
現象から現象(機序の一部)を説明することは回り道が効かないが、有限な事象の因果を辿る扉は無数に在る。

□ ENIGMA: an enterotype-like unigram mixture model
>> https://bmcgenomics.biomedcentral.com/articles/10.1186/s12864-019-5476-9
ENIGMA uses Operational Taxonomic Units abundances as input and models each sample by the underlying unigram mixture whose parameters are represented by unknown group effects and known effects of interest.
ENIGMA is regarded as Bayesian learning for detecting associations between a community structure and factors of interest.
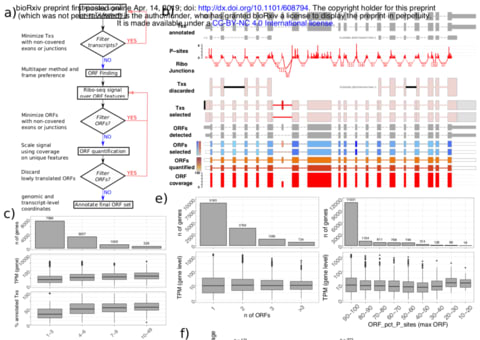
□ SaTAnn quantifies translation on the functionally heterogeneous transcriptome
>> https://www.biorxiv.org/content/biorxiv/early/2019/04/14/608794.full.pdf
SaTAnn (SaTAnn, Splice-aware Translatome Annotation) is a a new approach to annotate and quantify translation at the single Open Reading Frame level, that uses information from ribosome profiling to determine the translational state of each isoform in a comprehensive annotation. For most genes, one ORF represents the dominant translation product, but SaTAnn also detects translation from ORFs belonging to multiple transcripts per gene, including targets of RNA surveillance mechanisms such as nonsense-mediated decay.
□ Aligning the Aligners: Comparison of RNA Sequencing Data Alignment and Gene Expression Quantification
>> https://www.mdpi.com/2075-4426/9/2/18
STAR aligns the first portion, referred to as “seed”, for a specific read sequence output against a reference genome to the maximum mappable length (MML) of the read.
STAR alignment yielded more precise and accurate results with the fewest misalignments to pseudogenes compared to HISAT2.

□ ChromDragoNN : Integrating regulatory DNA sequence and gene expression of trans-regulators to predict genome-wide chromatin accessibility across cellular contexts
>> https://www.biorxiv.org/content/biorxiv/early/2019/04/11/605717.full.pdf
The integrative multi-modal deep learning architectures for predictive models that generalize across cellular contexts and obtaining insight into the dynamics of gene regulation. More transparent encodings of the gene expression space (e.g. using latent variables that directly model modules of functionally related genes or pathway annotations) would also improve interpretability.
ChromDragoNN Neural network architecture has 2 residual blocks, each with 2 convolution layers with 200 channels and filter size, Fully connected layer with 1000 dimension output.

□ ERASE: Extended Randomization for assessment of annotation enrichment in ASE datasets
>> https://www.biorxiv.org/content/biorxiv/early/2019/04/05/600411.full.pdf
ERASE is based on a randomization approach and controls for read depth, a significant confounder in ASE analyses.

□ Hybrid model for efficient prediction of Poly(A) signals in human genomic DNA
>> https://www.sciencedirect.com/science/article/pii/S104620231830361X
PolyA_Predicion_LRM_DNN predicts poly(A) signal (PAS) in human genomic DNA. It first utilizes signal processing transforms (Fourier-based and wavelet-based), statistics and position weight matrix PWM to generate sets of features that can help the poly(A) prediction problem.
Then, it uses deep neural networks DNN and Logistic Regression Model (LRM) to distinguish between true PAS and pseudo PAS efficiently.
The hybrid model contains 8 deep neural networks and 4 logistic regression models, and compared the results obtained by HybPAS to those reported by the state-of-the-art methods for PAS recognition, i.e., Omni-PolyA, DeepGSR, and DeeReCT-PolyA.

□ DeepSignal: detecting DNA methylation state from Nanopore sequencing reads using deep-learning
>> https://academic.oup.com/bioinformatics/advance-article-abstract/doi/10.1093/bioinformatics/btz276/5474907
DeepSignal can predict methylation states of 5% more DNA CpGs that previously cannot be predicted by bisulfite sequencing, and can achieve 90% above accuracy for detecting 5mC and 6mA using only 2x coverage of reads.
DeepSignal constructs a BiLSTM+Inception structure to detect DNA methylation state from Nanopore reads. DeepSignal achieves 90% correlation with bisulfite sequencing using just 20x coverage of reads, which is much better than HMM based methods.
□ POLYTE: Overlap graph-based generation of haplotigs for diploids and polyploids
>> https://academic.oup.com/bioinformatics/advance-article-abstract/doi/10.1093/bioinformatics/btz255/5474903
This method follows an iterative scheme where in each iteration reads or contigs are joined, based on their interplay in terms of an underlying haplotype-aware overlap graph.
Along the iterations, contigs grow while preserving their haplotype identity. Benchmarking experiments on both real and simulated data demonstrate that POLYTE establishes new standards in terms of error-free reconstruction of haplotype-specific sequence.
HaploConduct is a package designed for reconstruction of individual haplotypes from next generation sequencing data, in particular Illumina. Currently, HaploConduct consists of two methods: SAVAGE and POLYTE.

□ dnaasm-link: Linking De Novo Assembly Results with Long DNA Reads Using the dnaasm-link Application
>> https://www.hindawi.com/journals/bmri/2019/7847064/
dnaasm-link includes an integrated module to fill gaps with a suitable fragment of an appropriate long DNA read, which improves the consistency of the resulting DNA sequences.
dnaasm-link significantly optimizes memory and reduces computation time; it fills gaps with an appropriate fragment of a specified long DNA read; it reduces the number of spanned and unspanned gaps in existing genome drafts.

□ MemorySeq: Memory sequencing reveals heritable single cell gene expression programs associated with distinct cellular behaviors
>> https://www.biorxiv.org/content/biorxiv/early/2018/07/27/379016.full.pdf
a method combining Luria and Delbrück’s fluctuation analysis with population-based RNA sequencing (MemorySeq) for identifying genes transcriptome-wide whose fluctuations persist for several cell divisions. MemorySeq revealed multiple gene modules that express together in rare cells within otherwise homogeneous clonal populations.
□ scNBMF: A fast and efficient count-based matrix factorization method for detecting cell types from single-cell RNAseq data
>>https://bmcsystbiol.biomedcentral.com/articles/10.1186/s12918-019-0699-6
scNBMF is a fast and efficient count-based matrix factorization method that utilizes the negative binomial distribution to account for the over-dispersion problem of the count nature of scRNAseq data, single-cell Negative Binomial-based Matrix Factorization.
With the stochastic optimization method Adam implemented within TensorFlow framework, scNBMF is roughly 10 – 100 times faster than the existing count-based matrix factorization methods, such as pCMF and ZINB-WaVE.
The reason of choosing negative binomial model instead of zero-inflated negative binomial model is that not only the most scRNAseq data do not show much technical contribution to zero-inflation, but also can largely reduce the computation burden in estimating drop-out parameters.

□ Bazam: a rapid method for read extraction and realignment of high-throughput sequencing data
>> https://genomebiology.biomedcentral.com/articles/10.1186/s13059-019-1688-1
Using Bazam, a single-source alignment can be realigned using an unlimited number of parallel aligners, significantly accelerating the process when a computational cluster or cloud computing resource is available.
Bazam, an alternative to SamToFastq that optimizes memory use, while offering increased parallelism and other additional features. Bazam increases parallelism by splitting the output streams into multiple paths for separate realignment.
□ SICaRiO: Short Indel Call filteRing with bOosting
>> https://www.biorxiv.org/content/biorxiv/early/2019/04/07/601450.full.pdf
SICaRiO is a machine learning-based probabilistic filtering scheme to reliably identify false short indel calls. SICaRiO uses genomic features which can be computed from publicly available resources, hence, apply it on any indel callsets not having sequencing pipeline-specific information (e.g., read depth).

□ SmCCNet: Unsupervised discovery of phenotype specific multi-omics networks
>> https://academic.oup.com/bioinformatics/advance-article-abstract/doi/10.1093/bioinformatics/btz226/5430928
A sparse multiple canonical correlation network analysis (SmCCNet), for integrating multiple omics data types along with a quantitative phenotype of interest, and for constructing multi-omics networks that are specific to the phenotype.
□ TeraPCA: a fast and scalable software package to study genetic variation in tera-scale genotypes
>> https://academic.oup.com/bioinformatics/advance-article-abstract/doi/10.1093/bioinformatics/btz157/5430929
TeraPCA can be applied both in-core and out-of-core and is able to successfully operate even on commodity hardware with a system memory of just a few gigabytes. TeraPCA features no dependencies to external libraries and combines the robustness of subspace iteration with the power of randomization.
□ Scrublet: Computational Identification of Cell Doublets in Single-Cell Transcriptomic Data
>> https://www.cell.com/cell-systems/fulltext/S2405-4712(18)30474-5
Single-Cell Remover of Doublets (Scrublet), a framework for predicting the impact of multiplets in a given analysis and identifying problematic multiplets. Scrublet avoids the need for expert knowledge or cell clustering by simulating multiplets from the data and building a nearest neighbor classifier.

□ cisTopic: cis-regulatory topic modeling on single-cell ATAC-seq data
>> https://www.nature.com/articles/s41592-019-0367-1
cisTopic, a probabilistic framework used to simultaneously discover coaccessible enhancers and stable cell states from sparse single-cell epigenomics data.
Using a compendium of scATAC-seq data from differentiating hematopoietic cells, brain and transcription factor perturbations, this topic modeling can be exploited for robust identification of cell types, enhancers and relevant transcription factors.

□ Integration of a Computational Pipeline for Dynamic Inference of Gene Regulatory Networks in Single Cells
>> https://www.biorxiv.org/content/biorxiv/early/2019/04/18/612952.full.pdf
an integrated pipeline for inference of gene regulatory networks. The pipeline does not rely on prior knowledge, it improves inference accuracy by integrating signatures from different data dimensions and facilitates tracing variation of gene expression by visualizing gene-interacting patterns of co-expressed gene regulatory networks at distinct developmental stages.
□ GEOracle: Discovery of perturbation gene targets via free text metadata mining in Gene Expression Omnibus
>> https://www.sciencedirect.com/science/article/pii/S1476927119301963
GEOracle to discover conserved signalling pathway target genes and identify an organ specific gene regulatory network.
GEOracle is a R Shiny app that greatly speeds up the identification and processing of large numbers of perturbation microarray gene expression data sets from GEO. It uses text mining of the GEO metadata along with machine learning techniques to automatically.
□ Genomic Selection in Rubber Tree Breeding: A Comparison of Models and Methods for dealing with G x E
>> https://www.biorxiv.org/content/biorxiv/early/2019/04/09/603662.full.pdf
The objective of this paper was to evaluate the predictive capacity of GS implementation in rubber trees using linear and nonlinear kernel methods and the performance of such prediction when including GxE interactions in each of the four models.
The models included a single-environment, main genotypic effect model (SM), a multi-environment, main genotypic effect model, a multi-environment, single variance G×E deviation model and a multiple-environment, environment-specific variance G×E deviation model.

□ Recentrifuge: Robust comparative analysis and contamination removal for metagenomics
>> https://journals.plos.org/ploscompbiol/article?id=10.1371/journal.pcbi.1006967
Recentrifuge’s novel approach combines statistical, mathematical and computational methods to tackle those challenges with efficiency and robustness: it seamlessly removes diverse contamination, provides a confidence level for every result, and unveils the generalities and specificities in the metagenomic samples.

□ OscoNet: Inferring oscillatory gene networks
>> https://www.biorxiv.org/content/biorxiv/early/2019/04/09/600049.full.pdf
The pseudo-time estimation method is more accurate in recovering the true cell order for each gene cluster while-requiring substantially less computation time than the extended nearest insertion approach.
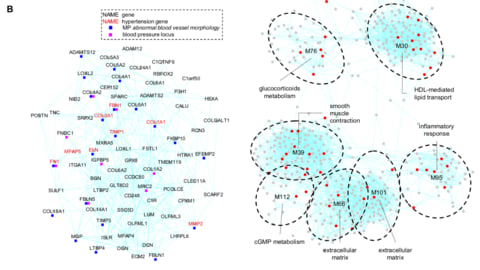
□ Gene modules associated with human diseases revealed by network analysis
>> https://www.biorxiv.org/content/biorxiv/early/2019/04/09/598151.full.pdf
A human gene co-expression network based on the graphical Gaussian model (GGM) was constructed using publicly available transcriptome data from the Genotype-Tissue Expression (GTEx) project. A graphical Gaussian model (GGM) network analysis identified unbiased data-driven gene modules with enriched functions in a variety of pathways and tissues.
□ Coupled MCMC in BEAST 2
>> https://www.biorxiv.org/content/biorxiv/early/2019/04/09/603514.full.pdf
an implementation of the coupled MCMC algorithm for the Bayesian phylogenetics platform BEAST 2. This implementation is able to exploit multiple-core CPUs while working with all models and packages in BEAST 2 that affect the likelihood or the priors and not directly the MCMC machinery.
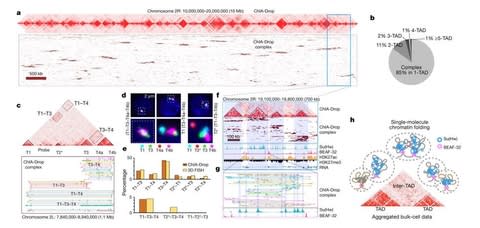
□ Multiplex chromatin interactions with single-molecule precision
>> https://www.nature.com/articles/s41586-019-0949-1
ChIA-Drop is a strategy for multiplex chromatin-interaction analysis via droplet-based and barcode-linked sequencing.
The chromatin topological structures predominantly consist of multiplex chromatin interactions with high heterogeneity; ChIA-Drop also reveals promoter-centred multivalent interactions, which provide topological insights into transcription.
□ RAINBOW: Haplotype-based genome wide association study using a novel SNP-set method
>> https://www.biorxiv.org/content/biorxiv/early/2019/04/18/612028.full.pdf
RAINBOW, is especially superior in controlling false positives, detecting causal variants, and detecting nearby causal variants with opposite effects.
By using the SNP-set approach as the proposed method, we expect that detecting not only rare variants but also genes with complex mechanisms, such as genes with multiple causal variants, can be realized.
□ High-Throughput Single-Molecule Analysis via Divisive Segmentation and Clustering
>> https://www.biorxiv.org/content/biorxiv/early/2019/04/09/603761.full.pdf
a new analysis platform (DISC) that uses divisive clustering to accelerate unsupervised analysis of single-molecule trajectories by up to three orders of magnitude with improved accuracy. Using DISC, reveal an inherent lack of cooperativity between cyclic nucleotide binding domains from HCN pacemaker ion channels embedded in nanophotonic zero-mode waveguides.
□ sbl: A Coordinate Descent Approach for Sparse Bayesian Learning in High Dimensional QTL Mapping and GWAS.
>> https://academic.oup.com/bioinformatics/advance-article-abstract/doi/10.1093/bioinformatics/btz244/5436130
The sparse Bayesian learning (SBL) method for quantitative trait locus (QTL) mapping and genome-wide association studies deals with a linear mixed model.

□ GRNTE: Gene regulatory networks on transfer entropy: a novel approach to reconstruct gene regulatory interactions
>> https://tbiomed.biomedcentral.com/articles/10.1186/s12976-019-0103-7
GRNTE uses transfer entropy to estimate an edge list based on expression values for different sets of genes that carry in time, and it corresponds to Granger causality for Gaussian variables in an autoregressive model.
This analytical perspective makes use of the dynamic nature of time series data as it relates to intrinsically dynamic processes such as transcription regulation, were multiple elements of the cell (e.g., transcription factors) act simultaneously and change over time.

□ HiCNN: A very deep convolutional neural network to better enhance the resolution of Hi-C data
>> https://academic.oup.com/bioinformatics/advance-article/doi/10.1093/bioinformatics/btz251/5436129
HiCNN is a computational method for resolution enhancement of Hi-C data. It uses a very deep convolutional neural network (54 layers) to learn the mapping between low-resolution and high-resolution Hi-C contact matrices.
□ TriNet: Multi-level Semantic Feature Augmentation for One-shot Learning
>> https://www.ncbi.nlm.nih.gov/pubmed/30969924/
In semantic space, searching for related concepts, which are then projected back into the image feature spaces by the decoder portion of the TriNet.
A concept space is a high-dimensional semantic space in which similar abstract concepts appear close and dissimilar ones far apart. The encoder part of the TriNet learns to map multi-layer visual features to a semantic vector.

□ Parsers, Data Structures and Algorithms for Macromolecular Analysis Toolkit (MAT): Design and Implementation
>> https://www.biorxiv.org/content/10.1101/605907v1
kD-Tree is a new approach of performance optimization by creating a few derived data structures. kD-Tree, Octree and graphs, for certain applications that need spatial coordinate calculations.
□ String Synchronizing Sets: Sublinear-Time BWT Construction and Optimal LCE Data Structure
>> https://arxiv.org/pdf/1904.04228.pdf
Given a binary string of length n occupying O(n/logn) machine words of space, the BWT construction algorithm due runs in O(n) time and O(n/logn) space. Recent advancements focus on removing the alphabet-size dependency in the time complexity, but they still require Ω(n) time.
the first algorithm that breaks the O(n)-time barrier for Burrows-Wheeler transform (BWT) construction. This algorithm is based on a novel concept of string synchronizing sets. a data structure of the optimal size O(n/logn) that answers longest common extension queries in O(1) time and, furthermore, can be deterministically constructed in the optimal O(n/logn) time.
□ DNBseqTM Rapid Whole Genome Sequencing
>> https://www.bgi.com/global/wp-content/uploads/sites/3/2019/04/DNBseq-Rapid-Whole-Genome-Sequencing-Service-Overview.pdf
DNBseqTM is an industry leading high-throughput sequencing technology, powered by combinatorial Probe-Anchor Synthesis (cPAS) and DNA Nanoball (DNB) technology. DNA Nanoball (DNBTM) technology concentrates more DNA copies in millions of nanospots in the flow cell, assuring high SNR imaging for accurate base calling.
□

□ Platanus-allee is a de novo haplotype assembler enabling a comprehensive access to divergent heterozygous regions
>> https://www.nature.com/articles/s41467-019-09575-2
The ultimate goal for diploid genome determination is to completely decode homologous chromosomes independently, and several phasing programs from consensus sequences have been developed. Platanus-allee (platanus2), initially constructs each haplotype sequence and then untangles the assembly graphs utilizing sequence links and synteny information. Platanus-allee with MP outperformed FALCON-Unzip and Supernova.

□ GOOGA: A platform to synthesize mapping experiments and identify genomic structural diversity
>> https://journals.plos.org/ploscompbiol/article?id=10.1371/journal.pcbi.1006949
Importantly for error-prone low-coverage genotyping, GOOGA propagates genotype uncertainty throughout the model, thus accommodating this source of uncertainty directly into the inference of structural variation.
Genome Order Optimization by Genetic Algorithm (GOOGA), couples a Hidden Markov Model with a Genetic Algorithm. The HMM yields the likelihood of a given ‘map’ (hereafter used to denote the ordering and orientation of scaffolds along a chromosome) conditional on the genotype data.
□ LCK metrics on complex spaces with quotient singularities:
>> https://arxiv.org/pdf/1904.07119v1.pdf
if a complex analytic space has only quotient singularities, then it admits a locally conformally Kaehler metric if and only if its universal cover admits a Kaehler metric such that the deck automorphisms act by homotheties of the Kaehler metric.
By using local Ka ̈hler potentials and compatibility conditions, the definition of locally conformally Ka ̈hler metrics can be extended to complex spaces.


□ HumanBase: data-driven predictions of gene expression, function, regulation, and interactions in human
>> https://hb.flatironinstitute.org
HumanBase applies machine learning algorithms to learn biological associations from massive genomic data collections. These integrative analyses reach beyond existing "biological knowledge" represented in the literature to identify novel, data-driven associations.
With NetWAS (Network-guided GWAS Analysis), HumanBase can aide researchers in identifying additional disease-associated genes.

□ deSALT: fast and accurate long transcriptomic read alignment with de Bruijn graph-based index
>> https://www.biorxiv.org/content/biorxiv/early/2019/04/17/612176.full.pdf
deSALT has the ability to well-handle the complicated gene structures as well as serious sequencing errors, to produce more sensitive, accurate and consensus alignments.
de Bruijn graph-based Spliced Aligner for Long Transcriptome read (deSALT) is a tailored 2-pass long read alignment approach constructs graph-based alignment skeletons to sensitively infer exons, and use them to generate spliced reference sequence to produce refined alignments.
□ Sparse Project VCF: efficient encoding of population genotype matrices:
>> https://www.biorxiv.org/content/biorxiv/early/2019/04/17/611954.full.pdf
This "Project VCF" (pVCF) form is a 2-D matrix with loci down the rows and participants across the columns, filled in with each called genotype and associated quality-control (QC) measures.
Sparse Project VCF (spVCF), an evolution of VCF with judicious entropy reduction and run-length encoding, delivering ∼10X size reduction for modern studies with practically minimal information loss.
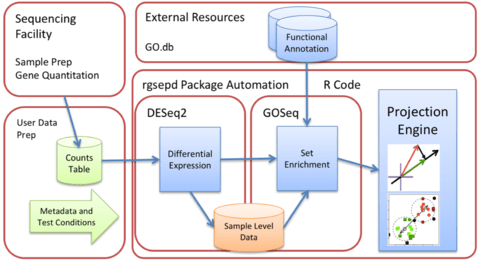
□ GSEPD: a Bioconductor package for RNA- seq gene set enrichment and projection display
>> https://bmcbioinformatics.biomedcentral.com/track/pdf/10.1186/s12859-019-2697-5
GSEPD, a Bioconductor package rgsepd that streamlines RNA-seq data analysis by wrapping commonly used tools DESeq2 and GOSeq in a user-friendly interface and performs a gene-subset linear projection to cluster heterogeneous samples by Gene Ontology (GO) terms.
Rgsepd computes significantly enriched GO terms for each experimental condition and generates multidimensional projection plots highlighting how each predefined gene set’s multidimensional expression may delineate samples.