

□ Transcriptional landscapes of de novo root regeneration from detached Arabidopsis leaves revealed by time-lapse and single-cell RNA sequencing analyses
>> https://www.cell.com/plant-communications/fulltext/S2590-3462(22)00053-0
Time-lapse RNA sequencing (RNA-seq) of the entire leaf within 12 h of leaf detachment revealed rapid activation of jasmonate, ethylene, and reactive oxygen species (ROS) pathways in response to wounding.
Time-lapse RNA-seq within 5 d of leaf detachment revealed the activation of genes involved in organogenesis, wound-induced regeneration, and resource allocation in the wounded region of detached leaves during adventitious rooting.

□ WarpSTR: Determining tandem repeat lengths using raw nanopore signals
>> https://academic.oup.com/bioinformatics/advance-article/doi/10.1093/bioinformatics/btad388/7199589
WarpSTR, a novel method for characterizing both simple and complex tandem repeats directly from raw nanopore signals using a finite-state automaton and a search algorithm analogous to dynamic time warping.
WarpSTR attenuates the signal normalization problem using a novel signal polishing phase. WarpSTR uses Bayesian Gaussian mixture models to summarize the information from multiple overlapping reads and to derive the final genotypes.

□ scFoundation: Large Scale Foundation Model on Single-cell Transcriptomics
>> https://www.biorxiv.org/content/10.1101/2023.05.29.542705v2
scFoundation, a large-scale model that models 19,264 genes with 100 million parameters, pre-trained on over 50 million scRNA-seq data. It uses xTrimoGene, a scalable transformer-based model that includes an embedding module and an asymmetric encoder-decoder structure.
scFoundation converts continuous gene expression scalars into learnable high-dimensional vectors. A read-depth-aware pre-training task enables scFoundation not only to model the gene co-expression patterns within a cell but also to link the cells with different read depths.

□ Exceiver: A single-cell gene expression language model
>> https://arxiv.org/abs/2210.14330
Exceiver is a single-cell gene expression language model with an attention-based transformer backbone that encodes long-context transcriptomic profiles. Exceiver utilizes discrete noise masking and enables self-supervised learning on unlabeled, continuously-valued datasets.
Exceiver retains the core Perceiver IO architectural components. Exceiver provides utility in transferring systems knowledge to downstream tasks, from the interrogation of molecular functions to the prediction of comprehensive phenotypes.

□ FAME: Efficiently Quantifying DNA methylation for bulk- and single-cell bisulfite data
>> https://academic.oup.com/bioinformatics/advance-article/doi/10.1093/bioinformatics/btad386/7199582
FAME (Fast and Accurate MEthylation) Aligner enables ultra-fast / parallel querying of reads w/o I/O overhead. Carries out alignment / methylation calling for CpGs of Whole Genome Bisulfite Sequencing (WGBS) reads in one go w/o the need of intermediate alignment or buffer files.
The FAME algorithm is working on the full alphabet (A,C,G,T), resolving the asymmetric mapping problem* correctly. It exploits spaced k-mer counting within short segments of the genome to quickly reduce the genomic search space.
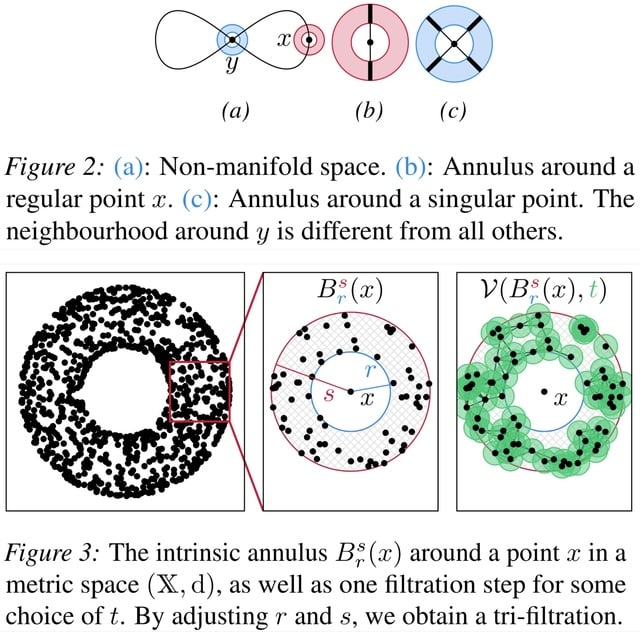
□ TARDIS: Topological Singularity Detection at Multiple Scales
>> https://arxiv.org/abs/2210.00069
TARDIS (Topological Algorithm for Robust DIscovery of Singularities) consists of two parts: a method to calculate a local intrinsic dimension of the data, and the 'manifoldness' via Euclidicity, a measure for assessing the multi-scale deviation from a Euclidean space.
TARDIS analyses data on multiple scales. The main idea involves constructing a collection of local (punctured) neighbourhoods for varying locality scales, and calculating their topological features.
Euclidicity can detect singular regions in data sets with known singularities. It enables the detection singularities in a large range of input data sets. For the subsequent description of TARDIS, we only assume that data can be represented as a finite metric space.

□ CellDancer: A relay velocity model infers cell-dependent RNA velocity
>> https://www.nature.com/articles/s41587-023-01728-5
cellDancer, a scalable deep neural network that locally infers velocity for each cell from its neighbors and then relays a series of local velocities to provide single-cell resolution inference of velocity kinetics.
cellDancer calculates a minimized loss function to train the DNN based on the similarity b/n the predicted future spliced / unspliced mRNA of each cell and the observation of its neighbor cells. Based on the defined nearest neighbors, CellDancer can predict velocity vector flow.

□ Matchtigs: minimum plain text representation of k-mer sets
>> https://genomebiology.biomedcentral.com/articles/10.1186/s13059-023-02968-z
Matchtigs, a polynomial algorithm computing a minimum plain-text representation of k-mer sets, as well as an efficient near-minimum greedy heuristic. Matchtigs uses a first algorithm to find a spectrum preserving string set (SPSS).
A minimum SPSS with repeated k-mers is polynomially solvable, based on a many-to-many min-cost path query and a min-cost perfect matching approach. A faster and more memory-efficient greedy heuristic computes a small SPSS that skips the optimal matching step.

□ scHiMe: predicting single-cell DNA methylation levels based on single-cell Hi-C data
>> https://academic.oup.com/bib/advance-article/doi/10.1093/bib/bbad223/7193585
scHiMe is a computational tool for predicting the base-pair-specific methylation levels in the promoter regions genome-wide based on the single-cell Hi-C data and DNA nucleotide sequences using the Graph Transformer algorithm.
scHiMe uses the collective influence (CI) algorithm to the promoter–promoter spatial interaction networks. The genomic regions that have single-cell Hi-C contacts or are spatially proximate in the 3D space may share similar single-cell methylation levels.

□ A Unified Model and Dimension for Interactive Estimation
>> https://arxiv.org/abs/2306.06184
Dissimilarity dimension, a combinatorial complexity measure, which largely captures learnability in interactive estimation. Intuitively, this measure corresponds to the length of the longest sequence of alternatives in which each one has a similar suboptimal value of similarity to all its predecessors.
Both regret bounds and PAC generalization bounds that are all polynomial in the dissimilarity dimension. This model subsumes the statistical query (SQ) model for designing noise-tolerant learning algorithms. In the SQ model, the learner can sequentially ask certain queries of an oracle.
The dissimilarity dimension is upper-bounded by the eluder dimension, and that there can in fact be a large gap between the two. This sometimes leads to an improved analysis when relying on the proposed dissimilarity measure rather than the eluder dimension.

□ Mistle: bringing spectral library predictions to metaproteomics with an efficient search index
>> https://academic.oup.com/bioinformatics/advance-article/doi/10.1093/bioinformatics/btad376/7192987
Mistle is a fast spectral search engine. It uses a fragment-indexing technique and SIMD intrinsics to match experimental MS2 spectra to large spectral libraries at a high performance.
Mistle emulates a classic protein sequence database search with protein digestion but builds a searchable index from spectral predictions as an in-between step. At its core, Mistle provides high-performance spectral matching based on the spectral dot product of binned peaks.

□ Cellular gradient flow structure linking single-cell-level rules and population-level dynamics
>> https://link.aps.org/doi/10.1103/PhysRevResearch.5.L022052
The single-cell rules and the population-level goal are naturally connected via a gradient flow structure of heterogeneous cellular populations and that single-cell rules, such as unidirectional type switching and hierarchical order in types, emerge from this structure.
Finally, it should be noted that a given population dynamics may not always fall into the class of gradient flow in the strict sense. Some modifications of the T-cell model can violate the conditions to be a gradient flow.
Nevertheless, the gradient-flow like behaviors can still be preserved if the modification is moderate, and the utility monotonically increases in time. Thus, the theory can be used to search for such behaviors.
Moreover, we can further extend the notion of gradient flow to accommodate oscillatory components, e.g. cell cycle, and others. It expands the applicability of this approach to a wide range of multicellular phenomena and will be pursued.

□ Finding Motifs Using DNA Images Derived From Sparse Representations
>> https://academic.oup.com/bioinformatics/advance-article/doi/10.1093/bioinformatics/btad378/7192989
A principled representation learning based on a hierarchical sparse representation. By approximating DNA strings as a sum of linear convolutions, the non-zero components in the sparse code act as indicators, indicating where filters should be used to represent DNA substrings.
The combined sparse code provides a high-level view on the spatial arrangements of the filters, which we build another sparse representation upon. It enables us to identify the conserved patterns in the dataset, akin to enumerating k-mers at the nucleotide level.

□ Bambu: Context-aware transcript quantification from long-read RNA-seq data
>> https://www.nature.com/articles/s41592-023-01908-w
Bambu estimates the novel discovery rate, which replaces arbitrary per-sample thresholds with a single, interpretable, precision-calibrated parameter. Bambu retains the full-length and unique read counts, enabling accurate quantification in presence of inactive isoforms.
Bambu performs error correction on the splice junctions of the aligned reads. It assigns read classes to transcripts in the extended annotation, categorizing them as having full-length or partial overlaps, and performs probabilistic transcript quantification.
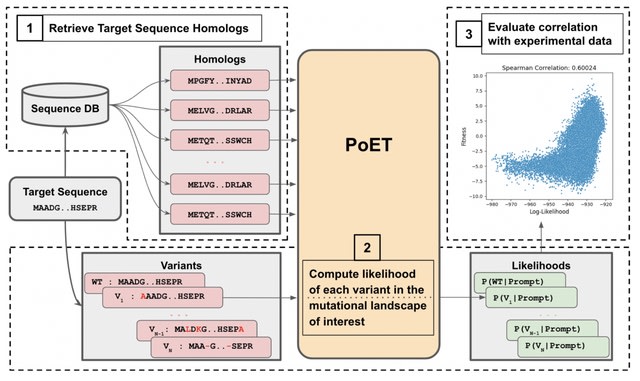
□ PoET: A generative model of protein families as sequences-of-sequences
>> https://arxiv.org/abs/2306.06156
Protein Evolutionary Transformer (PoET), an autoregressive generative model of whole protein families that learns to generate sets of related proteins as sequences-of-sequences across tens of millions of natural protein sequence clusters.
PoET can be used as a retrieval-augmented language model to generate and score arbitrary modifications conditioned on any protein family of interest. PoET improves variant effect prediction across proteins of all multiple sequence alignment depths.

□ TopicVelo: Dissection and Integration of Bursty Transcriptional Dynamics for Complex Systems
>> https://www.biorxiv.org/content/10.1101/2023.06.13.544828v1
TopicVelo disentangles potentially simultaneous processes using a probabilistic topic model, also known as a grade-of-membership model, which is a highly interpretable, Bayesian non-negative matrix factorization.
TopicVelo obtains a global transition matrix by leveraging cell topic weights to integrate process-specific signals. TopiVelo recovers complex transitions and terminal states, while the novel use of first-passage time analysis provides insights into transient transitions.

□ JAMIE: Joint variational autoencoders for multimodal imputation and embedding
>> https://www.nature.com/articles/s42256-023-00663-z
JAMIE uses VAE with a novel latent space aggregation technique in order to generate similar latent spaces for each modality. JAMIE preserves the branching structure of the manifold while aligning the cells of the same type in either modality and maintains cell type separation.
Intuitively, the continuous latent space allows for easy sampling and interpolation. Additionally, the proposed aggregation method relies on the interpolation of latent representations and is improved after switching to a continuous latent space.

□ DivBrowse: interactive visualization and exploratory data analysis of variant call matrices
>> https://academic.oup.com/gigascience/article/doi/10.1093/gigascience/giad025/7135628
DivBrowse combines the approach of genome browsers with the capability to visualize and interactively analyze thousands to millions of genomic variants for thousands of genotypes in the style of an exploratory data analysis.
DivBrowse calculates variant statistics such as minor allele frequencies, proportion of heterozygous calls / missing variant calls for each visualized genomic window. Variant effect predictions according to SnpEff can be displayed, provided they are present in the underlying VCF.

□ An introduction to the analysis of gradients systems
>> https://arxiv.org/abs/2306.05026
The aim of these notes to give an introductory overview on the analytical approaches for gradient-flow equations in Hilbert spaces, Banach spaces, and metric spaces and to show that on the first entry level these theories have a lot in common.
EDP-Convergence for gradient systems has similar properties as Γ-convergence. The notion is independent of the concept of "solution", which in the case of classical functionals means minimizer and in the case of gradient systems means solutions of the gradient-flow equation.

□ miniBUSCO: a faster and more accurate reimplementation of BUSCO
>> https://www.biorxiv.org/content/10.1101/2023.06.03.543588v1
miniBUSCO utilizes the protein-to-genome aligner miniprot and the datasets of conserved orthologous genes from BUSCO. The evaluation of the real human assembly indicates that miniBUSCO achieves a 14-fold speedup over BUSCO.
Frameshift refers to the insertion or deletion of several base pairs that are not a multiple of three, disrupting the triplet reading frame of a DNA sequence. Miniprot can align through frameshifts in the genome sequence and identify them.
A complete gene is considered to have a single-copy in the assembly if it only has one alignment, or duplicated if it has multiple alignments. MiniBUSCO reports the proportion of genes falling into each of the four categories as the assessment of assembly completeness.

□ SEMtree: tree-based structure learning methods with Structural Equation Models
>> https://academic.oup.com/bioinformatics/advance-article/doi/10.1093/bioinformatics/btad377/7192988
Structural Equation Models (SEM) Tree is able to capture biologically relevant sub-networks with simple visualization of directed paths. SEMtree() recovers the tree-based structure starting from the interactome and gene expression information while offering good enrichment metrics, perturbation extraction and classifier performance.

□ DeepITEH: A deep learning framework for identifying tissue-specific eRNAs from the human genome
>> https://academic.oup.com/bioinformatics/advance-article/doi/10.1093/bioinformatics/btad375/7192986
DeepITEH, a deep learning framework that leverages RNA-seq data and histone modification data from multiple samples of the same tissue to enhance the accuracy of identifying eRNAs.

□ Hidden protein-altering variants influence diverse human phenotypes
>> https://www.biorxiv.org/content/10.1101/2023.06.07.544066v1
The additional information provided by SNP haplotypes could enable analyses of abundant exome sequencing data to detect even small copy-number-altering SVs within individual protein-coding genes-including genes within multi-copy and segmental duplication regions.

□ zol & fai: large-scale targeted detection and evolutionary investigation of gene clusters
>> https://www.biorxiv.org/content/10.1101/2023.06.07.544063v1
zol (zoom-on-locus) and fai (find-additional-instances), which are designed for the identification and in-depth evolutionary genomics investigations of a wide array of gene cluster types.

□ PlotS: web-based application for data visualization and analysis
>> https://www.biorxiv.org/content/10.1101/2023.06.09.544161v1
PlotS is a visualization centric web-based application that allows the integration of statistical analysis into a single workflow. The current version has eight types of graphs and four statistical methods (T-test, ANOVA, Wilcoxon test and Krushkal-Wallis test).

□ Detecting haplotype-specific transcript variation in long reads with FLAIR2
>> https://www.biorxiv.org/content/10.1101/2023.06.09.544396v1
FLAIR2 is a variant-aware isoform detection pipeline. The modified FLAIR workflow (FLAIR2) now begins with an alignment of all reads to the annotated transcriptome. The addition of this ungapped alignment step was designed to improve small or microexon detection for error-containing, spliced reads which are difficult to align to the genome.

□ BRAKER3: Fully Automated Genome Annotation Using RNA-Seq and Protein Evidence with GeneMark-ETP, AUGUSTUS and TSEBRA
>> https://www.biorxiv.org/content/10.1101/2023.06.10.544449v1
BRAKER3 pipeline that builds on GeneMark-ETP and AUGUSTUS and further improves accuracy using the TSEBRA combiner. BRAKER3 outperforms its predecessors BRAKER1 and BRAKER2 by a large margin, as well as publicly available pipelines, such as MAKER2, FINDER and Funannotate.

□ GRACE: a comprehensive web-based platform for integrative single-cell transcriptome analysis
>> https://academic.oup.com/nargab/article/5/2/lqad050/7192646
GRACE (GRaphical Analyzing Cell Explorer) enables online massive single-cell transcriptome analysis. GRACE provides easy access to interactive visualization, customized parameters, and publication-quality graphs.
GRACE comprehensively integrates preprocessing, clustering, developmental trajectory inference, cell-cell communication, cell-type annotation, subcluster analysis, and pathway enrichment.

□ Albert Vilella
It seems like the fragment length distribution of $ILMN Illumina's CLR tech comes from this tagmentation step.

□ DeCOr-MDS: Orthogonal outlier detection and dimension estimation for improved MDS embedding of biological datasets https://www.biorxiv.org/content/10.1101/2023.02.13.528380v2
Multidimensional scaling (MDS) is a commonly used and fast method of data exploration and dimension reduction, with the unique capacity to take non-euclidean dissimilarities as its input.
DeCOr-MDS takes advantage of geometrical characteristics of the data to reduce the influence of orthogonal outliers, and estimate the dimension of the dataset. DeCOr-MDS addresses the challenge of the presence of orthogonal outliers in high dimensional space.

□ SnapCCESS: Ensemble deep learning of embeddings for clustering multimodal single-cell omics data
>> https://academic.oup.com/bioinformatics/advance-article/doi/10.1093/bioinformatics/btad382/7197799
SnapCCESS uses VAE and the snapshot ensemble learning technique to learn multiple embeddings each encoding multiple data modalities, and subsequently generate consensus clusters for multimodal single-cell omics data by combining clusters from each embedding.
SnapCCESS encodes features from multiple data modalities into a latent space using the VAE component of Matilda framework. SnapCCESS concatenates the output from the encoder trained from each data modality to perform joint learning using a fully connected layer with 100 neurons.

□ Implementation of Nanopore sequencing as a pragmatic workflow for copy number variant confirmation in the clinic
>> https://translational-medicine.biomedcentral.com/articles/10.1186/s12967-023-04243-y
Adaptive sampling enables real-time selection of DNA molecules in user-specified genomic target regions, which generates adequate on-target depth using a single flow cell for each sample. This test can be used as a clinical assay to confirm CNVs in samples from patients w/ NDDs.

□ RegCloser: a robust regression approach to closing genome gaps
>> https://bmcbioinformatics.biomedcentral.com/articles/10.1186/s12859-023-05367-0
RegCloser represents read coordinates and their overlaps by parameters and observations in a linear regression model. The optimal overlap is searched only in the restricted range consistent with insert sizes. The local DNA assembly becomes a robust parameter estimation problem.
RegCloser solves the problem by a customized robust regression procedure that resists the influence of false overlaps by optimizing a convex global Huber loss function. The global optimum is obtained by iteratively solving the sparse system of linear equations.
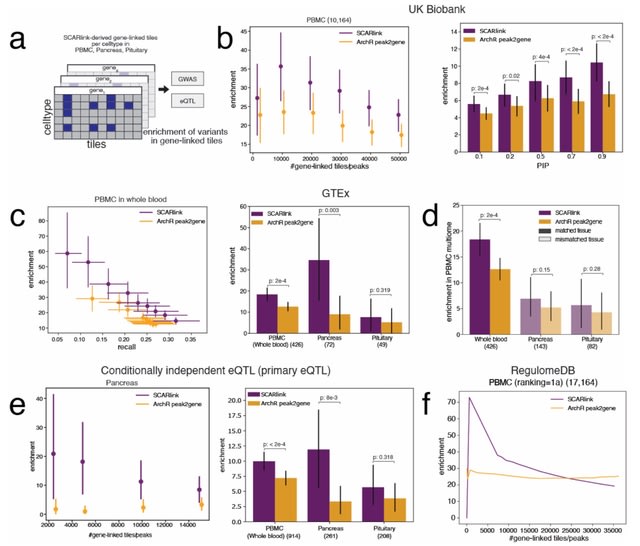
□ SCARlink: Single-cell multiome regression models identify functional and disease-associated enhancers and enable chromatin potential analysis
>> https://www.biorxiv.org/content/10.1101/2023.06.13.544851v1
SCARlink uses regularized Poisson regression on tile-level accessibility data to jointly model all regulatory effects at a gene locus, avoiding the limitations of pairwise gene-peak correlations and dependence on a peak atlas.
SCARlink captures the fact that elements both within the genic locus (e.g. intronic enhancers) and distal elements in flanking regions (+/- 250kb by default) jointly regulate expression of the gene. SCARlink can be used to identify putatively causal cell types for the variant action.

□ panacus: a tool for computing statistics for GFA-formatted pangenome graphs
>> https://github.com/marschall-lab/panacus

□ BIC: Defining the single base importance of human mRNAs and lncRNAs
>> https://www.biorxiv.org/content/10.1101/2023.06.12.544536v1
Base Importance Calculator (BIC), an algorithm to calculate the importance score of single bases based on sequence information of human mRNAs and long noncoding RNAs (lncRNAs).
BIC can effectively evaluate the pathogenicity of both genes and single bases by analyzing the BIC scores and the pathogenicity of Single Nucleotide Variations.
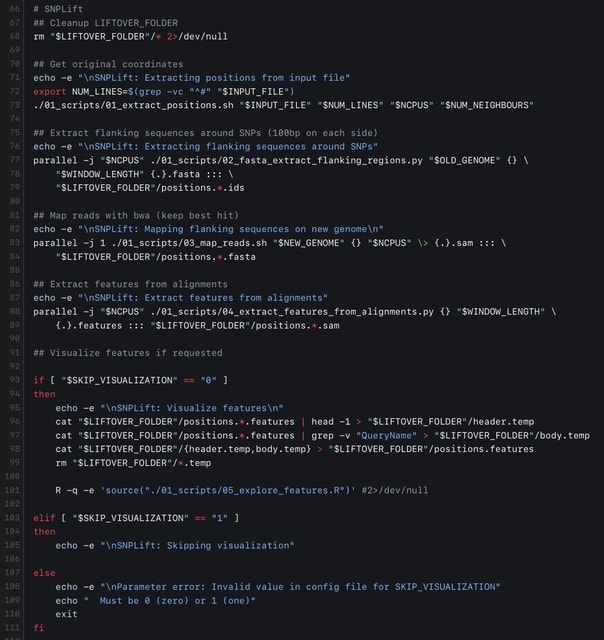
□ SNPLift: Fast and accurate conversion of genetic variant coordinates across genome assemblies
>> https://www.biorxiv.org/content/10.1101/2023.06.13.544861v1
SNPLift efficiently transfers coordinates, from VCF / other formats, from one version of a genome to another. SPLift enables the rapid utilisation of the valuable resources provided by updated reference genomes, mitigating the need for extensive / resource-intensive re-analyses.
SPLift extracts features to calculate scores for each marker. When transferring millions of positions, once the genome is indexed, SNPLift will typically transfer between 0.5 and 1 million positions per minute.