
□ OregaIchiban:
人の無意識は根元でつながり一つになっているという。そこに行く方法のひとつとして夢の境界を越える、というものがある。境界線を越えた時、もし景色が色を失くしたり赤くなった場合は気をつけたほうがいい。夢見るものは人や生者だけとは限らないからだ
■ 無意識の定義を二分法的に考えると、我々が作用する可算領域と非可算領域の縁が、まるで黄昏時の稜線のごとく茫漠と横たわる様が垣間見える。夢を見るのに眠りにつく必要はない。過去は否応もなく夢と1つになる。
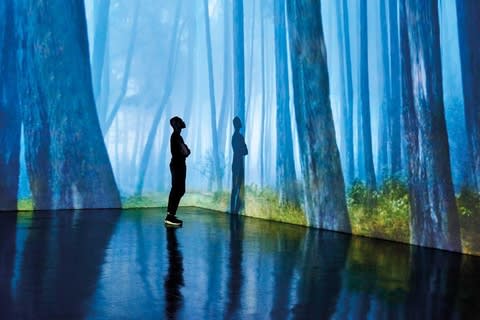
□ The Strange Order of Things: How we feel our way to being human
>> http://bit.ly/2FsulVu

□ _subodhpatil:
It's 3AM @CERN, and the dance of creation and annihilation continues above, and under ground...

□ From Tarski to Gödel. Or, how to derive the Second Incompleteness Theorem from the Undefinability of Truth without Self-reference:
>> https://arxiv.org/pdf/1803.03937v1.pdf
this could help us find a solution of Jan Krajicek problem to proof of the non-interpretability of the extension PC(A) of a consistent finitely axiomatized sequential theory A with predicative comprehension in A itself that does not run via the Second Incompleteness Theorem. A closely related question is whether our argument can be made constructive. This seems, at first sight, rather hopeless because of the radically non-constructive character of the Henkin construction. However, one can reduce the question the Second Incompleteness Theorem for constructive theories to the Second Incompleteness Theorem for classical theories. Now if we can make the argument completely theory-internal we would be there.

□ Asymptotic localization in the Bose-Hubbard model:
>> https://aip.scitation.org/doi/full/10.1063/1.5022757
For an equilibrating system, they would then expect that at some point in time the bound is surpassed because there should be a persistent energy current until the equilibrium energy content is reached, the theorem shows that these persistent currents are so small that the bound is not passed at times that are polynomially long in μ^−1. A fortiori, this shows that the timespan τeq needed for the system to reach equilibrium goes to infinity faster than any power of μ^−1.

□ Moonlight: a tool for biological interpretation and driver genes discovery:
>> https://www.biorxiv.org/content/biorxiv/early/2018/02/14/265322.full.pdf
A process is increased (decreased) if the associated functional enrichment analysis (FEA) yields positive (negative) Z-score values, i.e. high correlation (high anti-correlation) between the gene expression pattern and the literature-curated information. Then they determine whether a gene is increasing (decreasing) the biological process using an inferred gene regulatory network and subsequent Upstream Regulator Analysis (URA).

□ Quantifying configuration-sampling error in Langevin simulations of complex molecular systems:
>> https://www.biorxiv.org/content/biorxiv/early/2018/02/16/266619.full.pdf
a variant of the near-equilibrium estimator capable of measuring the error in the configuration-space marginal density, validating it against a complex but exact nested Monte Carlo estimator to show that it reproduces the KL divergence with high fidelity. a large collection of K = 1000 equilibrium samples using Extra-Chance Hamiltonian Monte Carlo (XC-HMC) to construct a large cache of independent equilibrium samples, amortizing the cost of equilibrium sampling across the many integrator variants.
□ PhysiBoSS: a multi-scale agent based modelling framework integrating physical dimension and cell signalling:
>> https://www.biorxiv.org/content/biorxiv/early/2018/02/16/267070.full.pdf
The multi-scale feature of PhysiBoSS - its agent-based structure and the possibility to integrate any Boolean network to it - provide a flexible and computationally efficient framework to study heterogeneous cell population growth in diverse experimental set-ups.

□ Nebula Genomics: Blockchain-enabled genomic data sharing and analysis platform:
>> https://www.nebulagenomics.io
>> https://www.nebulagenomics.io/assets/documents/NEBULA_whitepaper_v4.52.pdf
Data owners will privately store their genomic data and control access to it. Shared data will be protected through zero-trust, encryption-based secure computing. Data owners will remain anonymous, while data buyers will be required to be fully transparent about their identity. The Nebula blockchain will immutably store all data transaction records. Addressing data privacy concerns will likewise accelerate growth of genomic data.


Furthermore, a distributed secure computing platform based on SGX is currently being developed by Enigma (http://enigma.co). Nebula Genomics has established a partnership with Enigma. Enigma has a decentralized off- chain distributed hash-table (or DHT) that is accessible through the blockchain, which stores references to the data but not the data themselves.
□ OMEGA: a cross-platform data management, analysis, and dissemination of intracellular trafficking data that incorporates motion type classification and quality control:
>> https://www.biorxiv.org/content/biorxiv/early/2018/02/23/251850.full.pdf
OMEGA is based on the phase space of SMSS vs. ODC, which allows to quantify both the “speed” and the “freedom” of a group of moving objects independently. Global motion analysis reduces whole trajectories to a series of individual measurements or features. The combination of two or more of such features enables the representation of individual trajectories as points in n-dimensional phase space. OMEGA implements a single method to classify the dynamic behavior of individual particles regardless of their motion characteristics and employs the same method for particles whose dynamic behavior changes during the course of motion, as is commonly observed in living systems.
□ Cryptocurrency Will Boost Genome Sequencing
>> http://www.frontlinegenomics.com/news/19260/george-church-cryptocurrency-blockchain/

□ Characterization and visualization of RNA secondary structure Boltzmann ensemble via information theory:
>> https://bmcbioinformatics.biomedcentral.com/articles/10.1186/s12859-018-2078-5
Information entropy has been used to measure the complexity of the Boltzmann ensemble, and the mutual information between aligned sequences has been used to construct a consensus sequence. Using the nearest neighbor model (excluding pseudoknots), as implemented in the RNAstructure package, this algorithm finds the basepairs that provide the most information about other basepairs: the most informative base pairs (MIBPs).
□ DensityPath: a level-set algorithm to visualize and reconstruct cell developmental trajectories for large-scale single-cell RNAseq data:
>> https://www.biorxiv.org/content/biorxiv/early/2018/03/05/276311.full.pdf
by adopting the nonlinear dimension reduction algorithm elastic embedding, DensityPath reveals the intrinsic structures of the data. DensityPath extracts the separate high density clusters of representative cell states (RCSs) from the single cell multimodal density landscape of gene expression space, enabling it to handle the heterogeneous scRNAseq data elegantly and accurately. DensityPath constructs cell state-transition path by finding the geodesic minimum spanning tree of the RCSs on the surface of the density landscape, making it more computationally efficient and accurate for large-scale dataset. The cell state-transition path constructed by DensityPath has the physical interpretation as the minimum-transition-energy path.

□ Network-based Machine Learning and Graph Theory Algorithms. Excellent explanation of the graph Laplacian regularization in different learning frameworks with mathematical formulations in ST2
>> https://www.nature.com/articles/s41698-017-0029-7
In the hypergraph formulation introduced in the papers, the gene expression data are represented as weighted hyperedges on the patient nodes, and a graph Laplacian on the hypergraph can be introduced for semi-supervised learning on the patient samples.

□ Dynverse: A comparison of single-cell trajectory inference methods: towards more accurate and robust tools:
>> https://www.biorxiv.org/content/biorxiv/early/2018/03/05/276907.full.pdf
As there can be an overrepresentation of datasets of a certain trajectory type, first an arithmetic mean is calculated per trajectory type, followed by an overall arithmetic mean across all trajectory types, thus obtaining a ranking of the methods. To further limit the search space, they made sure the degree distributions between the two networks were similar, before assessing whether the two networks were isomorphic using the BLISS algorithm.
□ ExTraMapper: Exon- and Transcript-level mappings for orthologous gene pairs:
>> https://www.biorxiv.org/content/biorxiv/early/2018/03/06/277723.full.pdf
Their motivation behind using a greedy approach instead of a sequence alignment-like dynamic programming approach is that we want to favor exact or near exact mappings of exons over multiple mappings of lesser quality. ExTraMapper will have a great impact for translational sciences as it provides a dictionary for translating transcript-level information about gene expression and gene regulation from one organism to another.
□ NanoMod: a computational tool to detect DNA modifications using Nanopore long-read sequencing data:
>> https://www.biorxiv.org/content/biorxiv/early/2018/03/05/277178.full.pdf
Kolmogorov-Smirnov test is one of the most useful nonparametric test methods to quantify the distance between empirical distribution functions of two groups of samples. In NanoMod, Kolmogorov-Smirnov test is used for this purpose, since their purpose is to detect de novo modifications and since the actual distribution of signal intensity is not known a priori.

□ A Deep Predictive Coding Network for Learning Latent Representations:
>> https://www.biorxiv.org/content/biorxiv/early/2018/03/07/278218.full.pdf
a systematic approach for training deep neural networks using predictive coding in a biologically plausible manner. an inherent property of error-backpropagation is to systematically propagate information through the network in the forward direction and during learning, propagate the error gradients in the backward direction.
□ Shared contextual knowledge strengthens inter-subject synchrony and pattern similarity in the semantic network:
>> https://www.biorxiv.org/content/biorxiv/early/2018/03/07/276683.full.pdf

□ Hierarchical incompleteness results for arithmetically definable fragments of arithmetic:
>> https://arxiv.org/pdf/1803.01762v1.pdf
proving hierarchical versions of Mostowski’s theorem on independent formulae, Kripke’s theorem on flexible formulae, and a number of further generalisations thereof. As a corollary, we obtain the expected result that the formula expressing “T is Σn-ill” is a canonical example of a Σn+1 formula that is Πn+1-conservative over T. The properties of Σn-soundness and Σn+1-definability seem to go hand in hand since Σn-soundness of T implies consistency of T + ThΣn+1 (N).
□ Rapid calculation of maximum particle lifetime for diffusion in complex geometries:
>> https://aip.scitation.org/doi/full/10.1063/1.5019180
D∇^2Mk(x) = −kMk−1(x), x∈Ω,
For an arbitrary geometry, Eq. (2) can be solved numerically for Mk(x). To do this we use a finite volume method to discretize the governing equations over an unstructured triangular meshing of Ω. The finite volume method is implemented using a vertex centered strategy with nodes located at the vertices in the mesh and control volumes constructed around each node by connecting the centroid of each triangular element to the midpoint of its edges. Linear finite element shape functions are used to approximate gradients in each element. Assembling the finite volume equations yields a linear system, AMk=bk.
□ Differential Expression Analysis of Dynamical Sequencing Count Data with a Gamma Markov Chain:
>> https://arxiv.org/pdf/1803.02527.pdf
GMNB explicitly models the potential sequencing depth heterogeneity so that no heuristic preprocessing step is required. the gamma Markov negative binomial (GMNB) model that integrates a gamma Markov chain into a negative binomial distribution model, allowing flexible temporal variation in NGS count data. This allows GMNB to offer consistent performance over different generative models and makes it be robust for studies with different numbers of replicates by borrowing the statistical strength across both genes and samples.
□ Fast Parallel Algorithm for Large Fractal Kinetic Models with Diffusion:
>> https://www.biorxiv.org/content/biorxiv/early/2018/03/08/275248.full.pdf
the large-scale fractal kinetic models and the naive algorithm to a canonical substrate-enzyme model with explicit phase-separation in the product, and achieved a speed-up of up to 8 times over previous results with reasonably tight bounds on the accuracy of the simulation. Even a single diffusion error could catastrophically alter the dynamics of the simulation. their scheme, therefore, has to be completely devoid of diffusion errors. To generalize the naive algorithm to finite-cell multi-threaded simulations, they introduce the concept of covers. The cover containing one random sequence of cells from the lattice of length L x L would be equivalent to one Monte-Carlo Step of the naive algorithm. Thus, the naive algorithm can be thought of as such truly random cover at each MCS simulating the cells by its single sequence.
□ Optimizing Disease Surveillance by Reporting on the Blockchain:
>> https://www.biorxiv.org/content/biorxiv/early/2018/03/09/278473.full.pdf
Public Health agencies could fund the development of analytical models, directly through smart-contracts which would control the validation of the results releasing payments as the research project achieves pre-determined milestones, which can be validated automatically. the solution assuming a ledger with a Directed Acyclical Graph (DAG) topology, the system can be deployed on a classical linear blockchain, such as Ethereum.

□ 4Cin: A computational pipeline for 3D genome modeling and virtual Hi-C analyses from 4C data:
>> http://journals.plos.org/ploscompbiol/article?id=10.1371/journal.pcbi.1006030
4C-seq (Circular Chromosome Conformation Capture) is able to identify all the interactions of a given region of interest, usually termed ‘viewpoint’. With just ~1 million reads, 4C-seq can generate detailed high-resolution interaction profiles for a single locus. 5C (Chromosome Conformation Capture Carbon Copy) and Capture Hi-C, bridge somehow the gap between Hi-C and 4C-seq, being able to identify the large scale 3D chromatin organization of a given locus together with a high resolution contact map.
□ BART: a transcription factor prediction tool with query gene sets or epigenomic profiles:
>> https://www.biorxiv.org/content/biorxiv/early/2018/03/12/280982.full.pdf
Even though they have included as many ChIP-seq datasets as possible and will continue to update the compendium as more data become available, there are still many factors that do not have publicly available ChIP-seq data in any cellular system. After all, due to the incomplete coverage of cell and tissue types from public chromatin accessibility profiling and ChIP-seq data, the ability of BART in identifying transcription factors binding at specific cis-regulatory regions in an uncharacterized cell system is limited.
□ tri_iro:
無限時間チューリング機械の計算能力はかなり正確に分かっていて、Turing機械の停止問題,停止問題を神託に用いた機械の停止問題、これを神託に用いた機械の停止問題 etc. の計算順序数回の累積くらいなら無限時間チューリング機械では簡単に計算できて、超算術的階層くらいは軽く支配できるのですが
それだけでは留まらず、超算術的階層(Turing機械の停止問題の超限回の相対化の累積)を飛び越えるハイパージャンプという作用素があるんですけど、無限時間チューリング機械はハイパージャンプ作用素の超限回繰り返しも計算できます
ハイパーハイパージャンプは、ハイパージャンプの超限回の累積を越える計算不可能性を生み出す作用素で、集合論的には、ゲーデルの構成可能宇宙の次の再帰的到達不可能順序数ランクに飛ぶくらいですね。