

□ STMF: Sparse data embedding and prediction by tropical matrix factorization
>> https://bmcbioinformatics.biomedcentral.com/articles/10.1186/s12859-021-04023-9
Sparse Tropical Matrix Factorization (STMF) introduces non-linearity into matrix factorization models, which enables discovering the most dominant patterns, leading to a more straightforward visual interpretation compared to other methods for missing value prediction.
Integrative data fusion methods are based on co-factorization of multiple data matrices. Using standard linear algebra, DFMF is a variant of penalized matrix tri-factorization, which simultaneously factorizes data matrices to reveal hidden associations.

□ GNIPLR: Inference of gene regulatory networks using pseudo-time series data
>> https://academic.oup.com/bioinformatics/advance-article-abstract/doi/10.1093/bioinformatics/btab099/6134129
GNIPLR (gene networks inference based on projection and lagged regression) infers GRNs from time-series or non-time-series gene expression data.
GNIPLR projected gene data twice using the LASSO projection (LSP) algorithm and the linear projection (LP) approximation to produce a linear and monotonous pseudo-time series, and then determined the direction of regulation in combination with lagged regression analyses.

□ FASTRAL: Improving scalability of phylogenomic analysis
>> https://academic.oup.com/bioinformatics/advance-article/doi/10.1093/bioinformatics/btab093/6130791
ASTRAL’s algorithm is the use of dyna- mic programming to find an optimal solution to the MQSST (maximum quartet support supertree) within a constraint space that it computes from the input.
FASTRAL is based on ASTRAL, but uses a different technique for constructing the constraint space. FASTRAL is a polynomial time algorithm that is statistically consistent under the multi-locus coalescent model.

□ AQC: mRNA codon optimization on quantum computers
>> https://www.biorxiv.org/content/10.1101/2021.02.19.431999v1.full.pdf
An adiabatic quantum computer (AQC) is compared to a standard genetic algorithm (GA) programmed with the same objective function. The AQC is found to be competitive in identifying optimal solutions and future generations of AQCs may be able to outperform classical GAs.
The Leap Hybrid solver is capable of solving codon optimization problems expressed as a BQM with up to ~1,000 amino acids. The goal of the optimization is to find the combination of codons that minimizes the Hamiltonian. AQCs finds the ground state of the input Hamiltonian.
□ SVFS: Dimensionality reduction using singular vectors
>> https://www.nature.com/articles/s41598-021-83150-y
Let D=[A∣b] be a labeled dataset, where b is the class label and features are columns of matrix A. SVFS uses the signature matrix SD of D to find the cluster that contains b. Then, reduce the size of A by discarding features in the other clusters as irrelevant features.
Singular-Vectors Feature Selection (SVFS) uses the signature matrix SA of reduced A to partition the remaining features into clusters and choose the most important features from each cluster.
Pseudo-inverses are used in neural learning to solve large least square systems. the complexity of Geninv on a single-threaded processor is O(min(m3,n3)) whereas in a multi-thread, the time complexity is O(min(m,n)). the complexity of SVFS algorithm is at most O(max(m3,n2)).

□ MultiMAP: Dimensionality Reduction and Integration of Multimodal Data
>> https://www.biorxiv.org/content/10.1101/2021.02.16.431421v1.full.pdf
MultiMAP recovers a single manifold on which all of the data resides and projects into a low-dimensional space so as to preserve the manifold structure. MultiMAP is based on a Riemannian geometry / algebraic topology, and generalizes the UMAP algorithm to the multimodal setting.
MultiMAP takes as input any number of datasets of potentially differing dimensions. MultiMAP recovers geodesic distances on a single latent manifold on which all of the data is uniformly distributed.
These distances are then used to construct a neighborhood graph (MultiGraph) on the manifold. the data & manifold space are projected into a low-dimensional space by minimizing the cross entropy of the graph in the embedding space with respect to the graph in the manifold space.

□ scGAE: topology-preserving dimensionality reduction for single-cell RNA-seq data using graph autoencoder
>> https://www.biorxiv.org/content/10.1101/2021.02.16.431357v1.full.pdf
scGAE builds a cell graph / uses a multitask-oriented graph autoencoder to preserve topological structure information. scGAE accurately reconstructs developmental trajectory and separates discrete cell clusters under different scenarios, outperforming other deep learning methods.
scGAE combines the deep autoencoder and graphical model to embed the topological structure of high-dimensional scRNA-seq data to a low-dimensional space. After getting the normalized count matrix, scGAE builds the adjacency matrix among cells by K-nearest-neighbor algorithm.
scGAE maps the count matrix to a low-dimensional latent space by graph attentional layers. scGAE decodes the embedded data to the spaces with the same dimension as original data by minimizing the distance between the input data and the reconstructed data.

□ CANTARE: finding and visualizing network-based multi-omic predictive models https://bmcbioinformatics.biomedcentral.com/articles/10.1186/s12859-021-04016-8
CANTARE (Consolidated Analysis of Network Topology And Regression Elements) is a workflow for building predictive regression models from network neighborhoods in multi-omic networks. CANTARE models are competitive with random forests and elastic net.
The AUC values of CANTARE models were comparable to those of random forests and penalized regressions, whether the forests or regressions were generated with the universe of multi-omic data or the data underlying the Vnet.
CANTARE models are subject to the general constraints of linear regressions, such as linearity with log odds or continuous outcomes, normal distribution of the errors, and little to no multicollinearity between predictors.
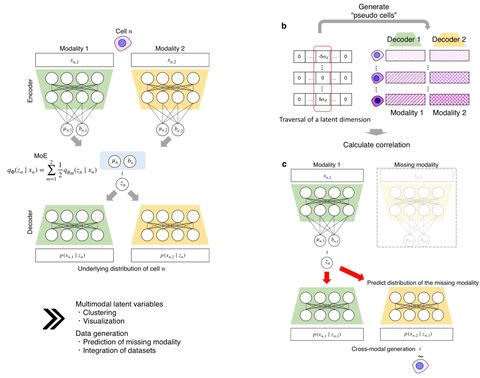
□ scMM: Mixture-of-experts multimodal deep generative model for single-cell multiomics data analysis
>> https://www.biorxiv.org/content/10.1101/2021.02.18.431907v1.full.pdf
scMM is based on a mixture-of-experts multimodal deep generative model and achieves end-to-end learning by modeling raw count data in each modality based on different probability distributions.
Using the learned standard deviation for the dth dimension σd, with other dimensions fixed to zero, and linearly changed the dth dimension from −5σd to 5σd at a rate of 0.5σd.
scMM uses a Laplace prior with different scale values in each dimension, which encourages disentanglement of information by learning axis-aligned representations.
□ SSRE: Cell Type Detection Based on Sparse Subspace Representation and Similarity Enhancement
>> https://www.sciencedirect.com/science/article/pii/S1672022921000383
SSRE computes the sparse representation similarity of cells based on the subspace theory, and designed a gene selection process and an enhancement strategy based on the characteristics of different similarities to learn more reliable similarities.
SSRE performs eigengap on the learned similarity matrix to estimate the number of clusters. Eigengap is a typical cluster number estimation method, and it determines the number of clusters by calculating max gap between eigenvalues of a Laplacian matrix.
□ AMBIENT: Accelerated Convolutional Neural Network Architecture Search for Regulatory Genomics
>> https://www.biorxiv.org/content/10.1101/2021.02.25.432960v1.full.pdf
AMBIENT maps a summary of that dataset to the initial state of the controller model and generates an optimal task-specific architecture. AMBIENT is more efficient than existing methods, allowing it to identify architectures of comparable accuracy at an accelerated pace.
AMBIENT uses a 10-layer model search space to evaluate the optimal architecture differences. And generates highly accurate CNN architectures for sequences of diverse functions, while substantially reducing the computing cost of conventional Neural Architecture Search.
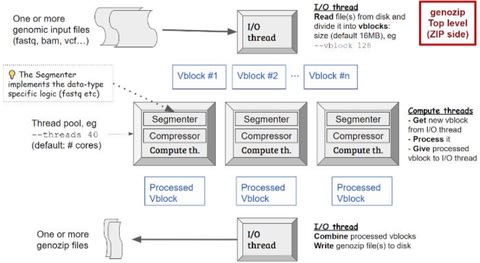
□ Genozip - A Universal Extensible Genomic Data Compressor
>> https://academic.oup.com/bioinformatics/advance-article/doi/10.1093/bioinformatics/btab102/6135077
Genozip is designed to be a general-purpose software and a development framework for genomic compression by providing five core capabilities – universality (support for all common genomic file formats), high compression ratios, speed, feature-richness, and extensibility.
Genozip supports all common genomic file formats - FASTQ, SAM/BAM/CRAM, VCF, GVF, FASTA, PHYLIP, and 23andMe. Genozip is architected with a separation of the Genozip Framework from file-format-specific Segmenters and data-type-specific Codecs.

□ AirLift: A Fast and Comprehensive Technique for Remapping Alignments between Reference Genomes
>> https://www.biorxiv.org/content/10.1101/2021.02.16.431517v1.full.pdf
AirLift, a fast and comprehensive method for moving alignments from one genome to another. AirLift reduces the number of reads that need to be fully mapped from the entire read set, and the overall execution time to remap read sets b/n two reference genome versions.
AirLift is the first tool that provides BAM-to-BAM remapping results of a read data set on which downstream analysis can be immediately performed. AirLift identifies similar rates of SNPs and Indels as the full mapping baseline.

□ iMAP: integration of multiple single-cell datasets by adversarial paired transfer networks
>> https://genomebiology.biomedcentral.com/articles/10.1186/s13059-021-02280-8
iMAP combines the two kinds of unsupervised deep network structures—autoencoders and generative adversarial networks. A novel autoencoder structure is used to build low-dimensional representations of the biological contents of cells disentangled from the technical variations.
iMAP framework consists of two stages, including building the batch-ignorant representations for all cells, and then guiding the batch effect removal of the original high-dimensional expression profiles. The input expression vectors for iMAP were log-transformed TPM-like values.
iMAP regards the cells in the mutual nearest neighbors (MNN) pairs as initial seeds, and adopts a random walk-based method to enroll new pairs, through successively selecting a cell from the kNNs (k nearest neighbors) of the seeds within each batch.
□ TransPi - a comprehensive TRanscriptome ANalysiS PIpeline for de novo transcriptome assembly
>> https://www.biorxiv.org/content/10.1101/2021.02.18.431773v1.full.pdf
TransPi utilizes various assemblers and kmers (i.e. k length sequences used for the assembly) to generate an over assembled transcriptome that is then reduced to a non-redundant consensus transcriptome with the EvidentialGene.
TransPi performs multiple assemblies with different parameters to then get a non-redundant consensus assembly. It also performs other valuable analyses such as quality assessment of the assembly, BUSCO scores, Transdecoder (ORFs), and gene ontologies (Trinotate).

□ Deep propensity network using a sparse autoencoder for estimation of treatment effects
>> https://academic.oup.com/jamia/advance-article-abstract/doi/10.1093/jamia/ocaa346/6139936
Drawing causal estimates from observational data is problematic, because datasets often contain underlying bias. To examine causal effects, it is important to evaluate what-if scenarios—the so-called counterfactuals.
DPN-SA: Architecture for propensity score matching & counterfactual prediction—Deep Propensity Network using a Sparse Autoencoder—to tackle the problems of high dimensionality, nonlinear/nonparallel treatment assignment, and residual confounding when estimating treatment effects.

□ IRIS-FGM: an integrative single-cell RNA-Seq interpretation system for functional gene module analysis
>> https://academic.oup.com/bioinformatics/advance-article-abstract/doi/10.1093/bioinformatics/btab108/6140779
IRIS-FGM (integrative scRNA-Seq interpretation system for functional gene module analysis) to support the investigation of FGMs and cell clustering using scRNA-Seq data.
Empowered by QUBIC2, IRIS-FGM can identify co-expressed and co-regulated FGMs, predict types/clusters, identify differentially expressed genes, and perform functional enrichment analysis. IRIS-FGM also applies Seurat objects that can be easily used in the Seurat vignettes.
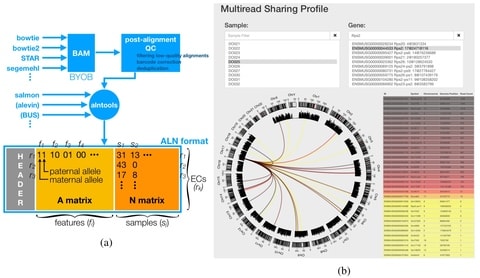
□ ALN: Decoupling alignment strategy from feature quantification using a standard alignment incidence data structure
>> https://www.biorxiv.org/content/10.1101/2021.02.16.431379v1.full.pdf
ALNtools processes next-generation sequencing read alignments into a sparse compressed incidence matrix and stores it in a pre-defined binary format for efficient downstream analyses. It enables us to compare, contrast, or combine the results of different alignment strategies.
ALN uses EMASE-Zero algorithm, In combination with alntools (that generates compressed three-dimensional incidence matrix), Zero estimates the expected read counts fast, over 10 times faster than RSEM. Zero generalizes the fast hierarchical EM to any decent alignment strategies.
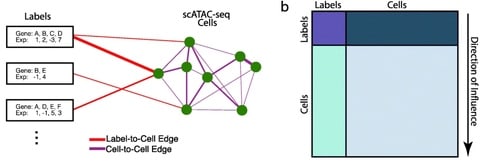
□ CellWalker integrates single-cell and bulk data to resolve regulatory elements across cell types in complex tissues
>> https://genomebiology.biomedcentral.com/articles/10.1186/s13059-021-02279-1
Using a graph diffusion implemented via a random walk with restarts, CellWalker computes a global influence matrix that relates every cell and label to every other cell and label based on information flow between them in the network.
CellWalker takes as input scATAC-seq data and labeling information, either directly in the form of marker genes, or by processing scRNA-seq data to generate labels (for example using Seurat). scATAC-seq data can optionally be converted into a cell-by-gene matrix using software such as SnapATAC, Cicero, or ArchR.

□ META-CS: Accurate SNV detection in single cells by transposon-based whole-genome amplification of complementary strands
>> https://www.pnas.org/content/118/8/e2013106118
META-CS achieved the highest accuracy in terms of detecting single-nucleotide variations, and provided potential solutions for the identification of other genomic variants, such as insertions, deletions, and structural variations in single cells.
with META-CS, a mutation can be identified with as few as four reads, which significantly reduces sequencing cost. In contrast to the 30 to 60× sequencing depth commonly used for single-cell SNV identification, most cells were sequenced between 3 and 8× in this work.

□ RaptGen: A variational autoencoder with profile hidden Markov model for generative aptamer discovery
>> https://www.biorxiv.org/content/10.1101/2021.02.17.431338v1.full.pdf
RaptGen, a variational autoencoder for aptamer generation. RaptGen uses a profile hidden Markov model decoder to efficiently create latent space in which sequences form clusters based on motif structure.
RaptGen learns the relationship b/n sequencing data and latent space embeddings. RaptGen constructs a latent space based on sequence similarity. And can propose candidates according to the activity distribution by transforming a latent representation into a probabilistic model.

□ PhylEx: Accurate reconstruction of clonal structure via integrated analysis of bulk DNA-seq and single cell RNA-seq data
>> https://www.biorxiv.org/content/10.1101/2021.02.16.431009v1.full.pdf
PhylEx: a clonal-tree reconstruction method that integrates bulk genomics and single-cell transcriptomics data. In addition to the clonal-tree, PhylEx also assigns single-cells to clones, which effectively produce clonal expression profiles, and generates clonal genotypes.
PhylEx improves over bulk-based clone reconstruction method and should be the preferred choice for inferring the guide tree needed for Cardelino. PhylEx is a strong alternative to DLP scDNA-seq for mapping expression profiles to clones using methods such as clonealign.
□ coupleCoC+: an information-theoretic co-clustering-based transfer learning framework for the integrative analysis of single-cell genomic data
>> https://www.biorxiv.org/content/10.1101/2021.02.17.431728v1.full.pdf
coupleCoC+ uses the linked features in the two datasets for effective knowledge transfer, and it also uses the information of the features in the target data that are unlinked with the source data.
coupleCoC+ can automatically adjust for sequencing depth, so we do not need to normalize for sequencing depth. coupleCoC+ is guaranteed to converge as the objective functions in Equations are non-increasing in each iteration.

□ multistrain SIRS: Localization, epidemic transitions, and unpredictability of multistrain epidemics with an underlying genotype network
>> https://journals.plos.org/ploscompbiol/article?id=10.1371/journal.pcbi.1008606
a multistrain Susceptible-Infectious-Recovered-Susceptible (multistrain SIRS) epidemic model with an underlying genotype network, allowing the disease to evolve along plausible mutation pathways as it spreads in a well-mixed population.
the genotype network do not affect the classic epidemic threshold but localize outbreaks around key strains and yield a second immune invasion threshold below which the epidemics follow almost cyclical and chaos-like dynamics.
□ Squidpy: a scalable framework for spatial single cell analysis
>> https://www.biorxiv.org/content/10.1101/2021.02.19.431994v1.full.pdf
Spatial graphs encode spatial proximity, and are, depending on data resolution, flexible in order to support the variety of neighborhood metrics that spatial data types and users may require.
Squidpy implements a pipeline based on Scikit-image for preprocessing and segmenting images, extracting morphological, texture, and deep learning-powered features. Squidpy’s Image Container stores the image with an on-disk/in-memory switch based on xArray and Dask.
□ VSAT: Variant-set association test for generalized linear mixed model
>> https://onlinelibrary.wiley.com/doi/10.1002/gepi.22378
An adjustment in the generalized linear mixed model (GLMM) framework, which accounts for both sample relatedness and non-Gaussian outcomes, has not yet been attempted.
a new Variant-Set Association Test (VSAT), a powerful and efficient analysis tool in GLMM, to examine the association between a set of omics variants and correlated phenotypes.

□ Estimating DNA methylation potential energy landscapes from nanopore sequencing data
>> https://www.biorxiv.org/content/10.1101/2021.02.22.431480v1.full.pdf
a novel approach that characterizes the probability distribution of methylation within a genomic region of interest using a parametric correlated potential energy landscape (CPEL) model that is consistent with methylation means and pairwise correlations at each CpG site.
an estimation approach based on the expectation-maximization (EM) algorithm. This method determines values for the parameters of the CPEL model by maximizing the likelihood that the observed nanopore sequencing data have been generated by the estimated model.
Within each DNA fragment, the C’s of all CG dinucleotide marked by 1 are replaced with M’s, a step that modifies the DNA sequence within each fragment by incorporating the methylation, as determined by the methylation states drawn from the ground truth CPEL model.
< br />
□ NanoMethPhase: Megabase-scale methylation phasing using nanopore long reads
>> https://genomebiology.biomedcentral.com/articles/10.1186/s13059-021-02283-5
SNVs from nanopore sequencing data using Clair. Clair is designed to call germline small variants from nanopore reads based on pileup format, and the authors demonstrated its superiority over other pileup-based tools.
NanoMethPhase and SNVoter detect allele-specific methylation (ASM) from a single sample using only nanopore sequence data with redundant sequence coverage as low as about 10×.
□ GuideStar: bioinformatics tool for gene characterization-case study:
>> https://www.biorxiv.org/content/10.1101/2021.02.25.432957v1.full.pdf
GUIdeStaR, a ready-to-plug-in-to-AI database integrated with five important nucleotide elements and structure, G-quadruplex, Uorf, IRES, Small RNA, Repeats.
□ Normalization of single-cell RNA-seq counts by log(x + 1) or log(1 + x)
>> https://academic.oup.com/bioinformatics/advance-article/doi/10.1093/bioinformatics/btab085/6155989
while it doesn’t matter whether one uses log(x + 1) or log(1 + x), the filtering and normalization applied to counts can affect comparative estimates in non-intuitive ways.
the SCnorm normalization is based on a preliminary filter for all cells with at least one count. Indeed, there have been reports of problems with SCnorm when applying the method to sparse datasets with many zeroes.
□ Demographic inference from multiple whole genomes using a particle filter for continuous Markov jump processes
>> https://journals.plos.org/plosone/article?id=10.1371/journal.pone.0247647
The algorithm relies on Radon-Nikodym derivatives, and establish criteria for choosing a finite set of “waypoints” that makes it possible to reduce the problem to the discrete-time case, while ensuring that particle degeneracy remains under control.
The Auxiliary Particle Filter for discrete-time models, generalise it to continuous-time and -space Markov jump processes. And use Variational Bayes to model the uncertainty in parameter estimates for rare events, avoiding biases seen with Expectation Maximization.

□ ASpli: Integrative analysis of splicing landscapes through RNA-Seq assays
>> https://academic.oup.com/bioinformatics/advance-article/doi/10.1093/bioinformatics/btab141/6156815
ASpli, a computational suite implemented in R statistical language, that allows the identification of changes in both, annotated and novel alternative splicing events and can deal with simple, multi-factor or paired experimental designs.
ASpli considers the same GLM model, applied to different sets of reads and junctions, in order to compute complementary splicing signals. the consolidation of these signals resulted in a robust proxy of the occurrence of splicing alterations.

□ StationaryOT: Optimal transport analysis reveals trajectories in steady-state systems
>> https://www.biorxiv.org/content/10.1101/2021.03.02.433630v1.full.pdf
The problem of inferring cell trajectories from single-cell measure- ments has been a major topic in the single-cell analysis community, with different methods developed for equilibrium and non-equilibrium systems.
StationaryOT, is mathematically motivated in a natural way from the hypothesis of a Waddington’s metaphor of an epigenetic landscape. StationaryOT with either entropic or quadratic regularisation consistently produces more accurate fate estimates compared to the scVelo method.
□ Mako: a graph-based pattern growth approach to detect complex structural variants
>> https://www.biorxiv.org/content/10.1101/2021.03.01.433465v1.full.pdf
Though long read sequencing technologies bring us promising opportunities to characterize CSVs, their application is currently limited to small-scale projects and the methods for CSV discovery are also underdeveloped.
Mako, utilizing a bottom-up guided model-free strategy, to detect CSVs from paired-end short-read sequencing. Mako uses a graph to build connections of mutational signals derived from abnormal alignment, providing the potential breakpoint connections of CSVs.