
(Symbiotic R Aquarii: @hubble_space by Judy Schmidt)
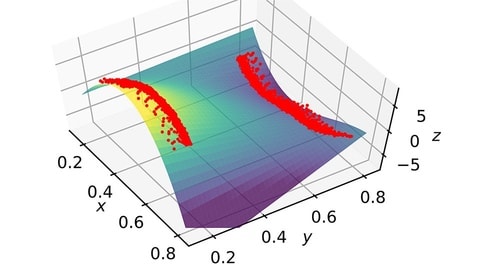
□ Scalar variable method draws analogies between some systems dealing with space, time and memory:
>> https://aip.scitation.org/doi/full/10.1063/1.5046671
State space reconstruction of spatially extended systems and of time delayed systems from the time series of a scalar variable. a bistable scalar system with delayed feedback, and a system composed by two lasers with delayed mutual cross coupling. Their dynamics can be reconstructed in a three-dimensional pseudo phase space, where the evolution is governed by the same polynomial potential.
□ Singular Value Decomposition of Operators on Reproducing Kernel Hilbert Spaces:
>> https://arxiv.org/pdf/1807.09331.pdf
Applications range from solving systems of linear equations and optimization problems to signal processing and to a variety of other methods in statistics and machine learning such as PCA, canonical correlation analysis, latent semantic analysis, and the hidden Markov models. Although the matrix SVD can be extended in a natural way to compact operators on Hilbert spaces, this infinite-dimensional generalization is not as multifaceted as the finite-dimensional case in terms of numerical applications. This is mainly due to the complicated numerical representation of infinite-dimensional operators and the resulting problems concerning the computation of their SVD.

□ Machine Learning for Integrating Data in Biology and Medicine: Principles, Practice, and Opportunities:
>> https://arxiv.org/pdf/1807.00123.pdf
The Bernoulli vectorization binarizes input data into discrete “on” or “off” categories for each region, based on whether or not the signal in that region exceeds a significance threshold based on a Poisson background distribution. IDEAS, finally, iteratively segments the genome for multiple input cell types at once, and classifies similar regions from across cell types using an infinite-state hidden Markov model.
□ D-SPACE: Deep Semantic Protein Representation for Annotation, Discovery, and Engineering:
>> https://www.biorxiv.org/content/biorxiv/early/2018/07/10/365965.full.pdf
D-SPACE encodes proteins in high-dimensional representations (embeddings), allowing the accurate assignment of over 180,000 labels for 13 distinct tasks. D-SPACE model is based on a deep convolutional neural network architecture with more than 100 million trainable parameters. Part of this model is a convergent affine ‘embedding’ layer consisting of 256 floating-point values, from which all classification outputs are derived. As an additional output, D-SPACE model includes an autoencoder to compress the 256-dimension protein embedding to a non-linear three-dimensional representation.
□ Elysium: RNA-seq Alignment in the Cloud:
>> https://www.biorxiv.org/content/biorxiv/early/2018/08/02/382937.full.pdf
Elysium has native programmatic access through the API to its functionality and alternative GUI. The uniform processing can place the newly processed data in context of more than 250,000 previously published RNA-seq data-sets currently available at the ARCHS4 resource.

□ scPred: Single cell prediction using singular value decomposition and machine learning classification:
>> https://www.biorxiv.org/content/biorxiv/early/2018/07/15/369538.full.pdf
scPred, a new generalizable method (scPred) for prediction of cell type(s), using a combination of unbiased feature selection from a reduced-dimension space, and and a support vector machine model. the advantage of scPred is that by reducing the dimensions of the gene expression matrix via singular value decomposition we also decrease the number of features to be fit, reducing both the computational requirements for prediction and the prediction model parameter space.
□ ExPecto: Deep learning based ab initio prediction of variant effects from DNA sequences:
>> https://www.nature.com/articles/s41588-018-0160-6
By exploiting the scalability of ExPecto, they characterized the regulatory mutation space for human RNA polymerase II–transcribed genes by in silico saturation mutagenesis and profiled > 140 million promoter-proximal mutations.
the chromatin predictions were computed from DeepSEA "Beluga" per 200bp bin, and 200 bins centered at TSS (40kb region) were used as input to predict expression effects. To reduce the dimensionality for ExPecto model training, the predicted chromatin spatial patterns were summarized to spatial features by 10 exponential basis functions. The summarized spatial features and gene expression levels were used to train regularized linear models for the final step of the prediction. The representative TSSes are selected based on FANTOM CAGE data.

□ Self-assembling Manifolds in Single-cell RNA Sequencing Data:
>> https://www.biorxiv.org/content/biorxiv/early/2018/07/07/364166.full.pdf
The Fano factor compares genes based on their variances relative to their average level of expression, which mitigates the inherent differences between gene expression distributions. Computing the Fano factors based on the kNN-averaged expressions links gene dispersion to the cellular topological structure. To directly visualize the corresponding kNN matrix, they used the Fruchterman-Reingold force-directed layout algorithm and drawing tools implemented by the Python package graph-tool.

□ pymfinder: a tool for the motif analysis of binary and quantitative complex networks:
>> https://www.biorxiv.org/content/biorxiv/early/2018/07/07/364703.full.pdf
the observed motif distribution is generally significantly different from the random expectation, showing either over- or under-representation relative to the results of the null model used here. This is evidence of a non-random organization of ecological communities, which speaks to the eco-evolutionary mechanisms shaping the ways in which different species interact with each other.

□ Multi-scale Deep Tensor Factorization Learns a Latent Representation of the Human Epigenome
>> https://www.biorxiv.org/content/biorxiv/early/2018/07/08/364976.full.pdf
a deep tensor factorization model, called Avocado, that outperforms both prior approaches in terms of the mean-squared error on a pre-defined 1% of the human genome. Avocado learns a latent representation of the genome that can be used to predict aspects of chromatin architecture, gene expression, promoter-enhancer interactions, and replication timing more accurately than similar predictions made from real or imputed data.
□ MLCSB: Machine Learning in Computational and Systems Biology (ISMB 2018)
>> https://www.iscb.org/cms_addon/conferences/ismb2018/mlcsb.php
#ISMB18
□ Stochastic Variational Inference of Mixture Models in Phylogenetics:
>> https://www.biorxiv.org/content/biorxiv/early/2018/07/08/358747.full.pdf
By proposing a new random variable Vk which is the unit length of the kth stick, the stick-breaking representation allows the construction of an infinite mixture structure. The allocations z = (zi) are drawn i.i.d from a multinomial of the infinite vector of mixing proportions, namely, φ = (φk ) , k ∈ [1, ..., ∞].

□ CNNC: Convolutional Neural Networks for Co-Expression Analysis:
>> https://www.biorxiv.org/content/biorxiv/early/2018/07/08/365007.full.pdf
Unlike most prior methods, CNNC is supervised which allows the CNN to zoom in on subtle differences between positive and negative pairs. CNNC provides a supervised way (tailored to the condition / question of interest) to perform co-expression analysis. To reduce overfitting CNNC determines specific thresholds based on the training for calling a pair correlated or anti-correlated or for inferring causality.
□ DoubletDecon: Cell-State Aware Removal of Single-Cell RNA-Seq Doublets:
>> https://www.biorxiv.org/content/biorxiv/early/2018/07/08/364810.full.pdf
DoubletDecon is able to account for cell-cycle effects, and is compatible with diverse species and unsupervised population detection algorithms (e.g., ICGS, Seurat).
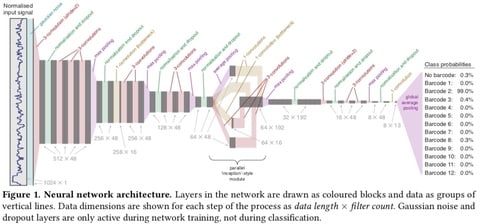
□ Deepbinner: Demultiplexing barcoded Oxford Nanopore reads with deep convolutional neural networks:
>> https://www.biorxiv.org/content/biorxiv/early/2018/07/10/366526.full.pdf
The 'signal-space' approach allows for greater accuracy than existing 'base-space' tools (Albacore and Porechop) in which signals have first been converted to DNA base calls, itself a complex problem that can introduce noise into the barcode sequence. Deepbinner had the lowest rate of unclassified reads (5.2%) and the highest demultiplexing precision (98.4% of classified reads were correctly assigned). It can be used alone (to maximise the number of classified reads) or in conjunction with Albacore (to maximise precision and minimise false positive classifications).

□ From squiggle to basepair: computational approaches for improving nanopore sequencing read accuracy:
>> https://genomebiology.biomedcentral.com/articles/10.1186/s13059-018-1462-9
The generation of multiple alignments of nanopore reads and the extraction of consensus sequences has the potential to eliminate all random errors, leaving only systematic errors that are introduced during sequencing or base calling.
□ UMAP Uniform Manifold Approximation and Projection for Dimension Reduction | SciPy 2018 |
UMAP is very efficient at embedding large high dimensional datasets. for a problem such as the 784-dimensional MNIST digits dataset with 70000 data samples, UMAP can complete the embedding in around 2.5 minutes.
the normalized Laplacian of the fuzzy graph representation of the i/p data is a discrete approximation of the Laplace-Betrami operator of the manifold, it can provide a suitable initialization for stochastic gradient descent by using the eigenvectors of the normalized Laplacian.
□ Carnelian: alignment-free functional binning and abundance estimation of metagenomic reads:
>> https://www.biorxiv.org/content/biorxiv/early/2018/07/23/375121.full.pdf
Carnelian (which uses Opal-Gallager hashes) trains on functionally annotated protein sequences by generating fixed-length fragments and their low-density spaced k-mer representations which are used as features by a set of one-against-all online classifiers. The learned model is then used to bin input amino acid sequences into appropriate functional bins. Abundance estimates are constructed from effective fragment counts in functional bins, and downstream differential abundance analysis is performed to find dysregulated ECs & pathways.

□ CellFishing.jl: an ultrafast and scalable cell search method for single-cell RNA-sequencing:
>> https://www.biorxiv.org/content/biorxiv/early/2018/07/25/374462.full.pdf
CellFishing.jl, a new method for searching atlas-scale data sets for similar cells with high accuracy and throughput. CellFishing.jl is scalable to more than one million cells, and the throughput of the search is approximately 1,350 cells per second (i.e., 0.74 ms per cell). a subspace with high variance is calculated by applying the SVD to the reference data matrix. Since the number of cells may be extremely large and singular vectors corresponding to small singular values are irrelevant, CellFishing.jl uses a randomized SVD algorithm that approximately computes singular vectors corresponding to the top D singular values.
□ M3C: A Monte Carlo reference-based consensus clustering algorithm:
>> https://www.biorxiv.org/content/biorxiv/early/2018/07/25/377002.full.pdf
In parallel, they developed clusterlab, a flexible Gaussian cluster simulator to test class discovery tools. Clusterlab can simulate high dimensional Gaussian clusters with precise control over spacing, variance, and size. M3C is also capable of dealing with complex structures using self-tuning spectral clustering, and can quantify structural relationships between consensus clusters using hierarchical clustering and SigClust.

□ polyRAD: Genotype calling with uncertainty from sequencing data in polyploids and diploids:
>> https://www.biorxiv.org/content/biorxiv/early/2018/07/30/380899.full.pdf
polyRAD can export genotypes as continuous numeric variables reflecting the probabilities of all possible allele copy numbers. This includes genotypes with zero reads, where the priors themselves are used for imputation. Genotype probabilities are estimated by polyRAD under a Bayesian framework, where priors are based on mapping population design, Hardy-Weinberg equilibrium, or population structure, with or without linkage disequilibrium.
□ Continuous State HMMs for Modeling Time Series Single Cell RNA-Seq Data:
>> https://www.biorxiv.org/content/biorxiv/early/2018/07/30/380568.full.pdf
they define the CSHMM model and provide efficient learning and inference algorithms which allow the method to determine both the structure of the branching process and the assignment of cells to these branches. Analyzing two developmental single cell datasets that the CSHMM method accurately infers the branching topology and that it is able to correctly and continuously assign cells to paths, in both cases improving upon prior methods proposed for this task.

□ Hofstadter’s butterfly and Langlands duality:
>> https://aip.scitation.org/doi/full/10.1063/1.4998635
a perspective on its mathematical structure of the corresponding tight-binding Hamiltonian from a viewpoint of the Langlands duality, a mathematical conjecture relevant to a wide range of the modern mathematics incl. number theory, solvable systems, representations, and geometry. Hofstadter’s fractal is deeply related with the Langlands duality of the quantum group. the existence of the corresponding elliptic curve expression interpreted from the tight-binging Hamiltonian implies a more fascinating connection with the Langlands program & quantum geometry.
□ A Robust Method to Estimate the Largest Lyapunov Exponent of Noisy Signals: A Revision to the Rosenstein’s Algorithm
>> https://www.biorxiv.org/content/biorxiv/early/2018/07/31/381111.full.pdf
This new method takes the advantage of choosing multiple neighboring points (rather than only one point as in the Rosenstein’s original method) at each step of computing divergence. Notwithstanding the relatively limited sample, the proposed method could be used to calculate LyE more reliably in experimental time series acquired from biological systems where noise is omnipresent.
□ All-optical machine learning using diffractive deep neural networks
>> http://science.sciencemag.org/content/early/2018/07/25/science.aat8084
3D-printed representations of neural networks you can run inference on by shining light through... literal *light speed* inference times.
□ Parameter tuning is a key part of dimensionality reduction via deep variational autoencoders for single cell RNA transcriptomics:
>> https://www.biorxiv.org/content/biorxiv/early/2018/08/05/385534.full.pdf
The k-means clustering approach evaluates the extent to which a hypersphere in the latent space is capable of capturing cell types accurately. with hyperparameter tuning, the performance of the Tybalt model, which was not optimized for scRNA-seq data, outperforms other popular dimension reduction approaches – PCA, ZIFA, UMAP and t-SNE.

□ DeepSignal: detecting DNA methylation state from Nanopore sequencing reads using deep-learning:
>> https://www.biorxiv.org/content/biorxiv/early/2018/08/06/385849.full.pdf
DeepSignal achieve similar performance on different methylation bases and different methylation motifs, while other methods, like signalAlign, has higher performance on 5mC methylation site than on 6mA methylation site. DeepSignal can detect 5mC and 6mA methylation site at genome level with above 90% accuracy under 5X coverage using controlled methylation data.
□ Featherweight long read alignment using partitioned reference indexes:
>> https://www.biorxiv.org/content/early/2018/08/07/386847
extend the Minimap2 aligner and demonstrate that long read alignment to the human genome can be performed on a system with 2GB RAM with negligible impact on accuracy.