
Zweifellos Ist aber Einer.
Der Kann täglich es ändern. Kaum bedarfer Gesez.
Denn nicht vermögen Die Himmlischen alles.
Nemlich es reichen Die Sterblichen eh'an den Abgrund.Also wendet es sich,das Echo Mit diesen.
Lang ist Die Zeit,es ereignet sich aber Das Wahre.
しかし疑いもなく ひとりの者が存在する。
この者は日ごとに 世の成り行きを変えることができる。
この者に掟はほとんど 用をなさない。
なぜなら天上の者たちも
すべてのことはなし得ないのだから
すなわち死すべき者たちは いち早く深淵に突き当たり、
そこで彼らはエコーとともに 方向を転じるのだ。
時の歩みは悠遠だが、それでも眞実のものは 現れ出る。
──『Mnemosyne.』(第2稿/断章)
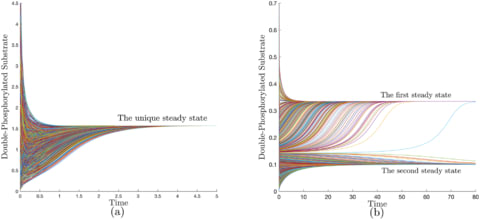
□ LEARN: A computational framework for a Lyapunov-enabled analysis of biochemical reaction networks
>> https://journals.plos.org/ploscompbiol/article?id=10.1371/journal.pcbi.1007681
a class of networks that are “structurally (mono) attractive” meaning that they are incapable of exhibiting multiple steady states, oscillation, or chaos by virtue of their reaction graphs. These networks are characterized by the existence of a universal energy-like function.
Robust Lyapunov function (RLF), a finite set of rank-one linear systems is introduced, which form the extremals of a linear convex cone.
LEARN (Lyapunov-Enabled Analysis of Reaction Networks) is provided that constructs such functions or rules out their existence.

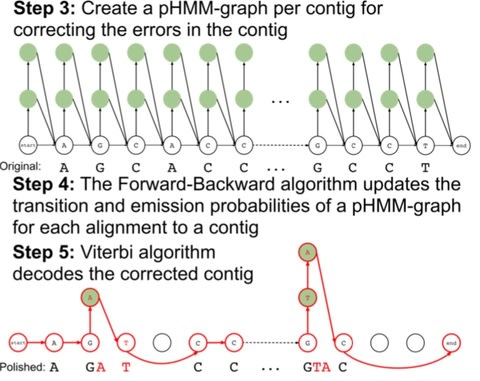
□ Apollo: A Sequencing-Technology-Independent, Scalable, and Accurate Assembly Polishing Algorithm
>> https://academic.oup.com/bioinformatics/advance-article-abstract/doi/10.1093/bioinformatics/btaa179/5804978
Apollo constructs a profile hidden Markov model graph (pHMM-graph) to represent the sequences of contig as well as the errors that a contig may have. A pHMM-graph includes states and directed transitions from a state to another.
Apollo is the only algorithm that uses reads from any sequencing technology within a single run and scales well to polish large assemblies without splitting the assembly into multiple parts.
Apollo models an assembly as a profile hidden Markov model (pHMM), uses read-to- assembly alignment to train the pHMM with the Forward-Backward algorithm, and decodes the trained model with the Viterbi algorithm to produce a polished assembly.
□ CReSCENT: CanceR Single Cell ExpressioN Toolkit
>> https://www.biorxiv.org/content/10.1101/2020.03.27.012740v1.full.pdf
CReSCENT’s interactive data visualizations allow users to deeply explore features of interest without re-running pipelines, as well as overlay additional, custom meta-data such as cell types or T cell receptor sequences.
CReSCENT provides Seurat’s clustering algorithm, and parallelization via the R library, ‘future’. Non-linear dimension reduction are used to visualize cells in a two-dimensional space according to features of interest, such as cell clusters, GE, or cell metadata.
CReSCENT requires a gene expression matrix in Matrix Market format (MTX) as input. Compared to gene-by-barcode text files, the MTX format requires less storage space for sparse matrices where many elements are zeros, as is often the case for scRNA-seq data sets.

□ Merqury: reference-free quality and phasing assessment for genome assemblies
>> https://www.biorxiv.org/content/10.1101/2020.03.15.992941v1.full.pdf
Merqury, a novel tool for reference-free assembly evaluation based on efficient k-mer set operations. Merqury provides an efficient way of determining phase blocks in diploid assemblies.
By comparing k-mers in a de novo assembly to those found in unassembled high-accuracy reads, Merqury estimates base-level accuracy and completeness.

□ rGFA: The design and construction of reference pangenome graphs
>> https://arxiv.org/pdf/2003.06079.pdf
reference Graphical Fragment Assembly (rGFA), a graph-based data model and associated formats to represent multiple genomes while preserving the coordinate of the linear reference genome, and developed a new Graphical mApping Format (GAF).
the reference GFA (rGFA) format encodes reference pangenome graphs. rGFA is an extension to GFA with three additional tags that indicate the origin of a segment from linear genomes, and also report a path or walk in the stable coordinate.
In rGFA, each segment is associated with one origin. This apparently trivial requirement in fact imposes a strong restriction on the types of graphs rGFA can encode: it forbids the collapse of different regions from one sequence, which would often happen in a cDBG.

□ HiCanu: accurate assembly of segmental duplications, satellites, and allelic variants from high-fidelity long reads
>> https://www.biorxiv.org/content/10.1101/2020.03.14.992248v1.full.pdf
HiCanu outputs contigs as “pseudo-haplotypes” that preserve local allelic phasing but may switch between haplotypes across longer distances.
On the effectively haploid CHM13 human cell line, HiCanu achieved an NG50 contig size of 77 Mbp with a per-base consensus accuracy of 99.999% (QV50), surpassing recent assemblies of high-coverage, ultra-long Oxford Nanopore reads in terms of both accuracy and continuity.

□ CONSENT: Scalable long read self-correction and assembly polishing with multiple sequence alignment
>> https://www.biorxiv.org/content/10.1101/546630v4.full.pdf
CONSENT (sCalable self-cOrrectioN of long reads with multiple SEquence alignmeNT) is a self-correction method for long reads. It works by, first, computing overlaps between the long reads, in order to define an alignment pile for each read.
CONSENT computes actual multiple sequence alignments, using a method based on partial order graphs. an efficient segmentation strategy based on k-mer chaining, it allows CONSENT to efficiently scale to ONT ultra-long reads.

□ Lagrangian Neural Networks
>> https://arxiv.org/pdf/2003.04630.pdf
Lagrangian Neural Networks (LNNs), which can parameterize arbitrary Lagrangians using neural networks.
LNNs does not restrict the functional form of learned energies and will produce energy-conserving models for a variety of tasks. LNNs can be applied to continuous systems and graphs using a Lagrangian Graph Network.
In contrast to models that learn Hamiltonians, LNNs do not require canonical coordinates, and thus perform well in situations where canonical momenta are unknown or difficult to compute.


□ Neural Tangents: Fast and Easy Infinite Neural Networks
>> https://arxiv.org/pdf/1912.02803.pdf
Neural Tangents is a library designed to enable research into infinite-width neural networks. It provides a high-level API for specifying complex and hierarchical neural network architectures.
Neural Tangents provides tools to study gradient descent training dynamics of wide but finite networks in either function space or weight space.
Infinite-width networks can be trained analytically using exact Bayesian inference or using gradient descent via the Neural Tangent Kernel.

□ tradeSeq: Trajectory-based differential expression analysis for single-cell sequencing data
>> https://www.nature.com/articles/s41467-020-14766-3
tradeSeq, a powerful generalized additive model framework based on the negative binomial distribution that allows flexible inference of both within-lineage and between-lineage differential expression.
while pseudotime can be interpreted as an increasing function of true chronological time, there is no guarantee that the two follow a linear relationship.
By incorporating observation-level weights, it allows to account for zero inflation. tradeSeq infers smooth functions for the GE measures along pseudotime for each lineage. As it is agnostic to the dimensionality reduction and TI, it scales from simple to complex trajectories.

□ Statistical significance of cluster membership for unsupervised evaluation of single cell identities
>> https://academic.oup.com/bioinformatics/advance-article/doi/10.1093/bioinformatics/btaa087/5788523
a posterior probability that a cell should be included in that clustering- based subpopulation. Posterior inclusion probabilities (PIPs) for cluster memberships can be used to select and visualize samples relevant to subpopulations.
The proposed p-values and PIPs lead to probabilistic feature selection of single cells, that can be visualized using PCA, t-SNE, and others. By learning uncertainty in clustering high-dimensional data, the proposed methods enable unsupervised evaluation of cluster memberships.
□ The Beacon Calculus: A formal method for the flexible and concise modelling of biological systems
>> https://journals.plos.org/ploscompbiol/article?id=10.1371/journal.pcbi.1007651
Performance Evaluation Process Algebra (PEPA) assigned a rate to each action so that the system could be mapped onto a continuous time Markov chain (CTMC).
the Beacon Calculus, which makes it simple and concise to encode models of complex biological systems. It is a tool that builds upon the intuitive syntax of PEPA and mobility in the π-calculus to produce models.
□ HAL: Hybrid Automata Library: A flexible platform for hybrid modeling with real-time visualization
>> https://journals.plos.org/ploscompbiol/article?id=10.1371/journal.pcbi.1007635
The main components of HAL consist of n-dimensional (0D,1D,2D,3D) grids that hold Agents, 1D,2D, and 3D finite difference PDE fields, 2D and 3D visualization tools, and methods for sampling distributions and data recording.
HAL also prioritizes performance in its algorithmic implementation. HAL includes efficient PDE solving algorithms, such as the ADI (alternating direction implicit) method, and uses efficient distribution sampling algorithms.
□ SphereCon - A method for precise estimation of residue relative solvent accessible area from limited structural information
>> https://academic.oup.com/bioinformatics/advance-article/doi/10.1093/bioinformatics/btaa159/5802464
SphereCon, a method for estimating the position and volume of residue atoms in cases when they are not known from the structure, or when the structural data are unreliable or missing.
SphereCon correlates almost perfectly with the directly computed relative solvent accessibility (RSA), and outperforms other previously suggested indirect methods. SphereCon is the only measure that yield accurate results when the identities of amino acids are unknown.

□ LDVAE: Interpretable factor models of single-cell RNA-seq via variational autoencoders
>> https://academic.oup.com/bioinformatics/advance-article/doi/10.1093/bioinformatics/btaa169/5807606
a linearly decoded variational autoencoder (LDVAE), an approach based on a modification of a recently published highly scalable variational autoencoder framework that provides interpretability without sacrificing much accuracy.
interpretable non-Gaussian factor models can be linked to variational autoencoders to enable interpretable, efficient and multivariate analysis of large datasets.
To illustrate the scalability of our model, fitting a 10-dimensional LDVAE to the data which allows identification of cells similar to each other and for the determination of co-varying genes.
□ BANDITS: Bayesian differential splicing accounting for sample-to-sample variability and mapping uncertainty
>> https://genomebiology.biomedcentral.com/articles/10.1186/s13059-020-01967-8
In order to infer the posterior distribution of the model parameters, developed a Metropolis-within-Gibbs MCMC algorithm where parameters are alternately sampled in three blocks.
BANDITS uses a Bayesian hierarchical structure to explicitly model the variability between samples and treats the transcript allocation of reads as latent variables.
□ SVXplorer: Three-tier approach to identification of structural variants via sequential recombination of discordant cluster signatures
>> https://journals.plos.org/ploscompbiol/article?id=10.1371/journal.pcbi.1007737
SVXplorer first forms discordant clusters from paired-end reads via formation of maximal cliques in a weight-thresholded bidirectional graph and consolidates them further into PE-supported variants.
SVXplorer uses a graph-based clustering approach streamlined by the integration of non-trivial signatures from discordant paired-end alignments, split-reads and read depth information.
□ SG-LSTM-FRAME: a computational frame using sequence and geometrical information via LSTM to predict miRNA-gene associations
>> https://academic.oup.com/bib/advance-article-abstract/doi/10.1093/bib/bbaa022/5807624
SG-LSTM-FRAME generates representational features for miRNAs and genes using both sequence and geometrical information and then leveraged a deep learning method for the associations’ prediction.
SG-LSTM-FRAME predicted the top 10 miRNA-gene relationships and recommended the top 10 potential genes for hsa-miR-335-5p for SG-LSTM-core.

□ JEBIN: New gene association measures by joint network embedding of multiple gene expression datasets
>> https://www.biorxiv.org/content/10.1101/2020.03.16.992396v1.full.pdf
JEBIN (Joint Embedding of multiple BIpartite Networks) algorithm, it can learn a low-dimensional representation vector for each gene by integrating multiple bipartite networks, and each network corresponds to one dataset.
JEBIN owns many inherent advantages, such as it is a nonlinear, global model, has linear time complexity with the number of genes, dataset or samples, and can integrate datasets with different distribution.

□ dynBGP: Estimation of dynamic SNP-heritability with Bayesian Gaussian process models
>> https://academic.oup.com/bioinformatics/advance-article/doi/10.1093/bioinformatics/btaa199/5809528
dynBGP, a completely tuning-free Bayesian Gaussian process (GP) based approach for estimating dynamic variance components and heritability as their function, and a modern Markov Chain Monte Carlo (MCMC) method which allows full uncertainty quantification.
dynBGP uses data from all time points at once, making it possible for the time points to ’borrow strength’ from one another through the prior covariance structure.

□ DeepDist: real-value inter-residue distance prediction with deep residual network
>> https://www.biorxiv.org/content/10.1101/2020.03.17.995910v1.full.pdf
DeepDist, a multi-task deep learning distance predictor based on new residual convolutional network architectures to simultaneously predict real- value inter-residue distances and classify them into multiple distance intervals.
The overall performance of DeepDist’s real-value distance prediction and multi-class distance prediction is comparable according to multiple evaluation metrics. DeepDist can work well on some targets with shallow multiple sequence alignments.
□ Estimating Assembly Base Errors Using K-mer Abundance Difference (KAD) Between Short Reads and Genome Assembled Sequences
>> https://www.biorxiv.org/content/10.1101/2020.03.17.994566v1.full.pdf
a novel approach, referred to as K-mer Abundance Difference (KAD), to compare the inferred copy number of each k-mer indicated by short reads and the observed copy number in the assembly.
KAD analysis can evaluate the accuracy of nucleotide base quality at both genome-wide and single-locus levels, which, indeed, is appropriate, efficient, and powerful for assessing genome sequences assembled with inaccurate long reads.

□ RASflow: an RNA-Seq analysis workflow with Snakemake
>> https://bmcbioinformatics.biomedcentral.com/articles/10.1186/s12859-020-3433-x
RASflow, a maximally general workflow, applicable to a wide range of data and analysis approaches and at the same time support research on both model and non-model organisms.
The most time-consuming part of the whole workflow is the alignment step. pseudo alignment to a transcriptome is much faster than alignment to a genome.
□ mergeTrees: Fast tree aggregation for consensus hierarchical clustering
>> https://bmcbioinformatics.biomedcentral.com/articles/10.1186/s12859-020-3453-6
mergeTrees, a method that aggregates a set of trees with the same leaves to create a consensus tree.
In this consensus tree, a cluster at height h contains the individuals that are in the same cluster for all the trees at height h. The method is exact and proven to be O(nqlog(n)), n being the individuals and q being the number of trees to aggregate.
□ ataqv: Quantification, Dynamic Visualization, and Validation of Bias in ATAC-Seq Data
>> https://www.cell.com/cell-systems/fulltext/S2405-4712(20)30079-X
ataqv metrics may be useful as covariates in downstream analysis, in part, because they may reflect latent variables.
a robust shift toward more extreme p-values from the analysis when the covariate was included, which indicates increased statistical power after controlling for the batch effect.
ataqv calculates coverage around the TSS using entire ATAC-seq fragments, whereas other packages calculate coverage using only the cutsite or by shifting and extending individual sequencing reads such that the reads are centered on the cutsite.
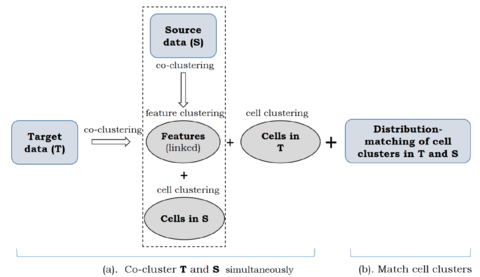
□ Coupled Co-clustering-based Unsupervised Transfer Learning for the Integrative Analysis of Single-Cell Genomic Data
>> https://www.biorxiv.org/content/10.1101/2020.03.28.013938v1.full.pdf
coupleCoC builds upon the information theoretic co-clustering framework. coupleCoC improves the overall clustering performance and matches the cell subpopulations across multimodal single-cell genomic data sets.
coupleCoC imputes each missing value in the sc-methylation data matrix with the same Bernoulli distribution with outcome: mean of non-missing values (probability p) or 0 (probability = 1 - p), where p is estimated by the frequency of non-zeros in the sc-methylation data matrix.
□ Hierarchical progressive learning of cell identities in single-cell data
>> https://www.biorxiv.org/content/10.1101/2020.03.27.010124v1.full.pdf
a hierarchical progressive learning method which automatically finds relationships between cell populations across multiple datasets and uses this to construct a hierarchical classification tree.
For each node in the tree either a linear SVM or one-class SVM, which enables the detection of unknown populations, is trained. Both the one-class and linear SVM also outperform other hierarchical classifiers.

□ EigenDel: Detecting genomic deletions from high-throughput sequence data with unsupervised learning
>> https://www.biorxiv.org/content/10.1101/2020.03.29.014696v1.full.pdf
EigenDel first takes advantage of discordant read-pairs and clipped reads to get initial deletion candidates, and then it clusters similar candidates by using unsupervised learning methods. And uses a carefully designed approach for calling true deletions from each cluster.
EigenDel uses discordant read pairs to collect deletion candidates, and it uses clipped reads to update the boundary for each of them. EigenDel first applies a read depth filter, and then it extracts four features for remaining candidates based on depth.
□ annonex2embl: automatic preparation of annotated DNA sequences for bulk submissions to ENA
>> https://academic.oup.com/bioinformatics/advance-article-abstract/doi/10.1093/bioinformatics/btaa209/5813725
annonex2embl converts an annotated DNA multi-sequence alignment (in NEXUS format) to an EMBL flatfile for submission to ENA via the Webin-CLI submission tool.
annonex2embl enables the conversion of DNA sequence alignments that are co-supplied with sequence annotations and metadata to submission-ready flatfiles.

□ CytoTalk: De novo construction of signal transduction networks using single-cell RNA-Seq data
>> https://www.biorxiv.org/content/10.1101/2020.03.29.014464v1.full.pdf
CytoTalk first constructs intracellular and intercellular gene-gene interaction networks using an information-theoretic measure between two cell types.
Candidate signal transduction pathways in the integrated network are identified using the prize-collecting Steiner forest algorithm.
□ MORFEE: a new tool for detecting and annotating single nucleotide variants creating premature ATG codons from VCF files
>> https://www.biorxiv.org/content/10.1101/2020.03.29.012054v1.full.pdf
MORFEE (Mutation on Open Reading FramE annotation) detects, annotates and predicts, from a standard VCF file, the creation of uORF by any 5’UTR variants on uORF creation.
MORFEE reads the input VCF file and use ANNOVAR (that has then to be beforehand installed) through the wrapper function vcf.annovar.annotation to annotate all variants.

□ MP-HS-DHSI: Multi-population harmony search algorithm for the detection of high-order SNP interactions
>> https://academic.oup.com/bioinformatics/advance-article-abstract/doi/10.1093/bioinformatics/btaa215/5813726
In HS, multiple criteria (Bayesian network-based K2-score, Jensen-Shannon divergence, likelihood ratio and normalized distance with joint entropy) are adopted by four harmony memories to improve the ability to discriminate diverse disease models.
the G-test statistical method and multifactor dimensionality reduction (MDR) are employed to verify the authenticity of the candidate solutions, respectively.
□ iCellR: Combined Coverage Correction and Principal Component Alignment for Batch Alignment in Single-Cell Sequencing Analysis
>> https://www.biorxiv.org/content/10.1101/2020.03.31.019109v1.full.pdf
Combined Coverage Correction Alignment (CCCA) and Combined Principal Component Alignment (CPCA).
CPCA skips the coverage correction step and uses k nearest neighbors (KNN) for aligning the PCs from the nearest neighboring cells in multiple samples.
CCCA uses a coverage correction approach (analogous to imputation) in a combined or joint fashion between multiple samples for batch alignment, while also correcting for drop-outs in a harmonious way.
□ ScaffoldGraph: an open-source library for the generation and analysis of molecular scaffold networks and scaffold trees
>> https://academic.oup.com/bioinformatics/advance-article-abstract/doi/10.1093/bioinformatics/btaa219/5814205
ScaffoldGraph (SG) is an open-source Python library and command-line tool for the generation and analysis of molecular scaffold networks and trees, with the capability of processing large sets of input molecules.
With the increase in high-throughput screening (HTS) data, scaffold graphs have proven useful for the navigation and analysis of chemical space, being used for visualisation, clustering, scaffold-diversity analysis and active-series identification.
□ A Flexible, Interpretable, and Accurate Approach for Imputing the Expression of Unmeasured Genes
>> https://www.biorxiv.org/content/10.1101/2020.03.30.016675v1.full.pdf
SampleLASSO, a sparse regression model that capture sample-sample relationships. SampleLASSO automatically leverages training samples from the same tissue. SampleLASSO is a powerful and flexible approach for harmonizing large-scale gene-expression data.
SampleLASSO outperforms all the other methods – consistently in a statistically significant manner – based on multiple error metrics, uniformly for unmeasured genes with a broad range of means and variances.

□ Recursive Convolutional Neural Networks for Epigenomics
>> https://www.biorxiv.org/content/10.1101/2020.04.02.021519v1.full.pdf
The restriction of free parameters bellow the cardinality of of the train-set making over-fitting practically impossible, and large-scale cross-domain demonstrating the proposed models tend to model generic biological phenomena rather than dataset specific correlations.
Recursive Convolutional Neural Networks (RCNN) architecture can be applied to data of an arbitrary size, and has a single meta-parameter that quantifies the models capacity, thus making it flexible for experimenting.