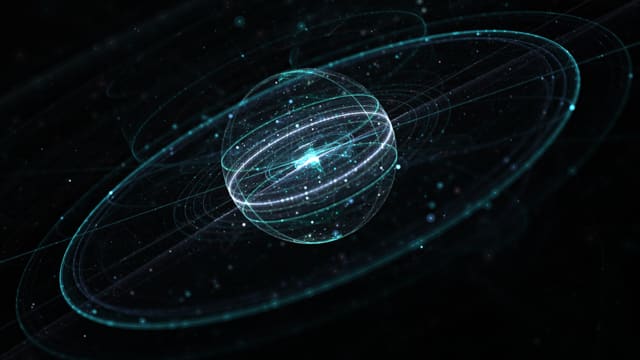

□ Genomic data integration systematically biases interactome mapping:
>> https://journals.plos.org/ploscompbiol/article?id=10.1371/journal.pcbi.1006474
the genomic data integration leads to an increase in the functional coherence of the resulting interactome maps, but this comes at the expense of a decrease in power to discover novel interactions. mRNA co-expression, phylogenetic profiling, & domain-domain interactions are among the most appropriate features for this purpose, as they yield significant increases in functional connectivity at the expense of relatively modest increases in the proportion of novel interactions.

□ Applying non-parametric testing to discrete transfer entropy:
>> https://www.biorxiv.org/content/biorxiv/early/2018/11/02/460733.full.pdf
a novel Markov-chain Monte Carlo method of detecting significance from Transfer Entropy, by sampling surrogate & experimental values. Non-parametric statistical test, Mann-Whitney U-test, is then applied to determine the significance of each hypothesis regarding the connectivity. Transfer Entropy also provides insights into the organization of information flow within this biological network, providing a view into development and identifying the emergence of hubs.

□ RUBRIC: Real-Time Selective Sequencing with Read Until with Basecall and Reference-Informed Criteria:
>> https://www.biorxiv.org/content/biorxiv/early/2018/11/02/460014.full.pdf
RUBRIC method is the first example of real-time selective sequencing where on-line basecalling enables alignment against conventional nucleic acid references to provide the basis for sequence/reject decisions. Built upon the original Read Until sample code provided by Loose, RUBRIC integrates ONT’s Nanonet basecaller (v2.0.0, included with the RUBRIC code) and replaces Dynamic Time Warping (DTW) -based target pattern-matching with sequence-based alignment using LAST (rev 759).
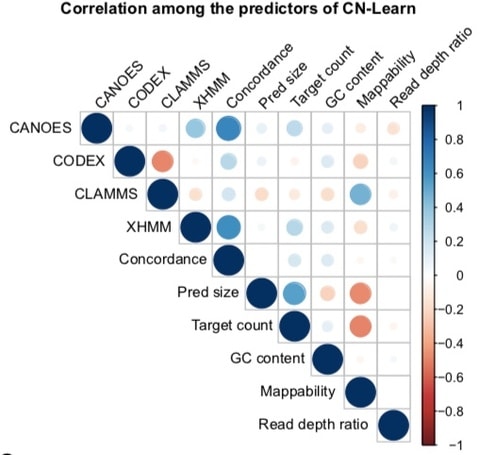
□ CN-Learn: A machine-learning approach for accurate detection of copy-number variants from exome sequencing:
>> https://www.biorxiv.org/content/biorxiv/early/2018/11/02/460931.full.pdf
CN-Learn leverages the extent of concordance among multiple method as well as additional genomic contexts such as GC content, mappability and local read depth fluctuations. Using this exhaustive set of predictors extracted for CNVs from a “gold-standard” CNV call set (CANOES, Codex, CLAMMS, XHMM), CN-Learn builds a ‘Random Forest’ classifier with hundreds of decision trees to estimate the probability of each CNV in a fresh set of samples being true.
□ Wtdbg2: a de novo sequence assembler for long noisy reads produced by PacBio or Oxford Nanopore Technologies:
>> https://github.com/ruanjue/wtdbg2/blob/master/README.md
Wtdbg2 is able to assemble the human and even the 32Gb Axolotl genome at a speed tens of times faster than CANU and FALCON while producing contigs of comparable base accuracy.
wtdgb2 assembles raw reads without error correction and then builds the consensus from intermediate assembly output. During assembly, wtdbg2 chops reads into 1024bp segments, merges similar segments into a vertex and connects vertices based on the segment adjacency on reads. The resulting graph is called fuzzy Bruijn graph (FBG). It is akin to De Bruijn graph but permits mismatches/gaps and keeps read paths when collapsing k-mers. The use of FBG distinguishes wtdbg2 from the majority of long-read assemblers.

□ PhyCLIP: Phylogenetic Clustering by Linear Integer Programming:
>> https://www.biorxiv.org/content/biorxiv/early/2018/10/23/446716.1.full.pdf
Phylogenetic Clustering by Linear Integer Programming (PhyCLIP) was developed to provide a statistically-principled phylogenetic clustering framework that negates the need for an arbitrarily-defined distance threshold. Using the pairwise patristic distance distributions of an input phylogeny, PhyCLIP parameterises the intra- and inter-cluster divergence limits as statistical bounds in an integer linear programming model which is subsequently optimised to cluster as many sequences as possible.


□ Modular modeling improves the predictions of genetic variant effects on splicing
>> https://www.biorxiv.org/content/early/2018/10/10/438986
The MMSplice modules are neural networks scoring exon, intron, and splice sites, trained on distinct large-scale genomics datasets. leveraging a massively parallel reporter assay to build individual modules. Also, models predicting Ψ and splicing efficiencies were trained on large-scale perturbation datasets (Vex-seq and MaPSy). Sequence-based model trained from perturbation assays can learn causal features.
In contrast, predictive models trained on variations across the reference genome or across natural genetic variations in the population may be limited by evolutionary confounding factors, limiting the model’s ability to make causal predictions about genetic variants.
□ scGESTALT: Large-scale reconstruction of cell lineages using single-cell readout of transcriptomes and CRISPR–Cas9 barcodes:
>> https://www.nature.com/articles/s41596-018-0058-x
scGESTALT provides a scalable platform to map lineage relationships between cell types in any system that permits genome editing during development, regeneration, or disease.

□ Clust: automatic extraction of optimal co-expressed gene clusters from gene expression data:
>> https://genomebiology.biomedcentral.com/articles/10.1186/s13059-018-1536-8
Clust is designed to extract co-expressed clusters of genes that satisfy the biological expectations of a co-expressed gene cluster, even if these datasets have different properties such as numbers of conditions or replicates. Clust is composed of 4 major steps: data pre-processing of the one or more input raw datasets, production of a pool of seed clusters, cluster evaluation & the selection of a subset of elite seed clusters, and the optimization of the elite seed clusters to produce final clusters.

□ Single cell RNA-seq data clustering using TF-IDF (Term Frequency - Inverse Document Frequency transformation) based methods:
>> https://bmcgenomics.biomedcentral.com/articles/10.1186/s12864-018-4922-4
Overall, algorithms using TF-IDF binarization have consistently high accuracy in all 2-class experiments, with existing methods and algorithms using TF-IDF based gene selection showing a higher degree of variability in accuracy across datasets. A limitation of the TF-IDF_Bin_HC methods’ group is the quadratic time required for distance calculations used in hierarchical clustering methods, which becomes a performance bottleneck for datasets with millions of single cells.

□ novoSpaRc: Charting a tissue from single-cell transcriptomes:
>> https://www.biorxiv.org/content/biorxiv/early/2018/10/30/456350.full.pdf
novoSpaRc (de novo Spatial Reconstruction) is a computational frame-
work that enables the spatial reconstruction of single-cell gene expression de novo, w/ no inherent reliance on an existing reference atlas and the flexibility to introduce prior information when it does exist. novoSpaRc using an efficient algorithm for the optimization problem (Methods) inspired by the combined results for entropically regularized optimal transport and Gromov-Wasserstein distance-based mapping between metric-measure spaces.

□ scMetric: An R package of metric learning and visualization for single-cell RNA- seq data:
>> https://www.biorxiv.org/content/biorxiv/early/2018/10/30/456814.full.pdf
scMetric outputs the t-SNE maps using the learned metric and using the conventional Euclidean distance metric. The learned metric as well as the key genes that compose most weights in the metric can also be output to other analysis methods that need a distance/similarity metric.
□ Octopus: A unified haplotype-based method for accurate and comprehensive variant calling:
>> https://www.biorxiv.org/content/biorxiv/early/2018/10/29/456103.full.pdf
Octopus is a probabilistic phasing algorithm that leverages both prior and read information, and can phase genotypes with arbitrary ploidy, including those that contain somatic mutations. Octopus takes inspiration from particle filtering by constructing a tree of haplotypes and dynamically pruning and extending the tree based on haplotype posterior probabilities in a sequential manner.
□ The Markov link method: a nonparametric approach to combine observations from multiple experiments:
>> https://www.biorxiv.org/content/biorxiv/early/2018/10/30/457283.full.pdf
The MLM makes a conditional independence assumption that holds in many multi-measurement contexts and provides a a way to estimate the link, the conditional probability of one type of measurement given the other. Some of this literature also comes from the field of causality. The Clauser-Horne-Shimony-Holt inequality was designed to help answer causality questions in quantum physics, but it also sheds light on what distributions are consistent with certain assumptions.
□ DUETT quantitatively identifies known and novel events in nascent RNA structural dynamics from chemical probing data:
>> https://www.biorxiv.org/content/biorxiv/early/2018/11/01/458703.full.pdf
□ Gkmexplain: Fast and Accurate Interpretation of Nonlinear Gapped k-mer Support Vector Machines Using Integrated Gradients:
>> https://www.biorxiv.org/content/biorxiv/early/2018/11/01/457606.full.pdf
gkmexplain, an algorithm based on Integrated Gradients that can be used to efficiently explain the predictions of a Support Vector Machine trained with various gapped k-mer string kernels. Using simulated regulatory DNA sequences, gkmexplain identifies predictive patterns with high accuracy while avoiding pitfalls of deltaSVM and ISM and being orders of magnitude more computationally efficient than SHAP.
□ Aneuvis: Web-based exploration of numerical chromosomal variation in single cells:
>> https://www.biorxiv.org/content/biorxiv/early/2018/11/01/459735.full.pdf
Aneuvis operates downstream of existing experimental and computational approaches that generate a matrix containing the estimated chromosomal copy number per cell.
□ Illumina is acquiring PacBio for $1.2 billion: That's $8 per share in cash, a 71% premium to PacBio's 30-day share-price average as of Oct. 31.
>> https://www.genomeweb.com/sequencing/illumina-acquire-pacific-biosciences-12-billion
Illumina said that PacBio's long-read sequencing technology will complement its own short-read sequencing platforms and will allow it to provide integrated workflows and innovations that bring together the best of both technologies.

□ Graphlet Laplacians: graphlet-based neighbourhoods highlight topology-function and topology-disease relationships:
>> https://www.biorxiv.org/content/biorxiv/early/2018/11/04/460964.full.pdf
In biological networks, clusters obtained by using different Graphlet Laplacians capture complementary sets of biological functions. The accuracy of Graphlet Laplacian based predictions slightly outperform Vicus. k-path Laplacian based diffusion on the human PPI network added no additional driver gene information compared to the standard Laplacian.
□ Harmony: Fast, sensitive, and flexible integration of single cell data
>> https://www.biorxiv.org/content/biorxiv/early/2018/11/04/461954.full.pdf
for small inter-datasets differences, cells from multiple datasets cluster together without the penalty. In this case, Harmony still integrates the cells during the linear correction phase. One could imagine that with an infinitely large penalty hyperparameter, Harmony would overmix datasets during clustering and hence overcorrect the data.

□ BELLA: Berkeley Efficient Long-Read to Long-Read Aligner and Overlapper:
>> https://www.biorxiv.org/content/biorxiv/early/2018/11/07/464420.full.pdf
BELLA, which implement a novel approach to compute overlaps using Sparse Generalized Matrix Multiplication. BELLA’s overlap detection has been coupled with a seed-and-extend banded-alignment method. a probabilistic model which demonstrates the soundness of using short, fixed length k-mers to detect overlaps, avoiding expensive pairwise alignment of all reads against all others.
□ GRISLI: Gene regulation inference from single-cell RNA-seq data with linear differential equations and velocity inference:
>> https://www.biorxiv.org/content/biorxiv/early/2018/11/07/464479.full.pdf
GRISLI infers a velocity vector field in the space of scRNA-seq data from profiles of individual data, and models the dynamics of cell trajectories with a linear ordinary differential equation to reconstruct the underlying GRN with a sparse regression procedure. The input to GRISLI is a set of time-stamped scRNA-seq data (xi,ti)i=1,...,C, where C is the number of cells, xi is the vector of GE for the i-th cell and ti is the time associated to the i-th cell; this time can be based on the real experimental time / calculated pseudo-time.
□ Vec2SPARQL: integrating SPARQL queries and knowledge graph embeddings:
>> https://www.biorxiv.org/content/biorxiv/early/2018/11/07/463778.full.pdf
the vector space feature representations generated from deep learning models can also be queried using functions that perform vector operations and may have some defined semantics. demonstrate using biomedical, clinical, and bioinformatics use cases how this approach can enable new kinds of queries and applications that combine symbolic processing and retrieval of information through sub-symbolic semantic queries within vector spaces.
□ Capturing Multicellular System Designs Using the Synthetic Biology Open Language (SBOL):
>> https://www.biorxiv.org/content/biorxiv/early/2018/11/07/463844.full.pdf
Automated design software could combine cell designs into a multicellular system and automatically determine the nature of any interactions between cells.
□ OrthoFinder2: fast and accurate phylogenomic orthology analysis from gene sequences:
>> https://www.biorxiv.org/content/biorxiv/early/2018/11/08/466201.full.pdf
OrthoFinder uses a novel, fast and scalable duplication-loss-coalescent resolution algorithm to identify gene duplication events and map them to the species tree.
□ duphold: scalalable, depth-based annotation and curation of high-confidence structural variant calls:
>> https://www.biorxiv.org/content/biorxiv/early/2018/11/08/465385.full.pdf
duphold algorithm is fully detailed in the mosdepth manuscript. Once the coverage array is filled, all remaining steps are independent of the number of alignments. Owing to the speed of in-memory array operations, subsequent depth calculations are nearly independent of the number of variants annotated in the VCF file.
□ TALON:
>> https://github.com/dewyman/TALON
TALON identifies known and novel genes/isoforms in long read transcriptome data sets. TALON is technology-agnostic in that it works from mapped SAM files, allowing data from different sequencing platforms (i.e. PacBio and Oxford Nanopore) to be analyzed side by side.
□ Building an automated bioinformatician: more accurate, large-scale genomic discovery using parameter advising:
>> https://www.dandeblasio.com/papers/talk_biodata18.pdf
The major contributions of this work are twofold: first, the first time that sets of alternative parameter vectors in certain domains can be found using methods other than exhaustive enumeration; and second, take some of the first steps towards producing a fully automated genomic analysis pipeline by automating sample-specific parameter selection.
□ Nanopore native RNA sequencing of a human poly(A) transcriptome
>> https://www.biorxiv.org/content/biorxiv/early/2018/11/09/459529.full.pdf
The study focused on poly(A) RNA from the human cell line GM12878, generating 9.9 million aligned sequence reads. These native RNA reads had an aligned N50 length of 1294 bases, and a maximum aligned length of over 21,000 bases. A total of 78,199 high-confidence isoforms were identified by combining long nanopore reads with short higher accuracy Illumina reads.