
(Art by JT DiMartile)


□ HyperG-VAE: Inferring gene regulatory networks by hypergraph variational autoencoder
>> https://www.biorxiv.org/content/10.1101/2024.04.01.586509v1
Hypergraph Variational Autoencoder (HyperG-VAE), a Bayesian deep generative model to process the hypergraph data. HyperG-VAE simultaneously captures cellular heterogeneity and gene modules through its cell and gene encoders individually during the GRNs construction.
HyperG-VAE employs a cell encoder with a Structural Equation Model to address cellular heterogeneity. The cell encoder within HyperG-VAE predicts the GRNs through a structural equation model while also pinpointing unique cell clusters and tracing the developmental lineage.

□ gLM: Genomic language model predicts protein co-regulation and function
>> https://www.nature.com/articles/s41467-024-46947-9
gLM (genomic language model) learns contextual representations of genes. gLM leverages pLM embeddings as input, which encode relational properties and structure information of the gene products.
gLM is based on the transformer architecture and is trained using millions of unlabelled metagenomic sequences, w/ the hypothesis that its ability to attend to different parts of a multi-gene sequence will result in the learning of gene functional semantics and regulatory syntax.

□ scDAC: deep adaptive clustering of single-cell transcriptomic data with coupled autoencoder and dirichlet process mixture model
>> https://academic.oup.com/bioinformatics/advance-article/doi/10.1093/bioinformatics/btae198/7644284
scDAC, a deep adaptive clustering method based on coupled Autoencoder (AE) and Dirichlet Process Mixture Model (DPMM). scDAC takes advantage of the AE module to be scalable, and takes advantage of the DPMM module to cluster adaptively without ignoring rare cell types.
The number of predicted clusters increased as parameter increased, which is consistent with the meaning of the Dirichlet process model. scDAC can obtain accurate numbers of clusters despite the wide variation of the hyperparameter.

□ Free Energy Calculations using Smooth Basin Classification
>> https://arxiv.org/abs/2404.03777
Smooth Basin Classification (SBC); a universal method to construct collective variables (CVs). The CV is a function of the atomic coordinates and should naturally discriminate between initial and final state without violating the physical symmetries in the system.
SBC builds upon the successful development of graph neural networks (GNNs) as effective interatomic potentials by using their learned feature space as ansatz for constructing physically meaningful CVs.
SBC exploits the intrinsic overlap that exists between a quantitative understanding of atomic interactions and free energy minima. Its training data consists of atomic geometries which are labeled with their corresponding basin of attraction.
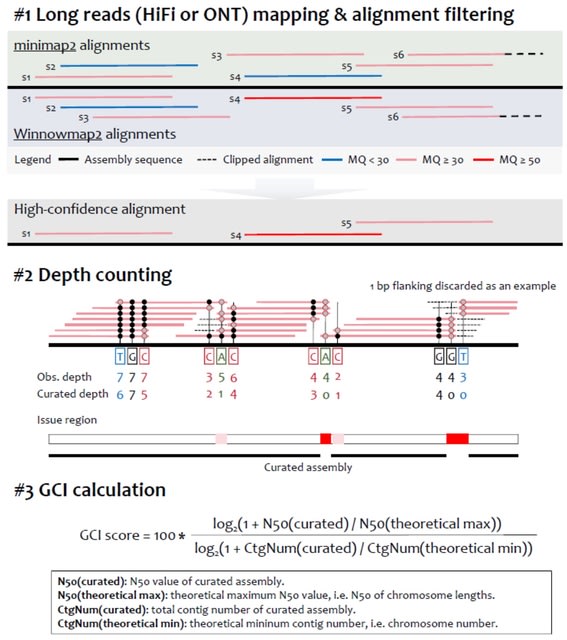
□ GCI: Genome Continuity Inspector for complete genome assembly
>> https://www.biorxiv.org/content/10.1101/2024.04.06.588431v1
Genome Continuity Inspector (GCI) is an assembly assessment tool for T2T genomes. After stringently filtering the alignments generated by mapping long reads back to the genome assembly, GCI will report potential assembly issues and a score to quantify the continuity of assembly.
GCI integrates both contig N50 value and contig number of curated assembly and quantifies the gap of assembly continuity to a truly gapless T2T assembly. Even if the contig N50 value has been saturated, the contig numbers could be used to quantify the continuity differences.
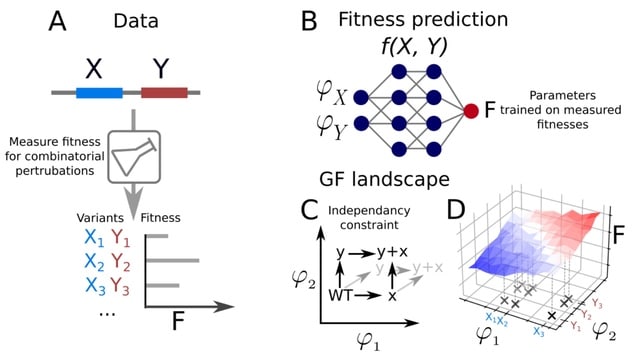
□ D-LIM: Hypothesis-driven interpretable neural network for interactions between genes
>> https://www.biorxiv.org/content/10.1101/2024.04.09.588719v1
D-LIM (the Direct-Latent Interpretable Model), a hypothesis-driven model for gene-gene interactions, which learns from genotype-to-fitness measurements and infers a genotype-to-phenotype and a phenotype-to-fitness map.
D-LIM comprises a genotype-phenotype map and a phenotype-fitness map. The D-LIM architecture is a neural network designed to learn genotype-fitness maps from a list of genetic mutations and associated fitness when distinct biological entities have been identified as meaningful.
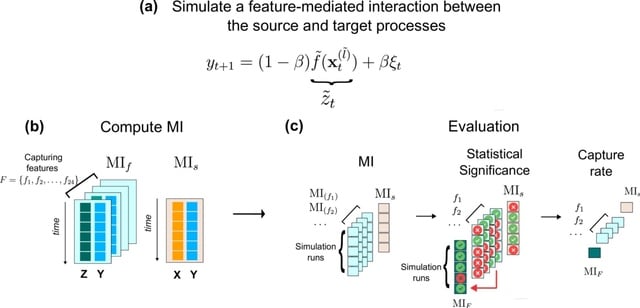
□ A feature-based information-theoretic approach for detecting interpretable, long-timescale pairwise interactions from time series
>> https://arxiv.org/abs/2404.05929
A feature-based adaptation of conventional information-theoretic dependence detection methods that combine data-driven flexibility w/ the strengths of time-series features. It transforms segments of a time series into interpretable summary statistics from a candidate feature set.
Mutual information is then used to assess the pairwise dependence between the windowed time-series feature values of the source process and the time-series values of the target process.
This method allows for the detection of dependence between a pair of time series through a specific statistical feature of the dynamics. Although it involves a trade-off in terms of information and flexibility compared to traditional methods that operate in the signal space.
It leverages more efficient representations of the joint probability of source and target processes, which is particularly beneficial for addressing challenges related to high-dimensional density estimation in long-timescale interactions.

□ PMF-GRN: a variational inference approach to single-cell gene regulatory network inference using probabilistic matrix factorization
>> https://genomebiology.biomedcentral.com/articles/10.1186/s13059-024-03226-6
PMF-GRN, a novel approach that uses probabilistic matrix factorization to infer gene regulatory networks from single-cell gene expression and chromatin accessibility information. PMF-GRN addresses the current limitations in regression-based single-cell GRN inference.
PMF-GRN uses a principled hyperparameter selection process, which optimizes the parameters for automatic model selection. It provides uncertainty estimates for each predicted regulatory interaction, serving as a proxy for the model confidence in each predicted interaction.
PMF-GRN replaces heuristic model selection by comparing a variety of generative models and hyperparameter configurations before selecting the optimal parameters with which to infer a final GRN.

□ CELEBRIMBOR: Pangenomes from metagenomes
>> https://www.biorxiv.org/content/10.1101/2024.04.05.588231v1
CELEBRIMBOR (Core ELEment Bias Removal In Metagenome Binned ORthologs) uses genome completeness, jointly with gene frequency to adjust the core frequency threshold by modelling the number of gene observations with a true frequency using a Poisson binomial distribution.
CELEBRIMBOR implements both computational efficient and accurate clustering workflows; mmseqs2, which scales to millions of gene sequences, and Panaroo, which uses sophisticated network-based approaches to correct errors in gene prediction and clustering.
CELEBRIMBOR enables a parametric recapitulation of the core genome using MAGs, which would otherwise be unidentifiable due to missing sequences resulting from errors in the assembly process.

□ ExDyn: Inferring extrinsic factor-dependent single-cell transcriptome dynamics using a deep generative model
>> https://www.biorxiv.org/content/10.1101/2024.04.01.587302v1
ExDyn, a deep generative model integrated with splicing kinetics for estimating cell state dynamics dependent on extrinsic factors. ExDyn provides a counterfactual estimate of cell state dynamics under different conditions for an identical cell state.
ExDyn identifies the bifurcation point between experimental conditions, and performs a principal mode analysis of the perturbation of cell state dynamics by multivariate extrinsic factors, such as epigenetic states and cellular colocalization.

□ GCNFrame: Coding genomes with gapped pattern graph convolutional network
>> https://academic.oup.com/bioinformatics/advance-article/doi/10.1093/bioinformatics/btae188/7644280
GCNFrame, a GP-GCN (Gapped Pattern Graph Convolutional Networks) framework for genomic study. GCNFrame transforms each gapped pattern graph (GPG) into a vector in a low-dimensional latent space; the vectors are then used in downstream analysis tasks.
Under the GP-GCN framework, they develop Graphage, a tool that performs four phage-related tasks: phage and integrative and conjugative element (ICE) discrimination. It calculates the contribution scores for the patterns and pattern groups to mine informative pattern signatures.

□ BiGCN: Leveraging Cell and Gene Similarities for Single-cell Transcriptome Imputation with Bi-Graph Convolutional Networks
>> https://www.biorxiv.org/content/10.1101/2024.04.05.588342v1
Bi-Graph Convolutional Network (BiGCN), a deep learning method that leverages both cell similarities and gene co-expression to capture cell-type-specific gene co-expression patterns for imputing ScRNA-seq data.
BIGCN constructs both a cell similarity graph and a gene co-expression graph, and employs them for convolutional smoothing in a dual two-layer Graph Convolutional Networks (GCNs). BiGCN can identify true biological signals and distinguish true biological zeros from dropouts.

□ Emergence of fractal geometries in the evolution of a metabolic enzyme
>> https://www.nature.com/articles/s41586-024-07287-2
The discovery of a natural metabolic enzyme capable of forming Sierpiński triangles in dilute aqueous solution at room temperature. They determine the structure, assembly mechanism and its regulation of enzymatic activity and finally how it evolved from non-fractal precursors.
Although they cannot prove that the larger assemblies are Sierpiński triangles rather than some other type of assembly, these experiments indicate that the protein is capable of extended growth, as predicted for fractal assembly.
シアノバクテリアのクエン酸シンターゼによる自己組織化過程におけるフラクタル構造の発現。シルピンスキー・ギャスケットだ!

□ Islander: Metric Mirages in Cell Embeddings
>> https://www.biorxiv.org/content/10.1101/2024.04.02.587824v1
Islander , a model that scores best on established metrics, but generates biologically problematic embeddings. Islanderis a three-layer perceptron, directly trained on cell type annotations with mixup augmentations.
scGraph compares each affinity graph to a consensus graph, derived by aggregating individual graphs from different batches, based on raw reads or PCA loadings. Evaluation by scGraph revealed varied performance across embeddings.

□ EpiSegMix: a flexible distribution hidden markov model with duration modeling for chromatin state discovery
>> https://academic.oup.com/bioinformatics/advance-article/doi/10.1093/bioinformatics/btae178/7639383
EpiSegMix, a novel segmentation method based on a hidden Markov model with flexible read count distribution types and state duration modeling, allowing for a more flexible modeling of both histone signals and segment lengths.
EpiSegMix first estimates the parameters of a hidden Markov model, where each state corresponds to a different combination of epigenetic modifications and thus represents a functional role, such as enhancer, transcription start site, active or silent gene.
The spatial relations are captured via the transition probabolities. After the parameter estimation, each region in the genome is annotated w/ the most likely chromatin state. The implementation allows to choose for each histone modification a different distributional assumption.

□ SVEN: Quantify genetic variants' regulatory potential via a hybrid sequence-oriented model
>> https://www.biorxiv.org/content/10.1101/2024.03.28.587115v1
Trying to "learn and model" regulatory codes from DNA sequences directly via DL networks, sequence-oriented methods have demonstrated notable performance in predicting the expression influence for SNV and small indels, in both well-annotated and poor-annotation genomic regions.
SVEN employs a hybrid architecture to learn regulatory grammars and infer gene expression levels from promoter-proximal sequences in a tissue-specific manner.
SVEN is trained with multiple regulatory-specific neural networks based on 4,516 transcription factor (TF) binding, histone modification and DNA accessibility features across over 400 tissues and cell lines generated by ENCODE.

□ PSMutPred: Decoding Missense Variants by Incorporating Phase Separation via Machine Learning
>> https://www.biorxiv.org/content/10.1101/2024.04.01.587546v1
LLPS (liquid-liquid phase separation) is tightly linked to intrinsically disordered regions (IDRs), into the analysis of missense variants. LLPS is vital for multiple physiological processes.
PSMutPred, an innovative machine-learning approach to predict the impact of missense mutations on phase separation. PSMutPred shows robust performance in predicting missense variants that affect natural phase separation.

□ EAP: a versatile cloud-based platform for comprehensive and interactive analysis of large-scale ChIP/ATAC-seq data sets
>> https://www.biorxiv.org/content/10.1101/2024.03.31.587470v1
Epigenomic Analysis Platform (EAP), a scalable cloud-based tool that efficiently analyzes large-scale ChIP/ATAC-seq data sets.
EAP employs advanced computational algorithms to derive biologically meaningful insights from heterogeneous datasets and automatically generates publication-ready figures and tabular results.

□ PROTGOAT : Improved automated protein function predictions using Protein Language Models
>> https://www.biorxiv.org/content/10.1101/2024.04.01.587572v1
PROTGOAT (PROTein Gene Ontology Annotation Tool) that integrates the output of multiple diverse PLMs with literature and taxonomy information about a protein to predict its function.
The TF-IDF vectors for each protein were then merged for the full list of train and test protein IDs, filling proteins with no text data with zeros, and then structured into a final numpy embedding for use in the final model.

□ Combs, Causality and Contractions in Atomic Markov Categories
>> https://arxiv.org/abs/2404.02017
Markov categories with conditionals need not validate a natural scheme of axioms which they call contraction identities. These identities hold in every traced monoidal category, so in particular this shows that BorelStoch cannot be embedded in any traced monoidal category.
Atomic Markov categories validate all contraction identities, and furthermore admit a notion of trace defined for non-signalling morphisms. Atomic Markov categories admit an intrinsic calculus of combs without having to assume an embedding into compact-closed categories.
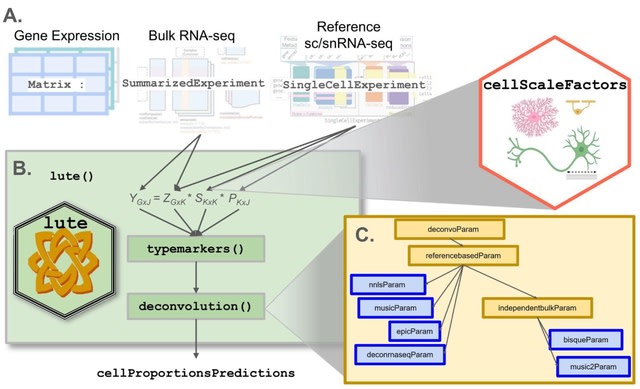
□ lute: estimating the cell composition of heterogeneous tissue with varying cell sizes using gene expression
>>
lute, a computational tool to accurately deconvolute cell types with varying cell sizes in heterogeneous tissue by adjusting for differences in cell sizes. lute wraps existing deconvolution algorithms in a flexible and extensible framework to enable their easy benchmarking and comparison.
For algorithms that currently do not account for variability in cell sizes, lute extends these algorithms by incorporating user-specified cell scale factors that are applied as a scalar product to the cell type reference and then converted to algorithm-specific input formats.

□ Originator: Computational Framework Separating Single-Cell RNA-Seq by Genetic and Contextual Information
>> https://www.biorxiv.org/content/10.1101/2024.04.04.588144v1
Originator deconvolutes barcoded cells into different origins using inferred genotype information from scRNA-Seq data, as well as separating cells in the blood from those in solid tissues, an issue often encountered in scRNA-Seq experimentation.
Originator can systematically decipher scRNA-Seq data by genetic origin and tissue contexts in heterogeneous tissues. Originator can remove the undesirable cells. It provides improved cell type annotations and other downstream functional analyses, based on the genetic background.

□ DAARIO: Interpretable Multi-Omics Data Integration with Deep Archetypal Analysis
>> https://www.biorxiv.org/content/10.1101/2024.04.05.588238v1
DAARIO (Deep Archetypal Analysis for the Representation of Integrated Omics) supports different input types and neural network architectures, adapting seamlessly to the high complexity data, which ranges from counts in sequencing assays to binary values in CpG methylation assays.
DAARIO encodes the multi-modal data into a latent simplex. In principle, DAARIO could be extended to combine data from non-omics sources (text and images) when combined with embeddings from other deep-learning models.

□ MGPfactXMBD: A Model-Based Factorization Method for scRNA Data Unveils Bifurcating Transcriptional Modules Underlying Cell Fate Determination
>> https://www.biorxiv.org/content/10.1101/2024.04.02.587768v1
MGPfactXMBD, a model-based manifold-learning method which factorize complex cellular trajectories into interpretable bifurcation Gaussian processes of transcription. It enables discovery of specific biological determinants of cell fate.
MGPfact is capable to distinguish discrete and continuous events in the same trajectory. The MGPfact-inferred trajectory is based solely on pseudotime, neglecting potential bifurcation processes occurring in space.

>> https://www.biorxiv.org/content/10.1101/2024.04.04.588041v1
The PhenoMultiOmics web server incorporates a biomarker discovery module for statistical and functional analysis. Differential omic feature data analysis is embedded, which requires the matrices of gene expression, proteomics, or metabolomics data as input.
Each row of this matrix represents a gene or feature, and each column corresponds to a sample ID. This analysis leverages the lima R package to calculate the Log2 Fold Change (Log2FC), estimating differences between case and control groups.

□ Alleviating cell-free DNA sequencing biases with optimal transport
>> https://www.biorxiv.org/content/10.1101/2024.04.04.588204v1
OT builds on strong mathematical bases and allows to define a patient-to-patient relationship across domains without the need to build a common latent representation space, as mostly done in the domain adaptation (DA) field.
Because they originally designed this approach for the correction of normalised read counts within predefined bins, it falls under the category of "global models" according to the Benjamini/Speed classification.
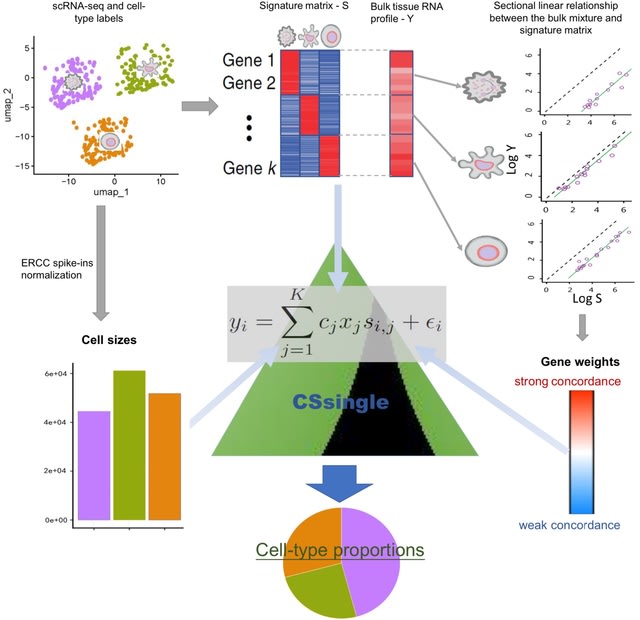
□ Leveraging cross-source heterogeneity to improve the performance of bulk gene expression deconvolution
>> https://www.biorxiv.org/content/10.1101/2024.04.07.588458v1
CSsingle (Cross-Source SINGLE cell deconvolution) decomposes bulk transcriptomic data into a set of predefined cell types using the scRNA-seq or flow sorting reference.
Within CSsingle, the cell sizes are estimated by using ERCC spike-in controls which allow the absolute RNA expression quantification. CSsingle is a robust deconvolution method based on the iteratively reweighted least squares approach.
An important property of marker genes (i.e. there is a sectional linear relationship between the individual bulk mixture and the signature matrix) is employed to generate an efficient and robust set of initial estimates.
CSsingle is a robust deconvolution method based on the concept of iteratively reweighted least squares (IRLS). The sectional linearity corresponds to the linear relationship between the individual bulk mixture and the cell-type-specific GEPs on a per-cell-type basis.
CSsingle up-weights genes that exhibit stronger concordance and down-weights genes with weaker concordance between the individual bulk mixture and the signature matrix.

□ vcfgl: A flexible genotype likelihood simulator for VCF/BCF files
>> https://www.biorxiv.org/content/10.1101/2024.04.09.586324v1
vegl, a lightweight utility tool for simulating genotype likelihoods. The program incorporates a comprehensive framework for simulating uncertainties and biases, including those specific to modern sequencing platforms.
vegl can simulate sequencing data, quality scores, calculate the genotype likelihoods and various VCF tags, such as 116 and QS tags used in downstream analyses for quantifying the base calling and genotype uncertainty.
vefgl uses a Poisson distribution with a fixed mean. It utilizes a Beta distribution where the shape parameters are adjusted to obtain a distribution with a mean equal to the specified error probability and variance equal to a specified variance parameter.

□ scPanel: A tool for automatic identification of sparse gene panels for generalizable patient classification using scRNA-seq datasets
>> https://www.biorxiv.org/content/10.1101/2024.04.09.588647v1
sPanel, a computational framework designed to bridge the gap between biomarker discovery and clinical application by identifying a minimal gene panel for patient classification from the cell population(s) most responsive to perturbations.
scPanel incorporates a data-driven way to automatically determine the number of selected genes. Patient-level classification is achieved by aggregating the prediction probabilities of cells associated with a. patient using the area under the curve score.

□ SimReadUntil for Benchmarking Selective Sequencing Algorithms on ONT Devices
>> https://academic.oup.com/bioinformatics/advance-article/doi/10.1093/bioinformatics/btae199/7644279
SimReadUntil, a simulator for an ONT device controlled by the ReadUntil API either directly or via gRPC, and can be accelerated (e.g. factor 10 w/ 512 channels). It takes full-length reads as input, plays them back with suitable gaps in between, and responds to ReadUntil actions.
SimReadUntil enables benchmarking and hyperparameter tuning of selective sequencing algorithms. The hyperparameters can be tuned to different ONT devices, e.g., a GridION with a GPU can compute more than a portable MinION/Flongle that relies on an external computer.

□ Predictomes: A classifier-curated database of AlphaFold-modeled protein-protein interactions
>> https://www.biorxiv.org/content/10.1101/2024.04.09.588596v1
This classifier considers structural features of each protein pair and is called SPOC (Structure Prediction and Omics-based Classifier). SPOC outperforms standard metrics in separating true positive and negative predictions, incl. in a proteome-wide in silico screen.
A compact SPOC is accessible at predictomes.org and will calculate scores for researcher-generated AF-M predictions. This tool works best when applied to predictions generated using AF-M settings that resemble as closely as possible those used to train the classifier.

□ Effect of tokenization on transformers for biological sequences
>> https://academic.oup.com/bioinformatics/advance-article/doi/10.1093/bioinformatics/btae196/7645044
Applying alternative tokenization algorithms can increase accuracy and at the same time, substantially reduce the input length compared to the trivial tokenizer in which each character is a token.
It allows interpreting trained models, taking into account dependencies among positions. They trained these tokenizers on a large dataset of protein sequences containing more than 400 billion amino acids, which resulted in over a three-fold decrease in the number of tokens.