

□ Reconstructing temporal and spatial dynamics in single-cell experiments
>> https://www.biorxiv.org/content/biorxiv/early/2019/07/09/697151.full.pdf
MAPiT(MAP of pseudotime into Time), an universal transformation method that recovers real-time dynamics of cellular processes from pseudotime scales.
MAPiT resolves the arbitrariness of pseudotime by nonlinearly transforming pseudotime to the true scale of the process.
MAPiT recovers spatial positions of cells within spheroids from flow cytometric data. Spatial information can be recovered by applying MAPiT to pseudotime trajectories.
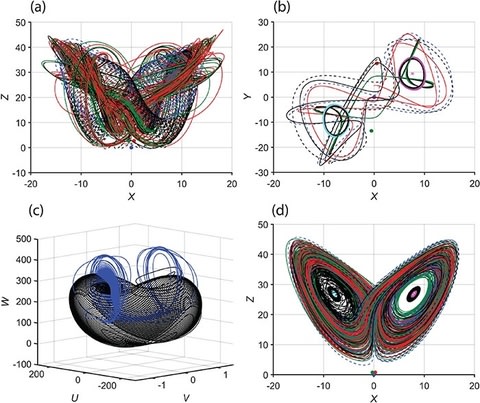
□ the Lorenz system to a six-dimensional system by incorporating rotation and density-affecting scalar. The rich dynamics and self-synchronization in the new system are explored.
>> https://aip.scitation.org/doi/10.1063/1.5095466
The new six-dimensional system is found to self-synchronize, and surprisingly, the transfer of solutions to only one of the variables is needed for self-synchronization to occur.
This study contributes to the mathematical field of nonlinear dynamics and chaos theory and is an important step toward bringing the existing Lorenz models closer to reality.
□ Megalodon: basecalling augmentation for raw nanopore sequencing reads
>> https://github.com/nanoporetech/megalodon
Megalodon provides "basecalling augmentation" for raw nanopore sequencing reads, including direct, reference-guided SNP and modified base calling.
Megalodon anchors the information rich neural network basecalling output to a reference genome. Variants, modified bases or alternative canonical bases, are then proposed and scored in order to produce highly-accurate reference anchored modified base or SNP calls.

□ FAUST: A new data-driven cell population discovery and annotation method for single-cell data
>> https://www.biorxiv.org/content/biorxiv/early/2019/07/13/702118.full.pdf
non-parametric method for unbiased cell population discovery in single-cell flow and mass cytometry that annotates cell populations with biologically interpretable phenotypes through a new procedure called Full Annotation Using Shape-constrained Trees (FAUST).
FAUST’s phenotypic annotations enable cross-study data integration and multivariate analysis in the presence of heterogeneous data and diverse immunophenotyping staining panels, demonstrating FAUST is a powerful method for unbiased discovery in single-cell data.
□ DeepMetaPSICOV: Prediction of inter‐residue contacts in CASP13
>> https://onlinelibrary.wiley.com/doi/abs/10.1002/prot.25779
DeepMetaPSICOV is a new deep learning‐based contact prediction tool, together with new methods and data sources for alignment generation.
DeepMetaPSICOV evolved from MetaPSICOV and DeepCov and combines the input feature sets used by these methods as input to a deep, fully convolutional residual neural network.


□ Corticall: Detection of simple and complex de novo mutations without, with, or with multiple reference sequences
>> https://www.biorxiv.org/content/biorxiv/early/2019/07/11/698910.full.pdf
Corticall, a graph-based method that combines the advantages of multiple technologies and prior data sources to detect arbitrary classes of genetic variant.
Corticall constructs multi-sample, coloured de Bruijn graphs from short- read data for all samples, align long-read-derived haplotypes and multiple reference data sources to restore graph connectivity information, and call variants using graph path-finding algorithms.

□ Inference of selection from genetic time series using various parametric approximations to the Wright-Fisher model
>> https://www.biorxiv.org/content/biorxiv/early/2019/07/10/696955.full.pdf
With the increase in genomic data, the evolution of genetic diversity in time becomes accessible either as a by-product of data accumulation or through dedicated projects in artificial populations (e.g. experimental evolution) or natural settings.
a new generic Hidden Markov Model likelihood calculator and applied it on genetic time series simulated under various evolutionary scenarios.
The Beta-with-Spikes approximation, which combines discrete fixation probabilities with a continuous Beta distribution, was found to perform consistently better than the others.
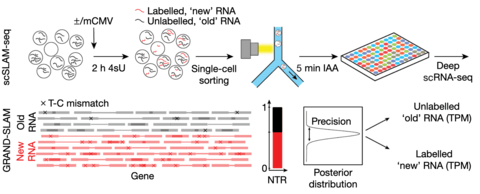
□ GRAND-SLAM: scSLAM-seq reveals core features of transcription dynamics in single cells
>> https://www.nature.com/articles/s41586-019-1369-y
GRAND-SLAM 2.0 for the parallel analysis of hundreds of SLAM-seq libraries derived from single cells.
The accuracy of quantification is further improved by analysing long reads (150 nucleotides) in paired-end mode, which allows 4sU conversions to be reliably distinguished from sequencing errors within the overlapping sequences.
‘globally refined analysis of newly transcribed RNA and decay rates using SLAM-seq’ (GRAND-SLAM)—a Bayesian method to compute the ratio of new to total RNA (NTR) in a fully quantitative manner including credible intervals.
□ Cooler: scalable storage for Hi-C data and other genomically-labeled arrays
>> https://academic.oup.com/bioinformatics/advance-article-abstract/doi/10.1093/bioinformatics/btz540/5530598
a file format called cooler, based on a sparse data model, that can support genomically-labeled matrices at any resolution.
Cooler has the flexibility to accommodate various descriptions of the data axes (genomic coordinates, tracks and bin annotations), resolutions, data density patterns, and metadata.

□ Cardigan: Disease gene prediction for molecularly uncharacterized diseases
>> https://journals.plos.org/ploscompbiol/article?id=10.1371/journal.pcbi.1007078
Cardigan (ChARting DIsease Gene AssociatioNs), uses semi-supervised learning and exploits a measure of similarity between disease phenotypes.
Cardigan uses an updatable disease phenotype similarity, and employs a non-linear transformation to define a prior probability distribution over the genes that mimics the distribution of disease genes in the interactome.

□ Scale free topology as an effective feedback system
>> https://www.biorxiv.org/content/biorxiv/early/2019/07/10/696575.full.pdf
This mapping provides a parametrization of scale free topology which is predictive at the ensemble level and also retains properties of individual realizations
Combining feedback analysis with mean field theory predicts a transition between convergent and divergent dynamics which is corroborated by numerical simulations.
□ SchNet: A deep learning architecture for molecules and materials
>> https://aip.scitation.org/doi/full/10.1063/1.5019779
the deep learning architecture SchNet that is specifically designed to model atomistic systems by making use of continuous-filter convolutional layers.
SchNet predicts potential-energy surfaces and energy-conserving force fields for molecular dynamics simulations of molecules & perform an exemplary study on the quantum-mechanical properties of C20-fullerene that would have been infeasible w/ regular ab initio molecular dynamics.
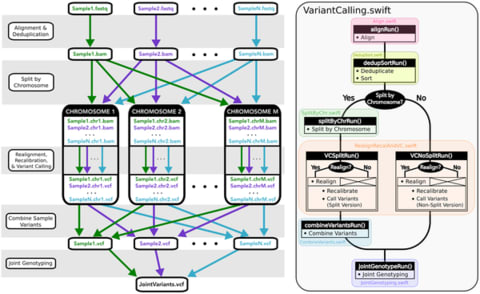
□ Managing genomic variant calling workflows with Swift/T
>> https://journals.plos.org/plosone/article?id=10.1371/journal.pone.0211608
Swift/T operates transparently in multiple cluster scheduling environments (PBS Torque, SLURM, Cray aprun environment, etc.), thus a single workflow is trivially portable across numerous clusters.
While Swift/T’s data-level parallelism eliminates the need to code parallel analysis of multiple samples, it does make debugging more difficult, as is common for implicitly parallel code.
□ linker: Comparison of single and module-based methods for modeling gene regulatory networks
>> https://academic.oup.com/bioinformatics/advance-article-abstract/doi/10.1093/bioinformatics/btz549/5530163
generating modules of co-expressed genes which are predicted by a sparse set of regulators using a variational bayes method, and then building a bipartite graph on the generated modules using LASSO,
yields more informative networks---as measured by the rate of enriched elements and a thorough network topology assessment---than previous single and module-based network approaches.
the proposed method produces networks closer to a scale-free topology, and the modules show up to 10x more enriched elements than when using single gene networks using TCGA data.
□ MCMCtreeR: functions to prepare MCMCtree analyses and visualise posterior ages on trees
>> https://academic.oup.com/bioinformatics/advance-article-abstract/doi/10.1093/bioinformatics/btz554/5530964
MCMCtree provides functions to refine parameters and visualise time-calibrated node prior distributions so that these priors accurately reflect confidence in known, usually fossil, time information.
Options also allow for the inclusion of the geological timescale, and these plotting functions are applicable with posterior age estimates from any Bayesian divergence-time estimation software.
□ MetaMaps: Strain-level metagenomic assignment and compositional estimation for long reads
>> https://www.nature.com/articles/s41467-019-10934-2
MetaMaps implements a two-stage analysis procedure. First, a list of possible mapping locations for each long read is generated using a minimizer-based approximate mapping strategy.
Second, each mapping location is scored probabilistically using a model developed here, and total sample composition is estimated using the EM algorithm.
MetaMaps utilizes a mapping approach enables MetaMaps to determine individual read mapping locations, estimated alignment identities, and mapping qualities.

□ Latent ODEs for Irregularly-Sampled Time Series
>> https://arxiv.org/pdf/1907.03907.pdf
generalizing RNNs to have continuous-time hidden dynamics defined by ordinary differential equations (ODEs).
Both ODE-RNNs and Latent ODEs can naturally handle arbitrary time gaps between observations, and can explicitly model the probability of observation times using Poisson processes.

□ Causal Inference Engine: A platform for directional gene set enrichment analysis and inference of active transcriptional regulators
>> https://www.biorxiv.org/content/biorxiv/early/2019/07/11/698852.full.pdf
a parallelized R-package for fast and flexible directional enrichment analysis that can run the inference on any user provided custom regulatory network.
Multiple inference algorithms are provided within the CIE platform along with regulatory networks from curated sources TRRUST and TRED as well as a causal protein-gene interactions derived from the STRINGdb.

□ Mathematical model of molecular evolution through a stochastic analysis
>> https://www.biorxiv.org/content/biorxiv/early/2019/07/11/699264.full.pdf
it is possible to make a mathematical model not only of mutations on the genome of species, but of evolution itself, including factors such as artificial and natural selection.
it also corresponds to the observed characteristics of evolution, no edge has a null value means that molecular evolution cannot be separated into several Markov chains while it also let us affirm that molecular evolution will never arrive to an equilibrium point nor finish.
□ A unified framework for geneset network analysis
>> https://www.biorxiv.org/content/biorxiv/early/2019/07/11/699926.full.pdf
PyGNA facilitates the integration with workflow systems, such as Snakemake, thus lowering the barrier to introduce network analysis in existing pipelines.
Python Gene Network Analysis (PyGNA) is designed with modularity in mind and to take advantage of multi-core processing available in most high-performance computing facilities.

□ CoGAPS 3: Bayesian non-negative matrix factorization for single-cell analysis with asynchronous updates and sparse data structures
>> https://www.biorxiv.org/content/biorxiv/early/2019/07/11/699041.full.pdf
CoGAPS as a sparse, Bayesian NMF approach for bulk and single-cell genomics analysis.
CoGAPS was designed to perform Gibbs sampling for a unique prior distribution that adapts the level of sparsity to the distribution of expression values in each gene and cell.
a new method for isolating the sequential portion of CoGAPS so that the majority of the algorithm can be run in parallel.

□ TADpole: Hierarchical chromatin organization detection
>> https://www.biorxiv.org/content/biorxiv/early/2019/07/11/698720.full.pdf
TADpole combines principal component analysis and constrained hierarchical clustering to provide an unsupervised set of significant partitions in a genomic region of interest.
TADpole identification of domains is robust to the data resolution, normalization strategy, and sequencing depth.
□ Guidelines for cell-type heterogeneity quantification based on a comparative analysis of reference-free DNA methylation deconvolution software
>> https://www.biorxiv.org/content/biorxiv/early/2019/07/11/698050.full.pdf
general guidelines for the development of reference-free deconvolution pipelines and define a benchmark pipeline to catalyze further application and improvement of reference-free deconvolution methods.
Deconvolution algorithms evaluation will then be significantly improved with the generation of dedicated in vivo benchmarking dataset.
□ Inferring the genetic architecture of expression variation from replicated high throughput allele-specific expression experiments
>> https://www.biorxiv.org/content/biorxiv/early/2019/07/11/699074.full.pdf
a beta-binomial model that estimates the cis-effect for each gene while permitting overdispersion of variance among replicates.
the beta-binomial model and binomial model differ by ~5% in the number of significant cis affected genes, which is less than the 15% - 25% difference in false-positive rate estimated from the null data.
This could perhaps be explained by the possibility that the two strains are sufficiently diverse that most of the genes are true positives.
□ Multivariate GWAS: Generalized Linear Models, Prior Weights, and Double Sparsity
>> https://www.biorxiv.org/content/biorxiv/early/2019/07/11/697755.full.pdf
extend and efficiently implement iterative hard thresholding (IHT) for multivariate regression.
This extensions accommodate generalized linear models (GLMs), prior information on genetic variants, and grouping of variants.
For GWAS, the sparsity model-size constant k also has a simpler and more intuitive interpretation than the lasso tuning constant λ.
□ Tree-weighting for multi-study ensemble learners
>> https://www.biorxiv.org/content/biorxiv/early/2019/07/11/698779.full.pdf
incorporating multiple layers of ensembling in the training process increases the robustness of the resulting predictor.
exploring the mechanisms by which the ensembling weights correspond to the internal structure of trees to shed light on the important features in determining the relationship between the Random Forests algorithm and the true outcome model.

□ VolcanoFinder: genomic scans for adaptive introgression
>> https://www.biorxiv.org/content/biorxiv/early/2019/07/11/697987.full.pdf
A genome-scan method—VolcanoFinder—to detect recent events of adaptive introgression using polymorphism data from the recipient species only.
VolcanoFinder detects adaptive introgression sweeps from the pattern of excess intermediate-frequency polymorphism they produce in the flanking region of the genome, a pattern which appears as a volcano-shape in pairwise genetic diversity.

□ DeePaC: Predicting pathogenic potential of novel DNA with reverse-complement neural networks
>> https://academic.oup.com/bioinformatics/advance-article-abstract/doi/10.1093/bioinformatics/btz541/5531656
DeePaC is a python package and a CLI tool for predicting labels (e.g. pathogenic potentials) from short DNA sequences (e.g. Illumina reads) with reverse-complement neural networks.
DeePaC includes a flexible framework allowing easy evaluation of neural architectures with reverse-complement parameter sharing. convolutional neural networks and LSTMs outperform the state-of-the-art based on both sequence homology and machine learning.
□ Refgenie: a reference genome resource manager
>> https://www.biorxiv.org/content/biorxiv/early/2019/07/12/698704.full.pdf
Refgenie is full-service reference genome manager that organizes storage, access, and transfer of reference genomes.
Refgenie provides programmatic access to a standard genome folder structure, so software can swap from one genome to another.
□ RESCUE: imputing dropout events in single-cell RNA-sequencing data
>> https://bmcbioinformatics.biomedcentral.com/articles/10.1186/s12859-019-2977-0
RESCUE (REcovery of Single-Cell Under-detected Expression), to mitigate the dropout problem by imputing gene expression levels using information from other cells with similar patterns.
To improve computation time RESCUE optionally implements the bootstrap iterations in parallel, with a reduction in total time by up to half when using 10 cores.

□ CONFINED: distinguishing biological from technical sources of variation by leveraging multiple methylation datasets
>> https://genomebiology.biomedcentral.com/articles/10.1186/s13059-019-1743-y
CONFINED, a sparse-CCA-based method to capture biologically replicable signal by leveraging shared structure between datasets.
Evaluating CONFINED on multiple datasets and sources of biological variability aside from cell-type composition, the optimal sparsity parameter for cell-type composition may not be optimal for other covariates of interest.

□ Giotto, a pipeline for integrative analysis and visualization of single-cell spatial transcriptomic data
>> https://www.biorxiv.org/content/biorxiv/early/2019/07/13/701680.full.pdf
This analysis highlights the utility of Giotto for characterizing tissue spatial organization as well as for the interactive exploration of multi-layer information in spatial transcriptomic and imaging data.
Giotto Analyzer requires as minimal input a gene-by-cell count matrix and the spatial coordinates for the centroid position of each cell.
Giotto Analyzer can be used to perform common steps similar to single-cell RNAseq analysis, such as pre-processing, feature selection, dimension reduction and unsupervised clustering.
□ TGStools: A Bioinformatics Suit to Facilitate Transcriptome Analysis of Long Reads from Third Generation Sequencing Platform
>> https://www.mdpi.com/2073-4425/10/7/519
currently no bioinformatics tools are built to automatically find nearby genomic features in order to filter transcripts.
TGStools, a package that implements multiple tools to facilitate routine transcriptome analysis, such as isoforms comparison, detecting alternative splicing (AS) pattern and lncRNAs identification.
□ PAST: Pathway Association Studies Tool
>> https://www.biorxiv.org/content/biorxiv/early/2019/07/13/691964.full.pdf
PAST is faster and more user-friendly than previous methods, requires minimal knowledge of programming languages, and is publicly available at Github, Bioconductor, CyVerse and MaizeGDB.
PAST uses as input TASSEL files that are generated as output from the General Linear or Mixed Linear Models, or files from any association analysis that has been similarly formatted, as well as genome annotations in GFF format.
□ MetaOmGraph: a workbench for interactive exploratory data analysis of large expression datasets
>> https://www.biorxiv.org/content/biorxiv/early/2019/07/14/698969.full.pdf
MetaOmGraph statistical tools include coexpression, differential expression, and differential correlation analysis, with permutation test-based options for significance assessments.
by incorporating metadata, MetaOmGraph adds extra dimensions to the analyses and provides flexibility in data exploration.

□ Janggu: Deep Learning for Genomics
>> https://www.biorxiv.org/content/biorxiv/early/2019/07/14/700450.full.pdf
The library includes dataset objects that manage the extraction and transformation of coverage information as well as fetching biological sequence directly from a range of commonly used file types, including FASTA, BAM or BIGWIG.
Janggu also exposes variant effect prediction functionality, similar as Kipoi and Selene, that allow to make use of the higher-order sequence encoding.

□ souporcell: Robust clustering of single cell RNAseq by genotype and ambient RNA inference without reference genotypes
>> https://www.biorxiv.org/content/biorxiv/early/2019/07/14/699637.full.pdf
no methods yet exist that enable deconvolving mixed samples without a priori genotype data while also accounting for doublets and ambient RNA.
souporcell, a novel method for clustering scRNAseq cells by genotype using sparse mixture model clustering with explicit ambient RNA modeling.

□ Improving interpretability of deep learning models: splicing codes as a case study
>> https://www.biorxiv.org/content/biorxiv/early/2019/07/14/700096.full.pdf
extending Integrated Gradients (IG) with nonlinear paths, embedding in latent space, alternative baselines, and a framework to identify important features which make it suitable for interpretation of deep models for genomics.
IG with nonlinear paths identify significant features missed using linear paths or simple gradients.
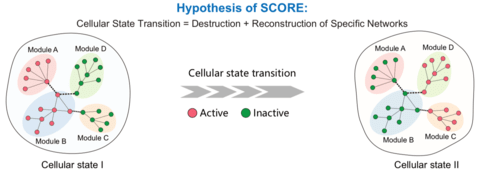
□ SCORE: Enhancing single-cell cellular state inference by incorporating molecular network features
>> https://www.biorxiv.org/content/biorxiv/early/2019/07/14/699959.full.pdf
SCORE is a network-based method, to simulate the dynamic changes of molecular networks among different cellular states. SCORE can identify crucial gene modules and construct the characteristic molecular interaction networks for a cellular state, providing more biological insights rather than mainly statistical interpretation and annotation.
□ VariantSpark: A Random Forest Machine Learning Implementation for Ultra High Dimensional Data
>> https://www.biorxiv.org/content/biorxiv/early/2019/07/15/702902.full.pdf
Recent improvements by Yggdrasil begin to address these limitations but do not extend to Random Forest.
CursedForest, a novel Random Forest implementation on top of Apache Spark and part of the VariantSpark platform, which parallelises processing of all nodes over the entire forest.
CursedForest extends Yggdrasil’s approach to Decision Trees to Random Forest models. CursedForest also introduces a novel method of paralleliza- tion in the tree growing process such that nodes of different trees are processed in parallel.
□ Evaluating probabilistic programming and fast variational Bayesian inference in phylogenetics
>> https://www.biorxiv.org/content/biorxiv/early/2019/07/15/702944.full.pdf
a tool based on the Stan package for Bayesian phylogenetic inference, the first application of variational Bayes to time trees with coalescent models.
focused on inferring phylogenetic models with a fixed topology due to the complexity and discrete nature of the topology space, recent research on subsplit Bayesian networks (SBN) has made a significant step toward modeling topological uncertainty in the variational frame-work.
In order to reconstruct temporal information, it then takes the single maximum likelihood tree and applies the TreeTime software to infer divergence times and evolutionary rates.
※コメント投稿者のブログIDはブログ作成者のみに通知されます