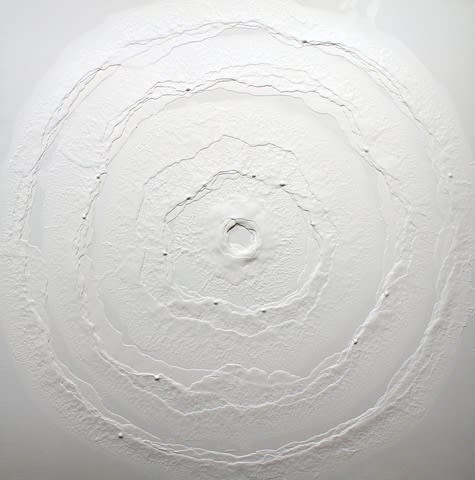
□ 夜は箱庭だった。一片の煌めきも抱けないはずの、その辺縁へと推しやる力を留めている。そこでは最初に価値が消え、次いで意味が失せていく。見えるもの、見たものを留めてはおけない。それらの影を己に見せているもの、そのスクリーンの向こう側を垣間見たいのだ。
□ 発話は記号の実効であり、発話が為されたと同時に、その反定立を意味論的に指向する。発話者の存在自体が、対話の瑕疵を予め規定しているのだ。然し我々そのものが遍在する記号でありながら、失うものだけに拘泥しようとする。事象や経験それ自体より、『解読』がエントロピーを基底状態へ導くからだ。
□ Arne Nordheim & Biosphere - Katedra Botaniki
ノルウェーの前衛音楽家アルネ・ヌールハイムは、いち早くから音像空間が時系列横断的な結晶であることを露呈させていた。その死後も進化を続ける音楽。
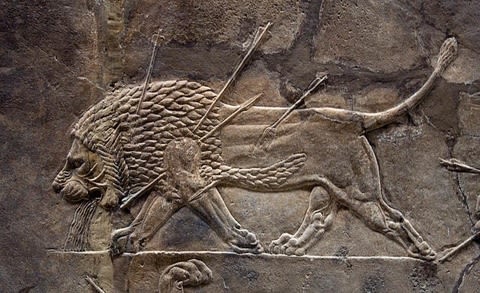
□ Ancient DNA reveals the lion’s past and (perhaps) future
>> http://blogs.biomedcentral.com/bmcseriesblog/2014/04/08/ancient-dna-reveals-the-lions-past-and-perhaps-future/
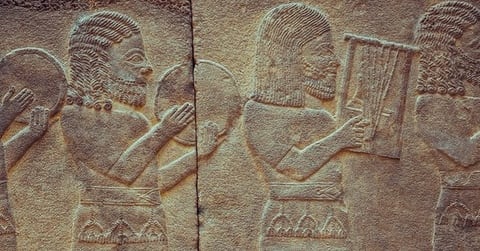
□ Music and human evolution
>> https://blog.oup.com/2017/07/music-human-evolution/

□ Mathematical mystery of ancient Babylonian clay tablet solved
>> https://eurekalert.org/e/7w48
□ GHOST: Recovering Historical Signal from Heterotachously-evolved Sequence Alignments:
>> http://www.biorxiv.org/content/biorxiv/early/2017/08/10/174789.full.pdf
the General Heterogeneous evolution On a Single Topology (GHOST) model for ML inference combines features of both MSR and MBL models. GHOST is free of the artificial constraints common in other models, included for computational expedience rather than biological relevance.
□ Orion: Detecting regions of the human non-coding genome that are intolerant to variation using population genetics:
>> http://journals.plos.org/plosone/article?id=10.1371/journal.pone.0181604
assessing the Orion scores by evaluating how they behave in in comparison with a number of genomic features, including protein coding exons, ultra-conserved non-coding elements (UCNEs), and DNase Hypersensitive sites (DHSs).
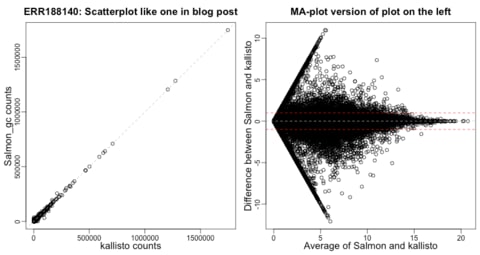
□ Salmon and kallisto
>> https://github.com/salmonteam/SalmonBlogResponse/blob/master/SalmonBlogResponse.md
>> https://liorpachter.wordpress.com/2017/08/02/how-not-to-perform-a-differential-expression-analysis-or-science/
Salmon (Patro et al., 2017) and kallisto (Bray et al., 2016) are clearly different algorithms. Salmon’s combination of a streaming phase with fragment-level modeling of each fragment-to-transcript mapping is what permits the support of fragment-level GC-content bias correction.
Eigen::VectorXd updateEffectiveLengths(ReadExperiment& readExp,
Eigen::VectorXd& effLensIn,
AbundanceVecT& alphas,
std::vector<double>& transcriptKmerDist)
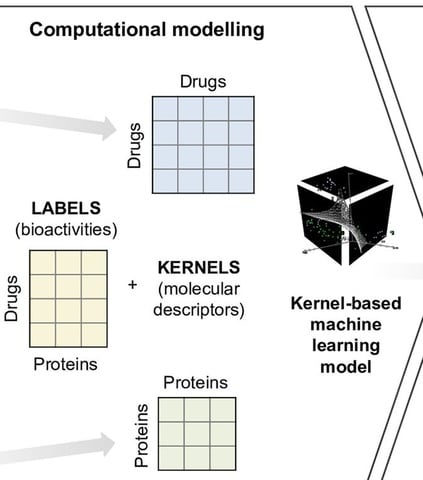
□ Computational-experimental approach to drug-target interaction mapping: kinase inhibitors:
>> http://journals.plos.org/ploscompbiol/article?id=10.1371/journal.pcbi.1005678
Molecular descriptors of drug compounds and protein targets are encoded as kernels, and used for binding affinity prediction with a regularized least squares regression model KronRLS.

□ Data-driven Advice for Applying Machine Learning to Bioinformatics Problems:
>> https://arxiv.org/pdf/1708.05070.pdf
empirically quantify the effect of hyperparameter tuning for each ML algorithm, demonstrating marked improvements in the predictive accuracy of nearly all ML algorithms.
GradientBoostingClassifier:
loss=“deviance”
learning_late=0.1
n_estimators=500
max depth=3
max features=“log2”
Datasets Covered: 51
□ RNAscClust: clustering RNA sequences using structure conservation and graph based motifs
>> https://academic.oup.com/bioinformatics/article-lookup/doi/10.1093/bioinformatics/btx114
The graph kernel decomposes each structure into several substructures, and can be regarded as an extension of k-mer decompositions from sequences to graphs.
1: G=(V,E)=(V,∅)
2: Rfam‐cliques=∅
3: for (h,l)∈{(0.95,0.9),(0.9,0.8),…,(0.5,0.4)}
do
4: E=E∪Ehl
5:whileG has a maximal clique of size ≥5
that contains a human sequencedo:
6:C=argmax meanPSI(c)
c∈maximal‐cliques(G),
c has human sequence,‖c‖≥5
7: Rfam‐cliques=Rfam‐cliques∪C
8: V=V∖C
remove vertices in C from G
□ ExSIS: Extended Sure Independence Screening for Ultrahigh-dimensional Linear Models:
>> https://arxiv.org/pdf/1708.06077.pdf
The ExSIS of linear models involving sub-Gaussian design matrices mainly requires establishing the screening condition and characterization. it is possible to reduce the dimension of an ultrahigh-dimensional, arbitrary linear model to almost the sample size, even when the number of active variables scales almost linearly with the sample size. on ultrahigh-dimensional linear models, the number of variables can scale exponentially w/ the sample size: log p = O(nα) for α∈(0, 1).
□ chiron 0.1.2.3: a deep learning CNN+RNN+CTC structure to establish end-to-end basecalling for the nanopore sequencer
>> https://pypi.python.org/pypi/chiron/0.1.2.3
>> http://www.biorxiv.org/content/early/2017/08/23/179531
Chiron has a novel architecture which couples a convolutional neural network w/ an RNN and a Connectionist Temporal Classification decoder. This enables it to model the raw signal data directly, without use of an event segmentation step. Chiron achieves basecalling speeds of over 2000 bases per second using desktop computer graphics processing units, making it competitive with other deep-learning-based basecalling algorithms.
Adjust Chiron parameters: change the hyper parameters in the FLAGS class.
self.home_dir = "/home/haotianteng/UQ/deepBNS/"
http://self.data _dir = self.home_dir + 'data/Lambda_R9.4/raw/'
□ Assessing consistency of genome-wide enhancer maps: Significant differences in distribution, evolution and function
>> http://www.biorxiv.org/content/early/2017/08/15/176610
a database of enhancer annotations in common biological contexts, creDB, which is designed to integrate into bioinformatics workflows. Simply calling all of the regions identified by the diverse enhancers obscures functionally relevant complexity & creates false dichotomies.
□ Maximizing ecological and evolutionary insight in bisulfite sequencing data sets
>> http://go.nature.com/2vIwpPA
「バイサルファイト塩基配列解読データセットにおける生態学的および進化的な手掛かりの最大化」
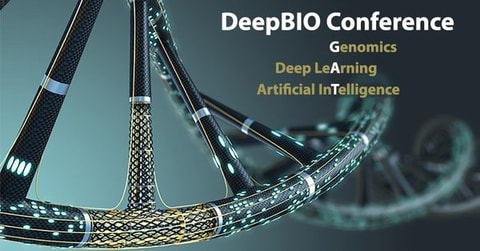
□ DeepBIO Conference: Deep Learning, Genomics, Bioinformatics and Machine Learning. (Dec 1st, 2017 in San Diego.)
>> https://www.mlsociety.com/events/deepbio-conference/
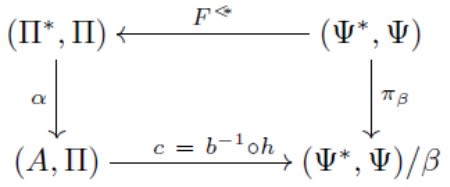
□ Towards a category theory approach to analogy: Analyzing re-representation and acquisition of numerical knowledge:
>> http://journals.plos.org/ploscompbiol/article?id=10.1371/journal.pcbi.1005683
a formal learning model of re-representation, language processing and analogy can explain the acquisition of knowledge of rational numbers. the symbolic model (Π*, Π) can “emulate” any semantic domain with form (A, Π) in a unique way through the mapping α.
□ Kafka Interfaces for Composable Streaming Genomics Pipelines:
>> http://www.biorxiv.org/content/biorxiv/early/2017/08/29/182030.full.pdf
Although scalability has been shown up to 12 nodes, there is circumstantial evidence that, in the chosen cluster setup and application, a single Kafka broker will be able to support scaling up to about one hundred nodes.
□ Streamformatics: Real-time nanopore species-typing viz. Runs local server to coord fastq extraction, alignment and species id
>> https://github.com/mbhall88/streamformatics
□ Game changer for laptop based analysis of nanopore data! Will incorporate into @zibraproject pipeline ASAP.
>> http://simpsonlab.github.io/2017/09/06/nanopolish-v0.8.0/
□ tmVar 2.0: Integrating genomic variant information from literature with dbSNP and ClinVar for precision medicine
>> https://academic.oup.com/bioinformatics/article/doi/10.1093/bioinformatics/btx541/4101939/tmVar-2-0-Integrating-genomic-variant-information
□ SANA: separating the search algorithm from the objective function in biological network alignment:
>> https://arxiv.org/pdf/1709.01464.pdf
an impartial comparison of modern network alignment objective functions using simulated annealing. SANA can perform over a million iterations per second on most machines, but without incremental evaluation it slows by orders of magnitude. L-GRAAL’s modified GDV similarity, normalized differently and using only graphlets with 4 or fewer nodes.
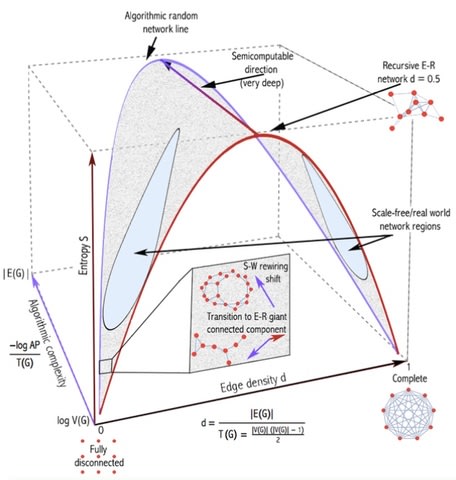
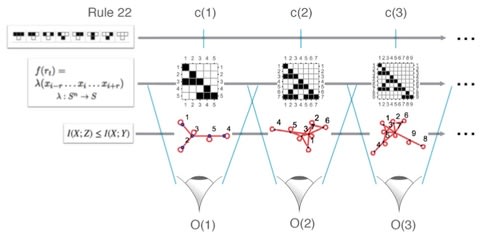
□ An Algorithmic Information Calculus for Causal Discovery and Reprogramming Systems:
>> http://www.biorxiv.org/content/biorxiv/early/2017/09/07/185637.full.pdf
Formally, |C(s)U1 - C(s)U2|

□ Complexity of evolutionary equilibria in static fitness landscapes:
>> http://www.biorxiv.org/content/biorxiv/early/2017/09/12/187682.full.pdf
hard landscapes allow adaptationist accounts for the absence of evolutionary equilibrium even in experimental models w/ static environments. We will have to switch to a language of “adapting” rather than “adapted”, reason from disequilibrium, and seek mechanisms by which the species selects which unbounded adaptive path to follow.
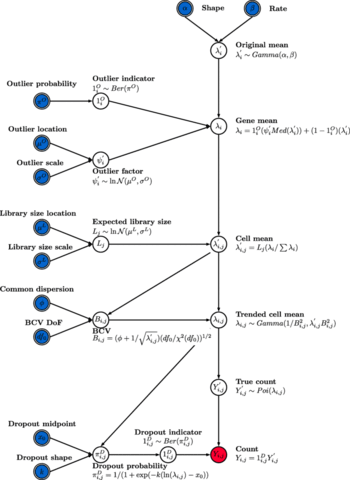
□ Splatter: simulation of single-cell RNA sequencing data based on a gamma-Poisson distribution:
>> https://genomebiology.biomedcentral.com/articles/10.1186/s13059-017-1305-0
the Splat simulation to capture many features observed in real scRNA-Seq data, including high expression outlier genes, differing sequencing depths between cells, trended gene-wise dispersion, and zero-inflation. dropout (in Splat) or zero-inflation often failed to improve the match to real datasets, suggesting that they are not truly zero-inflated.
□ clusterSeq: methods for identifying co-expression in high-throughput sequencing data:
>> http://www.biorxiv.org/content/biorxiv/early/2017/09/13/188581.full.pdf
These are both founded on the principle that two genes may be considered co-expressed if the observed values of the two events form identical equivalence clusters whose average behaviour is strictly monotonic b/n the 2 events.
□ Clive_G_Brown:
GPU accelerated "Guppy" base caller now on poretal - Developer only for now.
~800K bases per second / GPU
□ hackseq:
>> http://www.hackseq.com
Project 1: BioSyntax: Parsing biological file formats for humans with syntax highlighting 🖊️
□ DeepCode: deep learning of the AS (epi)genetic code reveals a novel linking histone modifications to fate decision
>> http://www.biorxiv.org/content/biorxiv/early/2017/09/15/189183.full.pdf
the multi-label architecture was introduced to decode the SPs represented as five-dimension binary vectors. validated the ability of DeepCode and DNN in predicting the inclusion level changes, which directly reflects the lineage-specificity.
□ conStruct: Inferring continuous and discrete population genetic structure across space:
>> http://www.biorxiv.org/content/biorxiv/early/2017/09/15/189688.full.pdf
a cross-validation analysis that uses Monte Carlo cross-validation to determine the statistical support for models with different numbers of layers or with and without a spatial component. but caution against overly strict interpretation of either.
the posterior probability
density of the parameters as:
P ( w,α,φ,η,γ | Ω ) ∝ P { Ω | Ω(w,α,φ,η,γ,D) } P(w)P(α)P(φ)P(η)P(γ),
calculate.layer.contribution <- function(conStruct.results,data.block,layer.order=NULL){
if(any(grepl("chain",names(conStruct.results))))
□ NanoLyse: using minimap2/mappy from @lh3lh3 to remove @nanopore sequencing reads mapping to the lambda phage genome.
>> https://github.com/wdecoster/nanolyse
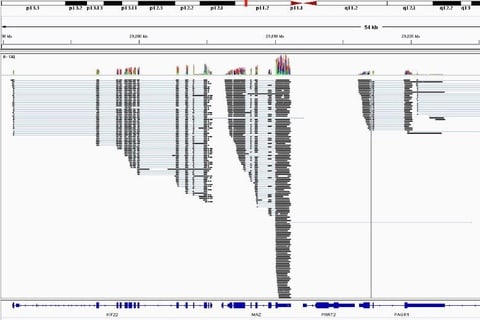
□ DrT1973:
Minimap2 splice, smashing it with @nanopore Direct RNA read mapping. 1.7M in 20mins, thx @lh3lh3 :0).
□ RAPID GWAS OF THOUSANDS OF PHENOTYPES FOR 337,000 SAMPLES IN THE UK BIOBANK
>> http://www.nealelab.is/blog/2017/7/19/rapid-gwas-of-thousands-of-phenotypes-for-337000-samples-in-the-uk-biobank

□ Integration of anatomy ontologies & Evo-Devo using structured Markov chains for modeling discrete phenotypic traits
>> https://www.biorxiv.org/content/early/2017/09/19/188672
the theoretical consideration for improving the modeling of discrete morphological characters in phylogenetic context. This approach substantially restricts the possibility for exploring hidden space of evolution.
□ LDJump: Estimating Variable Recombination Rates from Population Genetic Data:
>> https://www.biorxiv.org/content/biorxiv/early/2017/09/20/190876.full.pdf
apply a segmentation algorithm to estimate breakpoints in recombination rates subject to under type I error control against over-estimating the number of identified segments.

□ Distributed and dynamic intracellular organization of extracellular information:
>> https://www.biorxiv.org/content/biorxiv/early/2017/09/21/192039.full.pdf
multi-dimensional internal representations are widespread within cellular biology and their failures in encoding information, by causing dysfunctional decision-making, can instigate deleterious behaviors and disease.

□ A Dirichlet Mixture Model of Hawkes Processes for Event Sequence Clustering:
>> https://arxiv.org/pdf/1701.09177.pdf
Given N training sequences of C-dimensional Hawkes processes, each of which contains I events, when the parameters of each Hawkes process is sparse, its computational complexity will reduce to O(NI(IC +L(C +I))+C2). the learning algorithm discretizes each impact function into L points and estimates them via finite element analysis.
□ A Sequel to Sanger: Amplicon Sequencing That Scales. This platform allows massive amplicon characterization
>> http://bit.ly/2fdkYcP @PacBio
□ Goodarzi Uses Math, RNA Biology to Counter Metastasis:
>> https://nihrecord.nih.gov/newsletters/2017/09_22_2017/story4.htm
□ JuliaDiumenge:
Does the coding region of a gene contain regulatory information? Is it the same for all genes? @LucasBCarey CIO:
□ Promoter architecture determines co-translational regulation of mRNA
>> https://www.biorxiv.org/content/early/2017/09/22/192195
he ORF sequence is a major regulator of gene expression, and a non-linear interaction between promoters and ORFs determines mRNA levels.