets are linked by biomolecular metadata.
Linkage is performed prior to WebAtlas ingestion using existing data integration methods like Cell2location and StabMap that map scRNA-seq cell type references onto ST datasets and impute unobserved gene expression in the latter.

□ ROCCO: A Robust Method for Detection of Open Chromatin via Convex Optimization
>> https://www.biorxiv.org/content/10.1101/2023.05.24.542132v1
ROCCO determines consensus open chromatin regions across multiple samples simultaneously. ROCCO uses robust summary statistics across samples by solving a constrained optimization problem formulated to account for both enrichment & spatial features of open chromatin signal data.
The model accounts for features common to the edges of accessible chromatin regions, which are often hard to determine based on independently determined sample peaks that can vary widely in their genomic locations.

□ FuzzyPPI: Human Proteome at Fuzzy Semantic Space
>> https://www.biorxiv.org/content/10.1101/2023.05.24.541959v1
FuzzyPPI, a fuzzy semantic scoring function using the Gene Ontology (GO) graphs to assess the binding affinity between any two proteins at an organism level.
FuzzyPPI also constructs a fuzzy semantic network at proteome level from the above designed binding affinity function and extraction of meaningful biological insights.

□ Classifying high-dimensional phenotypes with ensemble learning
>> https://www.biorxiv.org/content/10.1101/2023.05.29.542750v1
A meta-analysis of 33 algorithms across 20 datasets containing over 20,000 high-dimensional shape phenotypes using an ensemble learning framework. Both binary and multi-class (e.g., species, genotype, population) classification tasks were considered.
They employs phenotypic datasets containing a range of anatomical data from different organisms with unique class distributions. Blending ensemble approaches involve strategically stacking a set of individual classifiers using a holdout validation set to improve performance.

□ buttery-eel: Accelerated nanopore basecalling with SLOW5 data format
>> https://academic.oup.com/bioinformatics/advance-article/doi/10.1093/bioinformatics/btad352/7186500
Buttery-eel, an open-source wrapper for Oxford Nanopore’s Guppy basecaller that enables SLOW5 data access, resulting in performance improvements that are essential for scalable, affordable basecalling.
Buttery-eel/BLOW5 demonstrates a ~3-fold performance improvement when using FAST basecalling, compared to ~20% improvement with HAC basecalling. This suggests that there is an underlying bottleneck in data access on the PromethION.

□ FAST: Flexible Analysis of Spatial Transcriptomics Data: A Deconvolution Approach
>> https://www.biorxiv.org/content/10.1101/2023.05.26.542550v1
A novel reference-free method based on regularized non-negative matrix factorization (NMF), named Flexible Analysis of Spatial Transcriptomics (FAST), that can effectively incorporate gene expression data, spatial coordinates, and histology information into a unified deconvolution framework.
FADT is adaptable to any graph Laplacian matrix, allowing for flexibility in its application. The second term imposes a constraint on cell proportions, encouraging their summation equals one.

□ autoStreamTree: Genomic variant data fitted to geospatial networks
>> https://www.biorxiv.org/content/10.1101/2023.05.27.542562v1
autoStreamTree provides a companion library of functions for calculating various measures of genetic distances among individuals or populations, including model-corrected p-distances as well as those based on allele frequencies.
autoStreamTree includes integrated functions for parsing an input vector shapefile of streams for calculation of pairwise stream distances b/n sites, as well as the ordinary or weighted least-squares fitting of reach-wise genetic distances according to the "stream tree" model.

□ Hierarchical Interleaved Bloom Filter: enabling ultrafast, approximate sequence queries
>> https://genomebiology.biomedcentral.com/articles/10.1186/s13059-023-02971-4
the Hierarchical Interleaved Bloom Filter (HIBF) that overcomes major limitations of the IBF data structure. The HIBF successfully decouples the user input from the internal representation, enabling it to handle unbalanced size distributions and millions of samples.
The HIBF structure has enormous potential. It can be used on its own, like in the tool Raptor, or can serve as a prefilter to distribute more advanced analyses such as read mapping. Querying ten million reads could be done by querying 11 HIBFs on different machines in parallel.

□ A survey of mapping algorithms in the long-reads era
>> https://genomebiology.biomedcentral.com/articles/10.1186/s13059-023-02972-3
Adapting and tailoring long-read aligners to such applications will significantly improve analysis over the limited possibilities existing with short reads. Moreover, using pangenomes represented as graphs made from a set of reference genomes is becoming more prevalent.
As a result, long-read mapping to these structures is a novel and active field for genomic reads but should soon expand to other applications such as transcriptomics.
Notably, pangenome graphs vary in definition and structure (overlap graphs, de Bruijn graphs, graphs of minimizers) and therefore expect a diversified algorithmic response to mapping sequences on these graphs.

□ SVcnn: an accurate deep learning-based method for detecting structural variation based on long-read data
>> https://bmcbioinformatics.biomedcentral.com/articles/10.1186/s12859-023-05324-x
SVcnn accurately detects DELs, INSs, DUPs, and INVs. SVcnn is a convolutional neural network (CNN) based method. It uses hierarchical clustering to identify if a region contains multi-allelic SVs. Moreover, SVcnn utilizes the LetNet model to distinguish whether an SV is a true SV or not.
The input of SVcnn consists of (i) a sorted long read bam file and (ii) a reference file. SVcnn mainly consists of three main steps: (1) Detecting candidate SVs, (2) Converting to image and building model, (3) Filtering and outputting SVs.

□ Epiphany: predicting Hi-C contact maps from 1D epigenomic signals
>> https://genomebiology.biomedcentral.com/articles/10.1186/s13059-023-02934-9
Epiphany, a neural network to predict cell-type-specific Hi-C contact maps from widely available epigenomic tracks. Epiphany uses Bi-LSTM layers to capture long-range dependencies and optionally a generative adversarial network architecture to encourage contact map realism.
Epiphany can be trained with MSE alone or with a combination of MSE and GAN loss. In the latter case, the full model consists of two parts: a generator to extract information and make predictions, and a discriminator to introduce adversarial loss into the training process.

□ networkGWAS: A network-based approach to discover genetic associations
>> https://academic.oup.com/bioinformatics/advance-article/doi/10.1093/bioinformatics/btad370/7191773
networkGWAS, a statistically sound approach to network-based GWAS using mixed models and neighborhood aggregation. It allows for population structure correction and for well-calibrated p-values, which are obtained through circular and degree-preserving network permutations.
networkGWAS successfully detects known associations on diverse synthetic phenotypes. It employs a FaST-LMM-Set like model to estimate the statistical associations with the phenotype of choice. networkGWAS presents higher recall in comparison to dmGWAS per each precision value.

□ NoVaTeST: Identifying Genes with Location Dependent Noise Variance in Spatial Transcriptomics Data
>> https://academic.oup.com/bioinformatics/advance-article/doi/10.1093/bioinformatics/btad372/7191774
NoVaTeST pipeline that offers a more general spatial gene expression modeling in ST data using the heteroscedastic Gaussian process. The pipeline uses Wilcoxon signed rank test and FDR correction to identify genes with location-dependent noise variance.

□ TreeTerminus - Creating transcript trees using inferential replicate counts
>> https://www.sciencedirect.com/science/article/pii/S2589004223010386
TreeTerminus, a data-driven approach for grouping transcripts into a tree structure where leaves represent individual transcripts and internal nodes represent an aggregation of a transcript set.
TreeTerminus constructs trees such that, on average, the inferential uncertainty decreases as ascending the tree topology. It provides the flexibility to analyze data at nodes that are at different levels of resolution and can be tuned depending on the analysis of interest.


□ hifiasm ultra-long (UL): Scalable telomere-to-telomere assembly for diploid and polyploid genomes with double graph
>> https://arxiv.org/abs/2306.03399
hifiasm (UL) provides an ultra-fast and robust solution for telomere-to-telomere genome assemblies in a population-scale. hifiasm (UL) will facilitate a more comprehensive understanding of complex genomic regions such as centromeres and highly repetitive segmental duplications.
Hifiasm (UL) constructs an integer graph by utilizing ultra-long integer sequences and their overlaps. hifiasm (UL) employs highly aggressive graph cleaning strategies to eliminate ambiguous edges associated with each node.
hifiasm (UL) produces its sequence by concatenating the subsequences of nodes. Each resulting contig is an integer sequence that is significantly longer than any individual ultra-long read. These integer contigs represent the paths that can untangle intricate structures.

□ hifieval: Evaluation of haplotype-aware long-read error correction
>> https://www.biorxiv.org/content/10.1101/2023.06.05.543788v1
hifieval evaluates phased assemblies and can distinguish under-corrections and over-corrections. It is perhaps the first user-facing EC evaluation tool that can be easily deployed to users' own datasets.
hifieval calculates three metrics: correct corrections (CC), errors that are in raw reads but not in corrected reads; under-corrections (UC), errors present in both raw and corrected reads; and over-corrections (OC), new errors found in corrected reads but not in raw reads.

□ Ewald-based Long-Range Message Passing for Molecular Graphs
>> https://arxiv.org/abs/2303.04791
Ewald message passing (MP) is a general framework that complements existing GNN layers in analogy to how the frequency-truncated long-range part complements the distance-truncated short-range part in Ewald summation.
Ewald message passing is architecture-agnostic and computationally efficient, which we demonstrate by implementing and testing it as a modification on top of existing GNN models. Ewald MP is more suitable for large or periodic structures containing a diverse set of atoms.

□ Pathformer: biological pathway informed Transformer model integrating multi-modal data of cancer
>> https://www.biorxiv.org/content/10.1101/2023.05.23.541554v1
Pathformer, a biological pathway informed deep learning model based on Transformer with bias to integrate multi-modal data. Pathformer leverages criss-cross attention mechanism to capture crosstalk between different biological pathways and between different modalities.
Pathformer utilizes a sparse neural network based on pathway knowledge to transform gene embeddings into pathway embeddings. Pathway crosstalk matrix is used to guide the direction of information flow, and updated according to encoded pathway embedding in each Transformer block.

□ DiffPack: A Torsional Diffusion Model for Autoregressive Protein Side-Chain Packing
>> https://arxiv.org/abs/2306.01794
DiffPack, an autoregressive torsional diffusion model that learns the joint distribution of side-chain torsional angles, the only degrees of freedom in side-chain packing, by diffusing and denoising on the torsional space.
DiffPack use s an SE(3)- invariant network to learn the gradient field for the joint distribution of torsional angles. This result in a much smaller conformation space of side-chain, thereby capturing the intricate energy landscape of protein side chains.

□ scGHOST: Identifying single-cell 3D genome subcompartments
>> https://www.biorxiv.org/content/10.1101/2023.05.24.542032v1
scGHOST is a single-cell compartmentalization framework and views scHi-C contact maps as graphs, where genomic loci are vertices in the graph and are connected through edge weights defined by Hi-C contact frequencies among loci.
scGHOST employs a unique random sampling procedure that filters noise in imputed scHi-C data, represents each genomic locus as a continuous-valued vector, and uses graph embedding neural networks to discretize single-cell genomes and identify 3D genome subcompartments.

□ Construction and representation of human pangenome graphs
>> https://www.biorxiv.org/content/10.1101/2023.06.02.542089v1
They collect all publicly available high-quality human haplotypes and constructed the largest human pangenome graphs to date, incorporating 52 individuals in addition to two synthetic references (CHM13 and GRCh38).
Building variation graphs and de Bruijn graphs of this collection using five of the state-of-the-art tools: Bifrost, mdbg, Minigraph, Minigraph-Cactus and pggb.
Counter-intuitively, a pangenome graph construction tool may in some cases generate different outputs when executed multiple times with the same haplotypes as input.
This unstability could be due to a permutation in the order of the sequences given as input, or non-determinism in the construction algorithm.
□ AGILE Platform: A Deep Learning-Powered Approach to Accelerate LNP Development for mRNA Delivery
>> https://www.biorxiv.org/content/10.1101/2023.06.01.543345v1
The AI-Guided Ionizable Lipid Engineering (AGILE) platform, a synergistic combination of deep learning and combinatorial chemistry. AGILE streamlines the iterative development of ionizable lipids, crucial components for LNP-mediated mRNA delivery.
AGILE utilizes vast amounts of unlabeled data, employing a self-supervised approach to learn differentiable lipid representations. AGILE can identify promising lipids for high mRNA transfection potency in specific cells from a significantly larger combinatorial library.

□ Dictionary learning for integrative, multimodal and scalable single-cell analysis
>> https://www.nature.com/articles/s41587-023-01767-y
‘bridge integration’, which integrates single-cell datasets measuring different modalities by leveraging a separate dataset where both modalities are simultaneously measured as a molecular ‘bridge’.
‘atomic sketch integration’, which combines dictionary learning and dataset sketching to improve the computational efficiency of large-scale single-cell analysis and enables rapid integration of dozens of datasets spanning millions of cells.
Motivated by a similar problem addressed by Laplacian Eigenmaps, they compute an eigen decomposition of the graph Laplacian for the multiomic dataset to reduce the dimensionality from the number of atoms to the number of selected eigenvectors.

□ minimap2-fpga: Integrating hardware-accelerated chaining for efficient end-to-end long-read sequence mapping
>> https://www.biorxiv.org/content/10.1101/2023.05.30.542681v1
minimap2-fpga, a Field Programmable Gate Array (FPGA) based hardware-accelerated version of minimap2 that is end-to-end integrated. minimap2-fpga speeds up the mapping process by integrating an FPGA kernel optimised for chaining.
minimap2-fpga is up to 79% and 53% faster than minimap2 for ∼ 30× ONT and ∼ 50× PacBio datasets, when mapping without base-level alignment. When mapping w/ base-level alignment, minimap2-fpga is up to 62% and 10% faster than minimap2 for ∼ 30× ONT and ∼ 50× PacBio datasets.
The accuracy is near-identical to that of original minimap2 for both ONT and PacBio data, when mapping both with and without base-level alignment. minimap2-fpga is supported on Intel FPGA-based systems and Xilinx FPGA-based systems.

□ StabMap: Stabilized mosaic single-cell data integration using unshared features
>> https://www.nature.com/articles/s41587-023-01766-z
StabMap, a mosaic data integration technique that stabilizes mapping of single-cell data by exploiting the non-overlapping features. StabMap accurately embeds single-cell data from multiple technology sources into the same low-dimensional coordinate space.
StabMap projects all cells onto supervised or unsupervised reference coordinates using all available features regardless of overlap with other datasets, instead relying on traversal along the mosaic data topology.

□ The Damage to Lunar Orbiting Spacecraft Caused by the Ejecta of Lunar Landers
>> https://arxiv.org/abs/2305.12234
The results for ~40 t landers show that the Lunar Orbital Gateway will be impacted by 1000s to 10,000s of particles per square meter but the particle sizes are very small and the impact velocity is low so the damage will be slight.
A spacecraft in Low Lunar Orbit that happens to pass through the ejecta sheet will sustain extensive damage w/ hundreds of millions of impacts per SQM: they are in the hypervelocity regime, and exposed glass on the spacecraft will sustain spallation over 4% of its surface.

□ Anansi: Knowledge-based Integration of Multi-Omic Datasets: Annotation-based Analysis of Specific Interactions
>> https://arxiv.org/abs/2305.10832
Anansi (Annotation-based Analysis of Specific Interactions) relies on the structure provided from knowledge databases. Typically, these are databases that contain knowledge on features and how they interact, for example in the form of a molecular interaction network.
Anansi takes a knowledge-based approach where external databases like KEGG are used to constrain the all-vs-all association hypothesis space, only considering pairwise associations that are a priori known to occur.

□ GNN-C2L: Spatio-relational inductive biases in spatial cell-type deconvolution
>> https://www.biorxiv.org/content/10.1101/2023.05.19.541474v1
GNN-C2L propagates learnable messages on the proximity graph of spot transcripts, effectively leveraging the spatial relationships between spots and exploiting the co-location of cell-types.
GNN-C2L achieves increased deconvolution performance over spatial-agnostic variants. GNN-C2L leverages proximal inductive biases to facilitate enhanced reconstruction of tissue architectures.

□ Tara Oceans + anvi’o: The story behind Mirusviruses
>> https://anvio.org/blog/mirus-discovery/
An unusual phylogenetic signal that guided the recovery of large eukaryotic virus genomes forming their very own phylum at the cross-road between two realms.

□ CADA-BioRE: A Co-adaptive Duality-aware Framework for Biomedical Relation Extraction
>> https://academic.oup.com/bioinformatics/advance-article/doi/10.1093/bioinformatics/btad301/7176367
CADA-BioRE, is designed as a bidirectional extraction structure that fully takes interdependence into account in the duality- aware extraction process of subject-object entity pair and relation.
CADA leverages a duality module for inverse extracting triplets and a matching module to correct errors. CADA-BioRE achieves outstanding performance gains even in complex scenarios involving various overlapping patterns, multiple triplets, and cross-sentence triplets.

□ Genekitr: Empowering biologists to decode omics data
>> https://bmcbioinformatics.biomedcentral.com/articles/10.1186/s12859-023-05342-9
Genekitr comprises four modules: gene information retrieval, ID (identifier) conversion, enrichment analysis and publication-ready plotting. The ID conversion module assists in ID-mapping of genes, probes, proteins, and aliases.
Genekitr integrates various functionalities into a single web server: GeneInfo module for batch query gene information, IDConvert and ProbeConvert for gene and probe identifier conversion, GeneEnrich for gene enrichment analysis and Plot module for publication-ready plotting.

□ BioModelsML: Building a FAIR and reproducible collection of machine learning models in life sciences and medicine for easy reuse
>> https://www.biorxiv.org/content/10.1101/2023.05.22.540599v1
The formalisation and pilot implementation of community protocol to enable FAIReR (Findable, Accessible, Interoperable, Reusable, and Reproducible) sharing of ML models.
The trained model should be made available in either native format or ONNX (Open Neural Network Exchange) format when possible. ONNX is a widely used open-source format designed to foster interoperability between different machine learning frameworks.

□ itol.toolkit accelerates working with iTOL (Interactive Tree Of Life) by an automated generation of annotation files
>> https://academic.oup.com/bioinformatics/advance-article/doi/10.1093/bioinformatics/btad339/7177989
This R package also provides an all-in-one data structure to store data and themes, accelerating the step from metadata to annotation files of iTOL visualizations through automatic workflows.

□ kmindex and ORA: indexing and real-time user-friendly queries in terabytes-sized complex genomic datasets
>> https://www.biorxiv.org/content/10.1101/2023.05.31.543043v1
kmindex offers the possibility to index thousands of highly complex metagenomes into an index that answers sequences queries in the tenth of a second. Using kmindex, the resulting indexes can be registered into a single meta-index allowing users to easily query multiple indexes.
Ocean Read Atlas (ORA) allows query one or several sequences across all of the Tara Oceans metagenomic raw datasets. ORA enables the visualization of the results on a geographic map. ORA provides new perspectives on the deep exploitation of Tara oceans resources.

□ Mutate and Observe: Utilizing Deep Neural Networks to Investigate the Impact of Mutations on Translation Initiation
>> https://academic.oup.com/bioinformatics/advance-article/doi/10.1093/bioinformatics/btad338/7177993
Getting DNNs to describe the biological relevance of what was learned from the data and extracting novel biological knowledge from DNNs are two tasks that are not easy to accomplish. In this research effort.
The usefulness of in silico mutations, in combination with meticulous experimental routines, to achieve a certain degree of biological relevance and to obtain novel insights into translation.

□ Pygenomics: manipulating genomic intervals and data files in Python
>> https://academic.oup.com/bioinformatics/advance-article/doi/10.1093/bioinformatics/btad346/7179791
Unlike general numeric intervals, the genomic intervals are associated with assembled genome sequences (chromosomes, scaffolds, or contigs) and the interval start and end positions are specified by non-negative integers bounded by sizes of the assembled genome sequences.
pygenomics, a Python package for working with genomic intervals and bioinfor- matic data files. The package implements interval operations, provides both API and CLI, and supports reading and writing data in widely used bioinformatic formats, including BAM, BED, GFF3 and VCF.

□ mutscan: a flexible R package for efficient end-to-end analysis of multiplexed assays of variant effect data
>> https://genomebiology.biomedcentral.com/articles/10.1186/s13059-023-02967-0
mutscan, a novel R package that provides a unified, flexible interface to the analysis of MAVE experiments, covering the entire workflow from FASTQ files to count tables and statistical analysis and visualization.
mutscan is directly applicable also to other types of data aimed at identifying and tabulating substitution variants compared to a provided reference sequence, or tabulating unique sequences directly, potentially after collapsing variants within a certain distance.

□ IsoTools: a flexible workflow for long-read transcriptome sequencing analysis
>> https://academic.oup.com/bioinformatics/advance-article/doi/10.1093/bioinformatics/btad364/7189737
IsoTools integrates a graph-based method for identifying alternative splicing events and a statistical approach based on the beta-binomial distribution for detecting differential events. IsoTools uses a novel model based approach to estimating the required depth of sequencing.
The model is based on the Cumulative Distribution Function of a negative binomial distribution. To reconstruct the transcriptome from aligned reads, IsoTools groups reads w/ the same intron chain into transcripts/groups transcripts sharing at least one splice junction into genes.
IsoTools uses the reference positions. To determine the positions of transcription start sites (TSSs) and polyadenylation sites (PASs), IsoTools employs a gene-wise peak calling approach to identify the most prominent start and end positions of reads.

□ WAGS: User-friendly, rapid, containerized pipelines for processing, variant discovery, and annotation of short read whole genome sequencing data
>> https://academic.oup.com/g3journal/advance-article/doi/10.1093/g3journal/jkad117/7181376
WAGS is an open-source set of user-friendly, containerized pipelines designed to simplify the process of identifying germline short (SNP and indel) and structural variants geared toward the veterinary community but adaptable to any species with a suitable reference genome.
WAGS consists of three pipelines for (1) processing raw short-read FAST files into GVCFs: OneWAG, (2) joint genotyping and annotating variants: ManyWAGS, and (3) the identification of private variants in a single sample: OnlyWAG.

□ MR-Horse: A Bayesian approach to Mendelian randomization using summary statistics in the univariable and multivariable settings with correlated pleiotropy
>> https://www.biorxiv.org/content/10.1101/2023.05.30.542988v1
MR-Horse had comparable power and bias to CAUSE, with substantially lower type I error rates. It again had slightly higher coverage and lower type I error rates compared with MR-cML-DP, and outperformed all other methods across each metric.
MVMR-Horse outperformed IVW, MVMR-Median and GRAPPLE in terms of bias, precision and type I error rates in all scenarios. MVMR-Horse retained type I error rates below the nominal level in all scenarios, with the trade-off of lower power compared with MVMR-cML-DP.

□ Jupyter AI
>> https://jupyter-ai.readthedocs.io/en/latest/index.html
Jupyter AI provides a user-friendly and powerful way to explore generative AI models in notebooks and improve your productivity in JupyterLab and the Jupyter Notebook

□ MolXPT: Wrapping Molecules with Text for Generative Pre-training
>> https://arxiv.org/abs/2305.10688
MolXPT, a unified language model of text and molecules pre-trained on SMILES (a sequence representation of molecules) wrapped by text. MolXPT can be finetuned for various text and molecular downstream tasks, like molecular property prediction and molecule-text translation.
MolXPT outperforms strong baselines of molecular property prediction on MoleculeNet, performs comparably to the best model in text-molecule translation while using less than half of its parameters, and enables zero-shot molecular generation without finetuning.

□ MTM: a multi-task learning framework to predict individualized tissue gene expression profiles
>> https://academic.oup.com/bioinformatics/advance-article/doi/10.1093/bioinformatics/btad363/7190366
Multi-tissue Transcriptome Mapping (MTM), a deep learning-based multi-task learning framework to predict individualized tissue gene expression profiles using any available tissue from a specific person.
By jointly leveraging individualized cross-tissue information from multi-tissue reference samples through multi-task learning, MTM achieves superior sample-level and gene-level accuracy, and larger proportions of predictable genes than existing methods on unseen individuals.

□ SMEAR: Soft Merging of Experts with Adaptive Routing
>> https://arxiv.org/abs/2306.03745
SMEAR avoids discrete routing by using a single "merged" expert constructed via a weighted average of all of the experts' parameters. SMEAR provides an effective alternative for modular models that use adaptive routing among expert subnetworks.
All components of SMEAR are fully differentiable enables standard gradient-based training. Empirically, SMEAR significantly attains a favorable performance/cost tradeoff to discrete routing solutions found via gradient estimation.

□ Orthogonal Statistical Learning
>> https://arxiv.org/abs/1901.09036
A meta-algorithm that takes as input arbitrary estimation algorithms for the target/nuisance parameter. If the population risk satisfies a Neyman orthogonality, the impact of the nuisance estimation error on the excess risk bound achieved by the meta-algorithm is of second order.
The theorem is agnostic to the particular algorithms used for the target/nuisance and only makes an assumption on their individual performance. It enables the use of a plethora of existing results from machine learning to give new guarantees for learning w/ a nuisance component.
This method can accommodate settings in which the target parameter belongs to a complex nonparametric class. It provide conditions on the metric entropy of the nuisance and target classes such that oracle rates of the same order as if we knew the nuisance parameter are achieved.

□ Biotite: new tools for a versatile Python bioinformatics library
>> https://bmcbioinformatics.biomedcentral.com/articles/10.1186/s12859-023-05345-6
flexibility can be harnessed to tackle a wide range of problems, without the need to write ‘glue’ code for communication between different programs. For most tasks the implementation in Biotite performs similar or is even faster than dedicated software.
Biotite is able to create sequence profiles from multiple sequence alignments consisting of nucleotide, protein or custom sequences. The usefulness of profiles lies in their better representation of information than a consensus sequence or a multiple sequence alignment.

□ DesiRNA: structure-based design of RNA sequences with a Monte Carlo approach
>> https://www.biorxiv.org/content/10.1101/2023.06.04.543636v1
DesiRNA, a versatile Python-based software tool for RNA sequence design. This program considers a comprehensive array of constraints, ranging from secondary structures (including pseudoknots) and GC content, to the distribution of dinucleotides emulating natural RNAs.
Additionally, it factors in the presence or absence of specific sequence motifs and prevents or promotes oligomerization, thereby ensuring a robust and flexible design process.
DesiRNA utilizes the Monte Carlo algorithm for the selection and acceptance of mutation sites. In tests on the EteRNA benchmark, DesiRNA displayed high accuracy and computational efficiency, outperforming most existing RNA design programs.

□ DiffSegR: An RNA-Seq data driven method for differential expression analysis using changepoint detection
>> https://www.biorxiv.org/content/10.1101/2023.06.05.543691v1
DiffSegR, an R package that uses a new strategy for delineating the boundaries of DERs. It segments the per-base log2 fold change using FPOP, a method designed to identify changepoints in the mean of a Gaussian signal.

□ The NanoFlow Repository
>> https://academic.oup.com/bioinformatics/advance-article/doi/10.1093/bioinformatics/btad368/7191772
The NanoFlow Repository to provide the first implementation of the MIFlowCyt-EV framework. It enables sharing of EP-FC data and standards-compliant metadata about experimental design, samples, instrument configuration, and analysis parameters.

GitHub Copilot Chatで実際にコーディングしてみた。
これはLaplacian Score Vectorに基づきgene sortingを行うようプロンプトに記述、その後にSubgraphの出力を指示したもの。更にCopilot側からCytoscapeを用いたヴィジュアリゼーションを提案、クリックすると左パネルに一連となるコードが生成された

『Soaring: Fantastic Flight (ソアリン)』
>> https://www.tokyodisneyresort.jp/en/tds/attraction/detail/219/
Tokyo Disney SEAの最新にして最大の人気を誇るアトラクションであり
「全人類が今すぐ味わうべき映像体験」
IMAX (OmniMax)で全視界を覆う世界各地のパノラマ映像とライド型アクションの融合で未知の冒険へ!
ゆーてでしょwとタカを括ってたら冒頭のマッターホルンで死にかけた









『Indiana Jones Adventure: Temple of the Crystal Skull』
>> https://www.tokyodisneyresort.jp/tds/attraction/detail/222/
新作映画が公開間近ということで普段より混んでた感。ライドまで広大に続く遺跡探査坑が雰囲気満点。








C-3PO号!( 『レイダース』本編では”OB-CPO”)