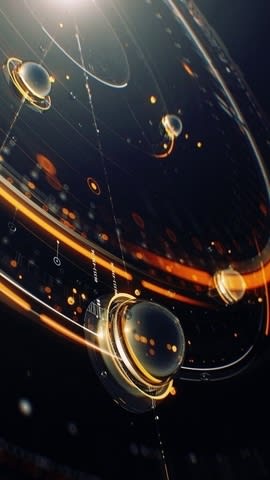

□ Amalgams: data-driven amalgamation for the reference-free dimensionality reduction of zero-laden compositional data
>> https://www.biorxiv.org/content/10.1101/2020.02.27.968677v1.full.pdf
data-driven amalgamation can outperform both PCA and principal balances as a feature reduction method for classification, and performs as well as a supervised balance selection method called selbal.
Amalgams encourages principled research into data-driven amalgamation as a tool for understanding high-dimensional compositional data, especially zero-laden count data for which standard log-ratio transforms fail.

□ CellOracle: Dissecting cell identity via network inference and in silico gene perturbation
>> https://www.biorxiv.org/content/10.1101/2020.02.17.947416v1.full.pdf
CellOracle, a computational tool that integrates single-cell transcriptome and epigenome profiles, integrating prior biological knowledge via regulatory sequence analysis to infer GRNs.
CellOracle against ground-truth TF-gene interactions and demonstrate its efficacy to recapitulate known regulatory changes across hematopoiesis, correctly predicting well-characterized phenotypic changes in response to TF perturbations.
Application of CellOracle to direct lineage reprogramming reveals distinct network configurations underlying different modes of reprogramming failure. GRN reconfiguration along successful cell fate conversion trajectories identifies new factors to enhance target cell yield.
□ Variance-adjusted Mahalanobis (VAM): a fast and accurate method for cell-specific gene set scoring
>> https://www.biorxiv.org/content/10.1101/2020.02.18.954321v1.full.pdf
Variance-adjusted Mahalanobis (VAM), that seamlessly integrates with the Seurat framework and is designed to accommodate the technical noise, sparsity and large sample sizes characteristic of scRNA-seq data.
The VAM method generates cell-specific gene set scores from scRNA-seq data using a variation of the classic Mahalanobis multivariate distance measure, and computes cell-specific pathway scores to transform a cell-by-gene matrix into a cell-by-pathway matrix.


□ Poincaré maps: Hyperbolic embeddings to understand how cells develop
>> https://ai.facebook.com/blog/poincare-maps-hyperbolic-embeddings-to-understand-how-cells-develop/
Poincaré maps, a method that harness the power of hyperbolic geometry into the realm of single-cell data analysis.
the Riemannian structure of hyperbolic manifolds enables the use of gradient-based optimization methods what is essential to compute embeddings of large-scale measurements.
To leverage the Poincaré disk as an embedding space, employing pairwise distances obtained from a nearest-neighbor graph as a learning signal to construct hyperbolic embeddings for the discovery of complex hierarchies in data.
Poincaré maps enables direct exploratory analysis and the use of its embeddings in a wide variety of downstream data analysis tasks, such as visualization, clustering, lineage detection and pseudo-time inference.

□ DR-A: A deep adversarial variational autoencoder model for dimensionality reduction in single-cell RNA sequencing analysis
>> https://bmcbioinformatics.biomedcentral.com/articles/10.1186/s12859-020-3401-5
DR-A (Dimensionality Reduction with Adversarial variational autoencoder), novel GAN-based architecture, to fulfill the task of dimensionality reduction. DR-A leverages a novel adversarial variational autoencoder-based framework, a variant of generative adversarial networks.
DR-A integrates the AVAE-DM structure with the Bhattacharyya distance. The novel architecture of an Adversarial Variational AutoEncoder with Dual Matching (AVAE-DM). An autoencoder (a deep encoder and a deep decoder) reconstructs the scRNA-seq data from a latent code vector z.
□ BioTracs: A transversal framework for computational workflow standardization and traceability
>> https://www.biorxiv.org/content/10.1101/2020.02.16.951624v1.full.pdf
BioTracs, a transversal framework for computational workflow standardization and traceability. It is based on PRISM architecture (Process Resource Interfacing SysteM), an agnostic open architecture.
As an implementation of the PRISM architecture, BioTracs paved the way to an open framework in which bioinformatics could specify ad model workflows. PRISM architecture is designed to provide scalability and transparency from the code to the project level.

□ scTSSR: gene expression recovery for single-cell RNA sequencing using two-side sparse self-representation
>> https://academic.oup.com/bioinformatics/advance-article/doi/10.1093/bioinformatics/btaa108/5740568
scTSSR simultaneously learns two non-negative sparse self-representation matrices to capture the gene-to-gene and cell-to-cell similarities. scTSSR has a competitive performance in recovering the true expression levels.
scTSSR takes advantage of the whole expression matrix. scTSSR does not impose a very strong assumption on the underlying data, and can be applied to data including both discrete cell clusters and continuous trajectories.

□ Deep learning of dynamical attractors from time series measurements
>> https://arxiv.org/abs/2002.05909
the inverse problem: given a single, time-resolved measurement of a complex dynamical system, is it possible to reconstruct the higher-dimensional process driving the dynamics?
This process, known as state space reconstruction, is the focus of many classical results in nonlinear dynamics theory, which has demonstrated various heuristics for reconstructing effective coordinates from the time history of the system.
a custom loss function and regularizer for autoencoders, the false-nearest-neighbor loss, that allows multiple autoencoder architectures to successfully reconstruct unseen dynamical variables from univariate time series.

□ npGraph: Real-time resolution of short-read assembly graph using ONT long reads
>> https://www.biorxiv.org/content/10.1101/2020.02.17.953539v1.full.pdf
npGraph, a streaming hybrid assembly tool using the assembly graph instead of the separated pre-assembly contigs. It is able to produce more complete genome assembly by resolving the path finding problem on the assembly graph using long reads as the traversing guide.
npGraph uses the stream of long reads to untangle knots in the assembly graph, which is maintained in memory. Because of this, npGraph has better estimation of multiplicity of repeat contigs, resulting in fewer misassemblies.
This strategy allows npGraph to progressively update the likelihood of the paths going through a knot. To align the long reads to the assembly graph components, both BWA-MEM or minimap2 were used in conjunction with npGraph.
□ SSIF: Subsumption-based Sub-term Inference Framework to Audit Gene Ontology
>> https://academic.oup.com/bioinformatics/advance-article/doi/10.1093/bioinformatics/btaa106/5739437
Used to audit Gene Ontology (GO) by leveraging its underlying graph structure and a novel term-algebra.
The formulation of algebraic operations for the development of a term-algebra based on this sequence-based representation, using antonyms and subsumption-based longest subsequence alignment.
The construction of a set of conditional rules (similar to default rules) for backward subsumption inference aimed at uncovering semantic inconsistencies in GO and other ontological structures.
□ Joint variable selection and network modeling for detecting eQTLs
>> https://www.degruyter.com/view/j/sagmb.ahead-of-print/sagmb-2019-0032/sagmb-2019-0032.xml
eveluate the performance of MSSL – Multivariate Spike and Slab Lasso, SSUR – Sparse Seemingly Unrelated Bayesian Regression, and OBFBF – Objective Bayes Fractional Bayes Factor, along with the proposed, JDAG (Joint estimation via a Gaussian Directed Acyclic Graph model) method.
The computation cost for SSUR is extremely high, while MSSL requires relatively short time for execution. Compared with JDAG which is designed for small to moderate datasets, SSUR and MSSL are shown to be working under larger dimensions.
□ SPsimSeq: semi-parametric simulation of bulk and single cell RNA sequencing data
>> https://academic.oup.com/bioinformatics/advance-article/doi/10.1093/bioinformatics/btaa105/5739438
SPsimSeq uses a specially designed exponential family for density estimation to constructs the distribution of gene expression levels, and simulates a new dataset from the estimated marginal distributions using Gaussian-copulas to retain the dependence between genes.
the logarithmic counts per millions of reads (log-CPM) values from a given real dataset are used for semi-parametrically estimating gene-wise distributions and the between- genes correlation structure.
□ sbVAE: Decision-Making with Auto-Encoding Variational Bayes
>> https://arxiv.org/pdf/2002.07217.pdf
fitting the scVI model with the standard VAE procedure as well as all three sbVAE algorithms. AIS is used to approximate the posterior distribution once the model is fitted. This includes the VAE + AIS and the M-sbVAE + AIS baselines.
using alternating minimization, and choose the variational distribution that minimizes an upper bound on the log evidence, equivalent to minimizing either the forward Kullback-Leibler, or the χ2 divergence, instead of the reverse KL divergence.

□ scCATCH: Automatic Annotation on Cell Types of Clusters from Single-Cell RNA Sequencing Data
>> https://www.cell.com/iscience/fulltext/S2589-0042(20)30066-3
scCATCH a single cell Cluster-based Annotation Toolkit for Cellular Heterogeneity from cluster marker genes identification to cluster annotation based on evidence-based score by matching the identified potential marker genes with known cell markers in CellMatch database.
the superiority of scCATCH over other methods of identifying marker genes, including Seurat, the cell-based annotation method CellAssign, Garnett, SingleR, scMap, and CHETAH, through three scRNA-seq validation datasets.
□ nanoMLST: accurate multilocus sequence typing using Oxford Nanopore Technologies MinION with a dual-barcode approach to multiplex large numbers of samples
>> https://www.microbiologyresearch.org/content/journal/mgen/10.1099/mgen.0.000336
The nanopore reads were polished iteratively with Racon and Nanopolish to generate consensus sequences, the accuracy of the consensus sequences was higher than 99.8 %, but there were homopolymer errors in consensus sequences notwithstanding.
implementing dual-barcode demultiplexing effectively using Minimap2 and this custom scripts. the demultiplexed reads for each sample were successfully polished and corrected to produce accurate STs, which would make our MLST approach a rapid way for molecular typing.

□ CMF-Impute: An accurate imputation tool for single cell RNA-seq data
>> https://academic.oup.com/bioinformatics/advance-article/doi/10.1093/bioinformatics/btaa109/5740569
CMF-Impute, a novel collaborative matrix factorization-based method to impute the dropout entries of a given scRNA-seq expression matrix.
CMF-Impute achieves the most accurate cell classification results in spite of the choice of different clustering methods like SC3 or t-SNE followed by K-means as evaluated by both adjusted rand index (ARI) and normalized mutual information (NMI).
CMF-Impute outperforms other methods in imputing to the original expression values as evaluated by both the sum of squared error (SSE) and Pearson correlation coefficient, and reconstructs cell-to-cell and gene-to-gene correlation, and in inferring cell lineage trajectories.
□ IPCO: Inference of Pathways from Co-variance analysis
>> https://bmcbioinformatics.biomedcentral.com/articles/10.1186/s12859-020-3404-2
IPCO utilises the biological co-variance observed between paired taxonomic and functional profiles and co-varies it with the queried dataset.
The references provided in IPCO are generated with UniRef90 database and the largest and manually curated MetaCyc mapping file provided along with HUMAnN2.
□ BEES: Bayesian Ensemble Estimation from SAS
>> https://www.cell.com/biophysj/fulltext/S0006-3495(19)30513-2
a Bayesian-based method for fitting ensembles of model structures to experimental SAS data that rigorously avoids overfitting.
BEES fits routine allows for secondary data sets to be supplied, thereby simultaneously fitting models to both SAS data as well as orthogonal information.
□ IHS: an integrative method for the identification of network hubs
>> https://www.biorxiv.org/content/10.1101/2020.02.17.953430v1.full.pdf
integration of the most important centrality measures that capture all topological dimensions of a network and synergizing their impacts could be a big step towards identification of the most influential nodes.
IHS is an unsupervised method that generates the synergistic product of the most important local, semi-local, & global centrality measures in a way that simultaneously removes the positional bias of betweenness centrality for the identification of hub nodes in the whole network.

□ HiFi Assembler Series, Part 2: HiCanu, near optimal repeat resolution using HiFi reads
>> https://medium.com/@Magdoll/hifi-assembler-series-part-2-hicanu-near-optimal-repeat-resolution-using-hifi-reads-412728ed167f
HiCanu is the latest member in the Canu assembler family that utilizes long read data. Based on the Celera Assembler, the original Canu was modified to work with long reads that had higher error rates by adapting a weighted MinHash-based overlapper with a sparse assembly graph.
Applying HiCanu to three human HiFI datasets (CHM13, HG0733, and NA12878) resulted in the fewest number of errors against the reference compared to Peregrine assemblies with HiFi, Canu assemblies with ONT, and 10X supernova assemblies.
□ Bonito A convolutional basecaller inspired by QuartzNet
> https://github.com/nanoporetech/bonito
□ Interpreting Deep Neural Networks Beyond Attribution Methods: Quantifying Global Importance of Genomic Features
>> https://www.biorxiv.org/content/10.1101/2020.02.19.956896v1.full.pdf
the causal effect of a specific sequence pattern w/ respect to a given molecular phenotype can be estimated by measuring the phenotypic outcome of sequences designed to contain a fixed, known pattern and randomizing the within the sequences as well as the intervention assignment.

□ Tree-SNE: Hierarchical Clustering and Visualization Using t-SNE
>> https://arxiv.org/pdf/2002.05687.pdf
Building on recent advances in speeding up t-SNE and obtaining finer-grained structure, combine the two to create tree-SNE, a hierarchical clustering and visualization algorithm based on stacked one-dimensional t-SNE embeddings.
Alpha-clustering, which recommends the optimal cluster assignment, without foreknowledge of the number of clusters, based off of the cluster stability across multiple scales.
□ HMW gDNA purification and ONT ultra-long-read data generation
>> https://www.protocols.io/view/hmw-gdna-purification-and-ont-ultra-long-read-data-bchhit36

□ FC-R2: Recounting the FANTOM Cage Associated Transcriptome
>> https://genome.cshlp.org/content/early/2020/02/20/gr.254656.119.full.pdf
FANTOM-CAT/recount2, FC-R2 is a comprehensive expression atlas across a broadly defined human transcriptome, inclusive of over 109,000 coding and non-coding genes, as described in the FANTOM-CAT. This atlas greatly extends the gene annotation used in the recount2 resource.
Overall, all analyzed tissue specific markers presented nearly identical expression profiles across GTEx tissue types between the alternative gene models considered, confirming the consistency between gene expression quantification in FC-R2 and those based on GENCODE.
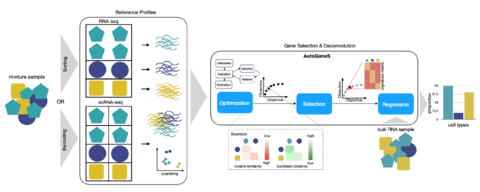
□ AutoGeneS: Automatic gene selection using multi-objective optimization for RNA-seq deconvolution
>> https://www.biorxiv.org/content/10.1101/2020.02.21.940650v1.full.pdf
AutoGeneS requires no prior knowledge about marker genes and selects genes by simultaneously optimizing multiple criteria: minimizing the correlation and maximizing the distance between cell types.
For a multi-objective optimization problem, there usually exists no single solution that simultaneously optimizes all objectives. In this case, the objective functions are said to be conflicting, and there exists a (possibly infinite) number of Pareto-optimal solutions.
□ Δ-dN/dS: New Criteria to Distinguish among Different Selection Modes in Gene Evolution
>> https://www.biorxiv.org/content/10.1101/2020.02.21.960450v1.full.pdf
the observation of dN/dS<1 can be explained by either the existence of essential sites plus some sites under (weak) positive selection, or the existence of essential sites in the dominance of strictly neutral evolution, or the nearly nearly-neutral evolution.
Under the context of strong purifying selection at some amino acid sites, this model predicts that dN/dS=1-H for the neutral evolution, dN/dS<1-H for the nearly-neutral selection, and dN/dS>1-H for the adaptive evolution.
□ MONET: Multi-omic patient module detection by omic selection
>> https://www.biorxiv.org/content/10.1101/2020.02.21.960062v1.full.pdf
MONET (Multi Omic clustering by Non-Exhaustive Types) uses ideas from Matisse , an algorithm to detect gene modules, and generalizes its algorithmic approach to multi-omic data.
Monet's solution can be used to create for every sample and module a score for the linking of the sample to that module: the sum of weights between the sample and all the module's samples across all omics covered by the module.
Monet can detect common structure across omics when it is present, but can also disregard omics with a different structure. The optimization problem Monet solves is NP- hard, so the algorithm is heuristic.
□ Nubeam-dedup: a fast and RAM-efficient tool to de-duplicate sequencing reads without mapping
>> https://academic.oup.com/bioinformatics/advance-article/doi/10.1093/bioinformatics/btaa112/5753947
Nubeam-dedup is based on the Nubeam, which represents nucleotides by matrices, transforms reads into products of matrices, and based on which assigns a unique number to a read.
With different reads assigned different numbers, Nubeam provides a perfect hash function for DNA sequences, which enables fast and RAM-efficient de-duplication.
□ Dynamics as a cause for the nanoscale organization of the genome
>> https://www.biorxiv.org/content/10.1101/2020.02.24.963470v1.full.pdf
it is not possible to directly analyze which molecular constituents of the system are involved and which pathway is responsible for the observed causality.
The framework represents a first step towards a more extensive inference of causal relationships in the highly complex context of chromatin in space and time.
exploring whether causal relationships exist between parameters characterizing the chromatin blob dynamics and structure, by adapting a framework for spatio-temporal Granger-causality inference using Deep-PALM.
□ Pair consensus decoding improves accuracy of neural network basecallers for nanopore sequencing
>> https://www.biorxiv.org/content/10.1101/2020.02.25.956771v1.full.pdf
a general approach for improving accuracy of 1D2 and related protocols by finding the consensus of two neural network basecallers, by combining a constrained profile- profile alignment with a heuristic variant of beam search.
PoreOver implements a CTC-style recurrent neural network basecaller and associated CTC decoding algorithms. Using the neural network output from PoreOver, our consensus algorithm yields a median 6% improvement in accuracy on a test set of 1D2 data.
a beam search decoding algorithm for the pair decoding of two reads, making use of a constrained dynamic programming alignment envelope heuristic to speed calculations by focusing on areas of each read which are likely to represent the same sequence.
□ PgmGRNs: A Probabilistic Graphical Model for System-Wide Analysis of Gene Regulatory Networks
>> https://academic.oup.com/bioinformatics/advance-article-abstract/doi/10.1093/bioinformatics/btaa122/5756207
PgmGRNs combines the formulation of probabilistic graphical modeling, standard statistical estimation, and integration of high-throughput biological data to explore the global behavior of biological systems and the global consistency between experimentally verified GRNs.
The model is represented as a probabilistic bipartite graph, which can handle highly complex network systems and accommodates partial measurements of diverse biological entities and various stimulators participating in regulatory networks.
□ MUM&Co: Accurate detection of all SV types through whole genome alignment
>> https://academic.oup.com/bioinformatics/advance-article-abstract/doi/10.1093/bioinformatics/btaa115/5756209
MUM&Co is a single bash script to detect Structural Variations utilizing Whole Genome Alignment. Using MUMmer’s nucmer alignment, MUM&Co can detect insertions, deletions, tandem duplications, inversions and translocations greater than 50bp.
Its versatility depends upon the WGA and therefore benefits from contiguous de-novo assemblies generated by 3rd generation sequencing technologies. Benchmarked against 5 WGA SV-calling tools.
□ SDip: A novel graph-based approach to haplotype-aware assembly based structural variant calling in targeted segmental duplications sequencing
>> https://www.biorxiv.org/content/10.1101/2020.02.25.964445v1.full.pdf
SDip is a novel graph-based approach that leverages single nucleotide differences in overlapping reads to distinguish allelic and duplication sequences information from long read accurate PacBio HiFi sequencing.
These differences enable to generate allelic and duplication-specific overlaps in the graph to spell out phased assembly used for structural variant calling. SDip produced SV call sets in complex segmental duplications that have potential applications in evolutionary genomics.

□ Mustache: Multi-scale Detection of Chromatin Loops from Hi-C and Micro-C Maps using Scale-Space Representation
>> https://www.biorxiv.org/content/10.1101/2020.02.24.963579v1.full.pdf
Mustache detects loops at a wide range of genomic distances, identifying potential structural and regulatory interactions that are supported by independent conformation capture experiments as well as by known correlates of loop formation such as CTCF binding, enhancers and promoters.
Mustache is a new loop caller for multi-scale detection of chromatin loops from Hi-C and Micro-C contact maps. Mustache uses recent technical advances in scale-space theory in Computer Vision to detect chromatin loops caused by interaction of DNA segments with a variable size.
□ MGI Deconstructs the Sequencer
>> http://omicsomics.blogspot.com/2020/02/mgi-deconstructs-sequencer.html
$100 genome via 700 genomes per run on radical rethink of sequencer architecture.

□ ELSA: Ensemble learning for classifying single-cell data and projection across reference atlases
>> https://academic.oup.com/bioinformatics/advance-article-abstract/doi/10.1093/bioinformatics/btaa137/5762611
ELSA, a boosted learner that overcomes the greatest challenge with status quo classifiers: low sensitivity, especially when dealing with rare cell types.
ELSA uses the RandomForestClassifier package in Sklearn to optimize feature selection for classification. ELSA bootstrap resamples the input training data, choosing samples uniformly and at random with replacement.
□ brt: An R package for integrating biological relevance with p value in omics data
>> https://www.biorxiv.org/content/10.1101/2020.02.27.968909v1.full.pdf
Analyses of large-scale -omics datasets commonly use p-values as the indicators of statistical significance. However, considering p-value alone neglects the importance of effect size in determining the biological relevance of a significant difference.
a new procedure, biological relevance testing (BRT), to address this problem of testing for biological relevance. the BRT procedure integrates the effect size information by averaging the effect among a set of related null hypotheses.