

□ GENELink: Graph attention network for link prediction of gene regulations from single cell RNA-sequencing data
>> https://academic.oup.com/bioinformatics/advance-article-abstract/doi/10.1093/bioinformatics/btac559/6663989
GENELink infers latent interactions between transcription factors (TFs) and target genes in GRN using graph attention network. GENELink integrates gene expression matrix (N×M) with prior gene topology (N×N) to learn low-dimensional vertorized representations with supervision.
GENELink projects the single-cell gene expression with observed TF-gene pairs to a low-dimensional space. Then, the specific gene representations are learned to serve for downstream similarity measurement or causal inference of pairwise genes by optimizing the embedding space.
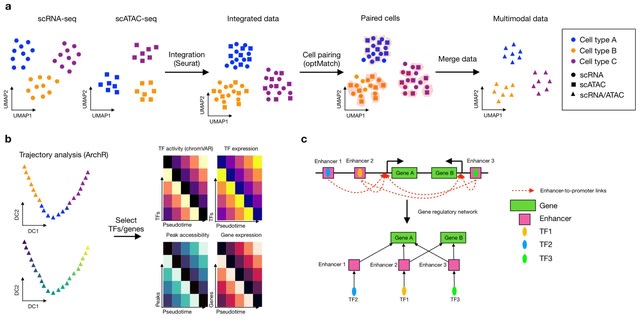
□ scMEGA: Single-cell Multiomic Enhancer-based Gene Regulatory Network Inference
>> https://www.biorxiv.org/content/10.1101/2022.08.10.503335v1.full.pdf
scMEGA is built upon Seurat, Signac and ArchR for single-cell data analysis. It enables users to perform end-to-end GRN inferences and prioritize important TFs and genes for experimental validation and the use of regulomes to analyze spatial transcriptomics.
scMEGA integrates the single-cell multi-omics profiles to create a pseudo-multimodal dataset where each cell is characterized by gene expression and chromatin accessibility. scMEGA calculates the correlation between TF binding activity and TF expression.

□ Satellite Repeat Finder:
>> https://github.com/lh3/srf
Satellite Repeat Finder (SRF) assembles motifs in satellite DNA that are tandemly repeated many times in the genome. It takes short reads, accurate long reads or high-quality contigs as input and reports the consensus of each repeat unit.
SRF can identify satellite repeats that are often missed in de novo assembly. It tends to find HORs instead of the minimal repeat unit. SRF may also find truly circular genomes. SRF works best with phased telomere-to-telomere assemblies and may work with trio hifiasm assemblies.

□ NAE: Evaluating gene regulatory network activity from dynamic expression data by regularized constraint programming
>> https://ieeexplore.ieee.org/document/9858601/
NAE employs the dynamic Bayesian network model to formulate the network structure with time series profiling data. NAE introduces an interpretable general loss function with regularization penalties to calculate the degree of consistency between gene network and gene expression data.
NAE uses a fast and convergent alternating direction method of multipliers (ADMM) algorithm to optimize the regularized constraint programming.
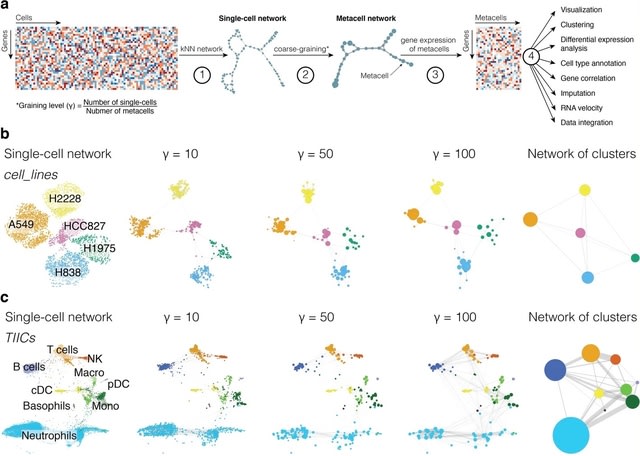
□ SuperCell: Metacells untangle large and complex single-cell transcriptome networks
>> https://bmcbioinformatics.biomedcentral.com/articles/10.1186/s12859-022-04861-1
SuperCell merges highly similar cells into metacells and perform standard analyses at the metacell level. scRNA-seq data are modeled as a kNN graph with nodes representing / edges connecting cells. Metacells are built by merging single cells with very high internal connectivity.
SuperCell uses the walktrap algorithm. The graining level is defined as the ratio b/n the number of cells / metacells. A metacell GE matrix is computed by averaging GE within metacells. It accelerates the construction of single-cell atlases, the integration of 1.46 million cells.

□ veloVI: Deep generative modeling of transcriptional dynamics for RNA velocity analysis in single cells
>> https://www.biorxiv.org/content/10.1101/2022.08.12.503709v1.full.pdf
veloVI (velocity variational inference) reformulates the inference of RNA velocity via a model that shares information between all cells and genes while learning the same quantities, namely kinetic parameters and latent time.
veloVI returns a posterior distribution of RNA velocity. This distribution can be used to quantify an intrinsic uncertainty over first- order directions a cell can take in the gene space. veloVI adds a notion of confidence to the velocity stream and highlights regions of the phenotypic manifold.

□ DeepGAMI: Deep biologically guided auxiliary learning for multimodal integration and imputation to improve phenotype prediction
>> https://www.biorxiv.org/content/10.1101/2022.08.16.504101v1.full.pdf
DeepGAMI uses prior biological knowledge to define the neural network architecture. Notably, it embeds an auxiliary-learning layer for cross-modal imputation while training the model from multimodal data.
DeepGAMI impute latent features of additional modalities and enable predicting phenotypes from a single modality only. DeepGAMI uses integrated gradient to prioritize multimodal features and links for phenotypes.

□ BLAZE: Identification of cell barcodes from long-read single-cell RNA-seq
>> https://www.biorxiv.org/content/10.1101/2022.08.16.504056v1.full.pdf
BLAZE provides accurate cell barcodes over a wide range of experimental read depths and sequencing accuracies, while other methodologies commonly identify false-positive barcodes and cell clusters, disrupting biological interpretation of LR scRNA-seq results.
BLAZE eliminates the requirement for matched SR scRNA-seq to interpret LR scRNA-seq. BLAZE seamlessly integrates the existing FLT-seq - FLAMES to enable identification and quantification of RNA isoforms and their expression profiles across individual cells and cell-types.

□ BindVAE: Dirichlet variational autoencoders for de novo motif discovery from accessible chromatin
>> https://genomebiology.biomedcentral.com/articles/10.1186/s13059-022-02723-w
BindVAE, based on Dirichlet variational autoencoders, for jointly decoding multiple TF binding signals from open chromatin regions. The VAE formulation of latent variable models uses advances in neural network learning and enables training using backpropagation of gradients.
BindVAE can disentangle an input DNA sequence into distinct latent factors that encode cell-type specific in vivo binding signals for individual TFs, composite patterns for TFs involved in cooperative binding, and genomic context surrounding the binding sites.

□ Cell Layers: uncovering clustering structure in unsupervised single-cell transcriptomic analysis
>> https://academic.oup.com/bioinformaticsadvances/article/2/1/vbac051/6655723
Cell Layers, an interactive Sankey tool for the quantitative investigation of GE, co-expression, biological processes and cluster integrity. Cell Layers enhances the interpretability of single-cell clustering by linking molecular data and cluster evaluation metrics.
In Cell Layers, the default construction of a kNN graph is based on the Euclidean distance of a user-defined PCA subspace. modularity and hierarchical clustering methods, cells are then iteratively grouped to optimize a modularity function thresholded by a resolution parameter.

□ ELIMINATOR: essentiality analysis using multisystem networks and integer programming
>> https://bmcbioinformatics.biomedcentral.com/articles/10.1186/s12859-022-04855-z
An in-silico method for the identification of patient-specific essential genes using constraint-based modelling (CBM). It first calculates the minimum number of lowly expressed genes required to be activated by the cell to sustain life as defined by a set of requirements.
These inputs are subsequently encoded into a mathematical model (Integer Linear Program) that finds the minimum number of lowly expressed genes required to activate the given relevant function.
ELIMINATOR identifies artificial gene knockouts that lead to require unexpressed genes to activate the critical biological entity/process. The Essentiality Congruity Score assigns a quantitative value to an otherwise binary score to represent the essentiality of a gene.

□ Scarf enables a highly memory-efficient analysis of large-scale single-cell genomics data
>> https://www.nature.com/articles/s41467-022-32097-3
Scarf wraps memory-efficient implementations of a graph-based t-stochastic neighbour embedding and hierarchical clustering algorithm. Moreover, Scarf performs accurate reference-anchored mapping of datasets while maintaining memory efficiency.
Scarf uses out-of-core (incremental) implementations of the algorithms that allow the iterative input in small chunks. It leads to the creation of a cell-cell neighbourhood graph structure which can be used for downstream steps like generating UMAP/t-SNE and pseudotime ordering.

□ WITCH-NG: Efficient and Accurate Alignment of Datasets with Sequence Length Heterogeneity
>> https://www.biorxiv.org/content/10.1101/2022.08.08.503232v1.full.pdf
Although WITCH-NG is designed for de novo multiple sequence alignment, it can also be used directly to add sequences into alignments, a problem that arises in updating existing alignments and trees as new sequences are assembled.
WITCH-NG sets all non-positive entries of S as −∞ and runs a by a polynomial time exact algorithm to align q to B with a constant zero gap penalty. Both Smith-Waterman and Needleman-Wunsch simplify into the same dynamic programming algorithm using this S as the scoring matrix.

□ SMaSH: a scalable, general marker gene identification framework for single-cell RNA-sequencing
>>.https://bmcbioinformatics.biomedcentral.com/articles/10.1186/s12859-022-04860-2
SMaSH extracts robust and biologically well-motivated marker genes, which characterise a given single-cell RNA-sequencing data-set better than existing computational approaches for general marker gene calculation.
SMaSH has been fully-integrated with the ScanPy framework. The framework is divided into four stages, beginning from the AnnData object which contains the raw scRNA-seq counts in a matrix of dimensionality determined by the number of barcoded cells and unique genes.

□ LRTK: A unified and versatile toolkit for analyzing linked-read sequencing data
>> https://www.biorxiv.org/content/10.1101/2022.08.10.503458v1.full.pdf
LRTK is a toolkit to process linked-read sequencing data from 10x genomics, stLFR, and TELL-Seq technologies. LRTK provides flexible functions to perform data simulation, format conversion, data preprocessing, barcode-aware read alignment, SNV/INDEL/SV.
LRTK (FreeBayes) achieved average recall rates of 94% for SNVs and 73% for INDELs. LRTK increased phase block N50 up to 26.1 Mb and 19.4 Mb for 10x linked-reads and stLFR. LRTK (Aquila) outperformed Long Ranger with respect to the recall of SVs, especially the deletions.

□ SEESAW: Detecting isoform-level allelic imbalance accounting for inferential uncertainty
>> https://www.biorxiv.org/content/10.1101/2022.08.12.503785v1.full.pdf
Statistical Estimation of Allelic Expression using Salmon and Swish (SEESAW), for inference of AI patterns. SEESAW utilizes Salmon to estimate expression with respect to an allele-specific reference transcriptome, and a non-parametric test Swish to test for AI.
SEESAW assumes that phased genotypes are available, and is designed for multiple replicates or conditions of organisms with the same genotype. SEESAW detects cases of AI that are consistent across all samples, differential AI across two groups, or dynamic AI over a covariate.

□ REViewer: haplotype-resolved visualization of read alignments in and around tandem repeats
>> https://genomemedicine.biomedcentral.com/articles/10.1186/s13073-022-01085-z
REViewer finds the top-scoring alignments to any haplotype sequence. A read pair originating completely within a sequence surrounding the repeats and shared by all haplotypes has exactly one alignment position on each haplotype.
REViewer selects pairs of alignments that correspond to fragment length closest to the mean fragment length calculated for read pairs mapping to the flanking regions surrounding the repeats. And generates read pileup by selecting one pair of alignments at random for each read.

□ MONI-k: An index for efficient pangenome-to-pangenome comparison
>> https://www.biorxiv.org/content/10.1101/2022.08.09.503358v1.full.pdf
MONI consists a run-length compressed BWT with suffix-array entries stored for each position i at a run boundary and a balanced, locally consistent SLP for T. This occupies O(r + g) words of space, where r is the number of runs in the BWT and g is the number of rules in the SLP.
MONI-k defines k-MEMs to be maximal substrings of a pattern that each occur exactly at least k times in a text (so a MEM is a 1- MEM) and briefly explain why computing k-MEMs could be useful for pangenome-to-pangenome comparison.

□ Constrained Fourier estimation of short-term time-series gene expression data reduces noise and improves clustering and gene regulatory network predictions
>> https://bmcbioinformatics.biomedcentral.com/articles/10.1186/s12859-022-04839-z
The constrained Fourier de-noising method helps to cluster noisy gene expression and interpret dynamic gene networks more accurately. The benefit of noise reduction is large and can constitute the difference between a successful application and a failing one.
Constrained Fourier with one and two harmonics sufficiently estimated noisy data. Approximating the temporal data using an optimal least squares trust-region method, and restricted the optimality search to frequencies that can construct these basic patterns.

□ CircWalk: a novel approach to predict CircRNA-disease association based on heterogeneous network representation learning
>> https://bmcbioinformatics.biomedcentral.com/articles/10.1186/s12859-022-04883-9
Considering the ceRNA hypothesis, they integrate multiple resources to construct a heterogeneous network from circRNAs, mRNAs, miRNAs, and diseases. Next, the DeepWalk algorithm is applied to the network to extract feature vectors for circRNAs and diseases.
The XGBoost is utilized to generate a novel approach, called CircWalk, to predict CircRNA-Disease associations. Seven types of bipartite networks were combined based on their common nodes. circRNAs have multiple notations. To avoid duplication, CircWalk uses the CircBase dataset.

□ medna-metadata: an open-source data management system for tracking environmental DNA samples and metadata
>> https://academic.oup.com/bioinformatics/advance-article/doi/10.1093/bioinformatics/btac556/6663773
medna-metadata, an open-source, modular system that aligns with Findable, Accessible, Interoperable, and Reusable (FAIR) guiding principles that support scholarly data reuse and the database and application development of a standardized metadata collection structure.
The metadata database schema was developed to be extendable to other cases. The system can still track samples that could be characterized using other seq methods, but the mednaーmetadata application would need to be modified to support sequencing workflows beyond metabarcoding.

□ binny: an automated binning algorithm to recover high-quality genomes from complex metagenomic datasets
>> https://www.biorxiv.org/content/10.1101/2021.12.22.473795v5.full.pdf
binny combines k-mer composition, read coverage, and lineage-specific marker gene sets for iterative, non-linear dimension reduction of genomic signatures and subsequent automated contig clustering with cluster assessment.
The Fast Fourier Transform-accelerated Interpolation-based t-distributed Stochastic Neighbor Embedding (FIt-SNE) implementation of openTSNE is used.
binny produced high-quality MAGs from contiguous as well as highly fragmented genomes. PCA is used beforehand to lower the dimensionality of the initial feature matrix to either as many dimensions needed to explain 75% of the variation or to a maximum of 75 dimensions.

□ Accessible, interactive and cloud-enabled genomic workflows integrated with the NCI Genomic Data Commons
>> https://www.biorxiv.org/content/10.1101/2022.08.11.503660v1.full.pdf
The GDC mRNA-Seq workflow aligns raw sequence files to the GRCh38.d1.vd1 reference sequence using the STAR (Spliced Transcripts Alignment to a Reference) aligner, followed by the quantification step that outputs raw read counts and normalized read counts.
This implementation in the Bwb consisting of the following steps: download the reference and sample data; create a genome index using the reference sequence; align reads to the reference, quantify the number of reads mapped to each gene, and calculate normalized GE values.

□ Cytocipher detects significantly different populations of cells in single cell RNA-seq data
>> https://www.biorxiv.org/content/10.1101/2022.08.12.503759v1.full.pdf
Distinct cell populations may exist that are not clearly demarcated by a single marker gene, but instead co-express a unique combination of genes; a phenomenon which is difficult to detect by manual examination.
Cytocipher, an scverse compatible bioinformatics method and software that scores cells for unique combinatorial gene co-expression and statistically tests whether clusters are significantly different.

□ classLog: Logistic regression for the classification of genetic sequences
>> https://www.biorxiv.org/content/10.1101/2022.08.15.503907v1.full.pdf
classLog, a machine learning logistic regression pipeline that can assign classifications to genetic sequence data. classLog implements an intuitive approach to developing a trained prediction model that runs in linear time complexity, generating accurate output more rapidly.
Once a logistic regression classifier has been trained on a high-quality multisequence alignment that broadly covers all cases interest, that classifier can be recyled to classify unknown sequences in linear run time.
This classification is based on the idea that clade defining mutations are linearly seperable where each position in the sequence is a nominal axis.

□ node2vec+: Accurately modeling biased random walks on weighted networks
>> https://www.biorxiv.org/content/10.1101/2022.08.14.503926v1.full.pdf
node2vec+, a natural extension of node2vec that accounts for edge weights when calculating walk biases and reduces to node2vec in the cases of unweighted graphs or unbiased walks.
node2vec+ is more effective for weighted graphs by taking into account the edge weight connecting the previous vertex and the potential next vertex.

□ DeepUMQA2: Improved model quality assessment using sequence and structural information by enhanced deep neural networks
>> https://www.biorxiv.org/content/10.1101/2022.08.12.503819v1.full.pdf
On the basis of the features of the input model, sequence features from multiple sequence alignment and structural features from homologous templates are incorporated for the characterization of the potential properties of the model.

□ AutoComplete: Deep Learning-based Phenotype Imputation on Population-scale Biobank Data Increases Genetic Discoveries
>> https://www.biorxiv.org/content/10.1101/2022.08.15.503991v1.full.pdf
AutoComplete employes copy-masking, a procedure that propagates missingness patterns present in the data. AutoComplete can impute both binary and continuous phenotypes while scaling with ease to datasets with half a million individuals and millions of entries.
Given a vector of features that represent the phenotypes measured on an individual, AutoComplete maps the features to a hidden-representation using a non-linear transformation which is then mapped back to the original space of features to reconstruct the phenotypes.

□ SimpleMKKM: Simple Multiple Kernel K-Means
>> https://arxiv.org/pdf/2005.04975.pdf
SimpleMKKM extends the widely used supervised kernel alignment criterion to multi-kernel clustering. This criterion is given by an intractable minimization-maximization problem in the kernel coefficient and clustering partition matrix.
SimpleMKKM re-formulates the problem as a smooth mini- mization one, which can be solved efficiently us- ing a reduced gradient descent algorithm.

□ Deep Local Analysis evaluates protein docking conformations with locally oriented cubes
>> https://academic.oup.com/bioinformatics/advance-article/doi/10.1093/bioinformatics/btac551/6665900
DLA-Ranker successfully identifies near-native conformations from ensembles generated by molecular docking. DLA-Ranker considers the local geometry of the interfacial residues along with their neighboring atoms and the regions of the interface w/ different solvent accessibility.

□ ARAX: a graph-based modular reasoning tool for translational biomedicine
>> https://www.biorxiv.org/content/10.1101/2022.08.12.503810v1.full.pdf
ARAXi is ARAX’s intuitive language for specifying a workflow for analyzing a knowledge graph. ARAX accesses to 15 knowledge providers (which themselves access over 100 underlying knowledge sources) from a single reasoning tool, using a standardized interface and semantic layer.

□ GraphMB: Metagenomic binning with assembly graph embeddings
>> https://academic.oup.com/bioinformatics/advance-article/doi/10.1093/bioinformatics/btac557/6668279
GraphMB, a binner developed using long-read metagenomic data and incorporates the assembly graph into the contig features learning process, taking full advantage of its potential by training a neural network to give more importance to higher coverage edges.
GraphMB requires an assembly consisting of a set of contig sequences in FASTA format and an assembly graph in GFA format.
The edge read coverage is used to assign different weights to graph edges, so that edges with higher coverage have a more impact in the model. GraphMB is also compatible with GFA files that do not have this information.

□ syntenet: an R/Bioconductor package for the inference and analysis of synteny networks
>> https://www.biorxiv.org/content/10.1101/2022.08.16.504079v1.full.pdf
syntenet offers a simple and complete framework, including data preprocessing, synteny detection and network inference, network clustering and phylogenomic profiling, and microsynteny-based phylogeny inference.
Network clustering is performed with the Infomap algorithm. Synteny networks can be explored to detect deeply conserved and taxa-specific clusters, to explore genomic rearrangement.

□ SEAT: Incorporating cell hierarchy to decipher the functional diversity of single cells
>> https://www.biorxiv.org/content/10.1101/2022.08.17.504240v1.full.pdf
SEAT constructs cell hierarchies utilizing structure entropy by minimizing the global uncertainty in cell-cell graphs. With cell hierarchies, SEAT deciphers functional diversity in 36 data sets covering scRNA, scDNA, scATAC, and scRNA-scATAC multiome.
SEAT finds optimal cell subpopulations with high clustering accuracy. It identifies cell types or fates from omics profiles and boosts accuracy from 0.34 to 1. Second, SEAT detects insightful functional diversity among cell clubs. SEAT cell hierarchy generates cell order representing the cell cycle pseudotime.