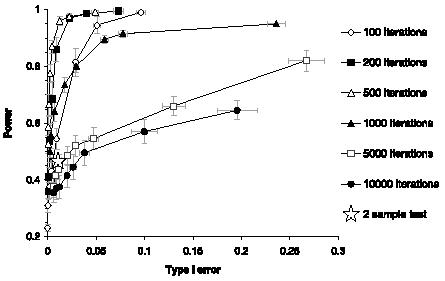
A.Chilingaryan, N.Gevorgyan, A.Vardanyan, D.Jones and A.Szabo
Multivariate approach for selecting sets of differentially expressed genes
Mathematical Biosciences Volume 176, Issue 1, March 2002, Pages 59-69
[PDF][Web Site]
・遺伝子抽出のための多変量解析的方法(multi-start random search method with early stopping (MRSES))の提案。マハラノビス距離を指標にして遺伝子を抽出する。
・データ
1.人工データ
2.Two colon cancer cell lines (HT29, HCT116)
・問題点「Currently used approaches ignore the multidimensional structure of the data. However it is well known that correlation among covariates can enhance the ability to detect less pronounced differences.」
・方法「We use the Mahalanobis distance between vectors of gene expressions as a criterion for simultaneously comparing a set of genes and develop an algorithm for maximizing it.」
・「It is well known that genes do not work independently; activation of one gene usually triggers changes in the expression level of other genes, that is genes are involved in so-called pathways.」
・特徴「In this paper we develop an approach that uses both the mean expression levels and the covariance structure of the data.」
・問題点「First, the number of possible gene combinations is enormously large; therefore it is impossible to compare all gene subsets and find the optimal one. On the other hand, if a global optimum could be found, it would be overly training sample specific, because of the phenomenon of overfitting.」
・問題点「Unfortunately, the problem of developing a simulation model for microarray data has been largely ignored, we are aware of only one attempt [19] in which a highly specific parametric model assuming independent genes is used.」
Multivariate approach for selecting sets of differentially expressed genes
Mathematical Biosciences Volume 176, Issue 1, March 2002, Pages 59-69
[PDF][Web Site]
・遺伝子抽出のための多変量解析的方法(multi-start random search method with early stopping (MRSES))の提案。マハラノビス距離を指標にして遺伝子を抽出する。
・データ
1.人工データ
2.Two colon cancer cell lines (HT29, HCT116)
・問題点「Currently used approaches ignore the multidimensional structure of the data. However it is well known that correlation among covariates can enhance the ability to detect less pronounced differences.」
・方法「We use the Mahalanobis distance between vectors of gene expressions as a criterion for simultaneously comparing a set of genes and develop an algorithm for maximizing it.」
・「It is well known that genes do not work independently; activation of one gene usually triggers changes in the expression level of other genes, that is genes are involved in so-called pathways.」
・特徴「In this paper we develop an approach that uses both the mean expression levels and the covariance structure of the data.」
・問題点「First, the number of possible gene combinations is enormously large; therefore it is impossible to compare all gene subsets and find the optimal one. On the other hand, if a global optimum could be found, it would be overly training sample specific, because of the phenomenon of overfitting.」
・問題点「Unfortunately, the problem of developing a simulation model for microarray data has been largely ignored, we are aware of only one attempt [19] in which a highly specific parametric model assuming independent genes is used.」
「マハラノビス距離」
検索結果→またもやぴかりん語(笑)!!!!!
ラーメンとお天気の話はどこにも…うぅぅぅ…。。。
夕飯はインスタントラーメンにします。
なんでAとかBとか、せめてわかりやすいアルファベットを使わないのでしょうか。
ただ、ベクトルはさすがに覚えていて安心しましたです、ハイ。
・さんぱち 100m
・山岡家 300m
・時計台ラーメン 800m
三つのラーメン屋さんがあったとして、今晩どこに行こうか? と考えたときに、一番近い「さんぱち」に行くかというと、そうとは限りませんよね。店までの距離(長さ、メートル)だけでなく、ラーメンの味、値段、その日の気分、天気などなどを全て考え合わせた結果で、どの店に行くかが決まると思います。
このように従来の単純な距離を、いろんな要素も考慮に入るように拡張して、それまでうまく測れなかったことも測れるように、マハラノビスさんが考案した便利な距離が『マハラノビス距離』、という感じかなぁ。大雑把に言うと。
《参考》
フリー百科事典『ウィキペディア(Wikipedia)』 マハラノビス距離
http://ja.wikipedia.org/wiki/%E3%83%9E%E3%83%8F%E3%83%A9%E3%83%8E%E3%83%93%E3%82%B9%E8%B7%9D%E9%9B%A2
ポイントは変数の数(いろんな要素)にあるのではなく、各変数の平均と分散を考慮に入れた、"偏り" または "重みづけ" に意義があるようです。
そろそろボロが出そうなのでこの辺で止めておこう。。。